Machine Learning Enabled High-Sigma Verification for Memory Design – Innovation to cure ‘Chip Memory Loss’
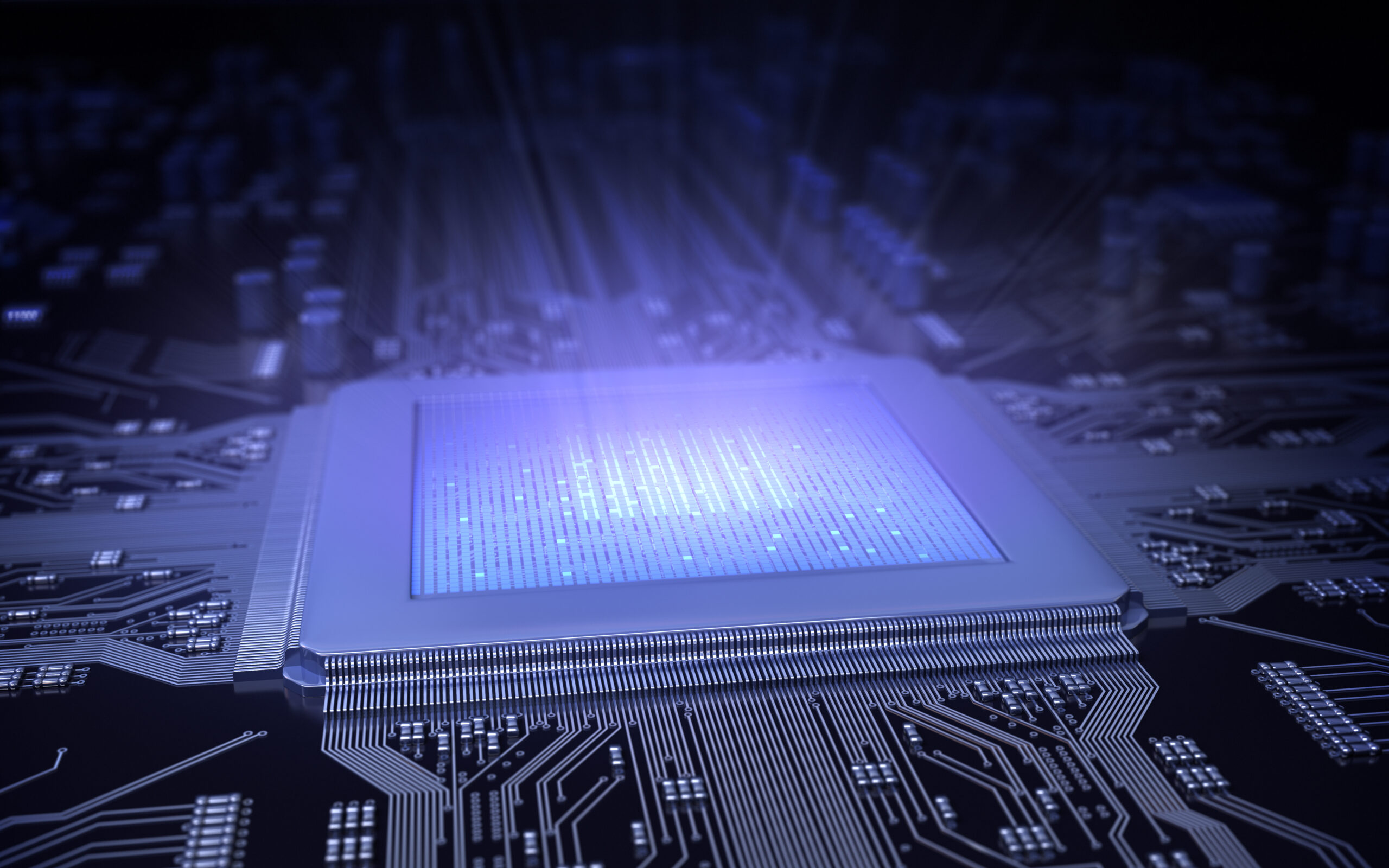
The Covid-19 pandemic has presented us with many challenges in this past year. Working from home has certainly changed my daily structure, and I would venture to say the same goes for most of my colleagues and customers. Our use of network and application tools, including daily video conference calls, and high volumes of data analyzation and content creation, has become a living constant. Then, there is the growing demand for in-home streaming entertainment content spurred by the many stay-at-home orders. Indeed, the growth of hyperscale data centers continues to be fueled by the connectivity boom and new 5G technologies, in turn driving chip demand.
Memory design accounts for a significant portion of these chips used in data center application, driven by the demand to store, transfer and access vast amounts of data. In addition to cost and scalability, energy efficiency has become an increasingly important factor for these hyperscale data centers, driving innovation for low-power consumption memory designs.
High-sigma verification is imperative to meet memory design specifications and requirements for low-power, low-die and high-performance. Requiring massive amounts of Monte Carlo Simulations – 10s of millions to billions – to verify using a traditional brute force approach.
I invite you to explore my recently published article ““Machine Learning Enabled High-Sigma Verification for Memory Designs” and learn how Siemens EDA’s Solido Variation Designer uses proprietary machine learning techniques, to deliver fast and accurate high-sigma verification for memory design.