Listen to the symphony of ‘Symphony Pro’ at DVCon 2023
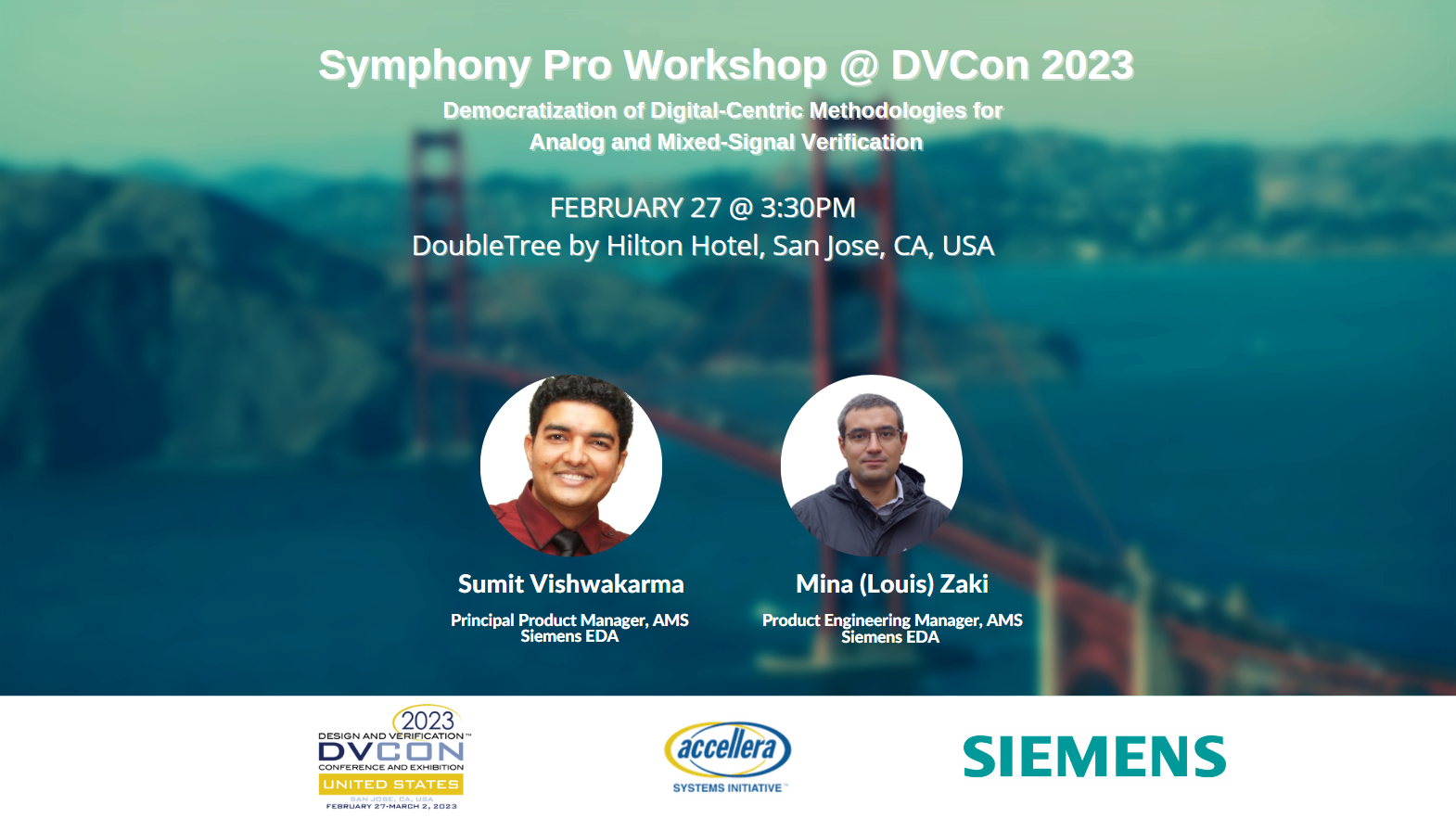
We are at a tipping point of AI-generated content, be it text, images, video, or music. Chat Generative Pre-trained Transformer a.k.a. ChatGPT is the most recent talk of the town. It is a conversational AI model developed by OpenAI and is trained on a massive dataset of text from the internet. Training large language models like GPT-3.5 requires a significant amount of computational power and resources. This is due to the size of these models, which can have billions of parameters, making them highly complex and demanding on hardware. To meet these demands, state-of-the-art semiconductor chips and specialized hardware are required. These chips, such as graphics processing units (GPUs) or tensor processing units (TPUs), are designed to handle the massive amount of mathematical computations necessary for training large language models. They are highly sophisticated, optimized for deep learning workloads, and provide the necessary parallel processing capabilities to process large amounts of data quickly. In addition to specialized hardware, the infrastructure supporting the training of these models must also be highly optimized, with fast data storage, high-bandwidth interconnects, and efficient power management. These factors combine to make the training of large language models a challenging and complex task that requires a deep understanding of both hardware and software systems.
For example, verifying high-bandwidth interconnect PHYs in AI hardware is a challenging task that requires advanced mixed-signal verification EDA tools. These interconnects play a crucial role in the overall performance of AI systems and their verification must be accurate and efficient. The high-speed and complex nature of these interconnects make them susceptible to various electrical and timing issues, making it difficult to verify their functionality using traditional verification methods. The verification process involves checking the integrity of connectivity between digital and analog blocks, which adds another layer of complexity to the verification process. To ensure that these interconnects meet the desired specifications and performance targets, high-class mixed-signal verification tools are required to perform comprehensive simulations and analysis. These tools must be capable of handling high-speed signals, accurate modeling of the interconnects, and be able to verify the complex interactions between the digital and analog signals.
The interaction between analog and digital signals in today’s System-on-a-Chip (SoC) has become increasingly complex making traditional divide-and-conquer analog verification methodologies impractical. Analog and mixed-signal designers are investing in methodologies used by the digital verification teams by modeling analog at a higher abstraction level. Digital verification methodologies, which are mature, structured, and automated, have become the industry standard for ensuring the quality and reliability of digital circuits. However, traditional analog verification methods, such as directed tests, sweeps, and Monte Carlo analysis, are insufficient for mixed-signal SoC designs. To address these challenges, analog verification teams must adopt digital verification techniques, such as automated stimulus generation, assertions, and coverage-driven verification, to ensure the functional correctness of their designs.
At DVCon 2023, we are hosting a workshop “Democratizing digital-centric mixed-signal verification methodologies” covering all the capabilities of our latest advanced mixed-signal verification solution Symphony Pro along with a live demo. Come join us at DVCon 2023 on Feb 27th at 3:30 PM and listen to the soothing symphony of Symphony Pro. (I can assure you it will be much more comforting than your AI-generated euphony 😊 )