A “Whirley Pop” for Library Characterization
In this new era of social distancing, my binge-watching has peaked. Well, what I do miss of course is the nice crisp buttery popcorn that I get at movie theaters. I do make popcorn at home in my microwave. However, it always resulted in several un-popped kernels that tend to ruin my popcorn enjoyment… So, lately, I have been experimenting with various ways to pop popcorn kernels and finally found a product – ‘Whirley Pop’ – that does exactly what I want – fully popped kernels which not only taste yummy but also cut down my popping time by half.
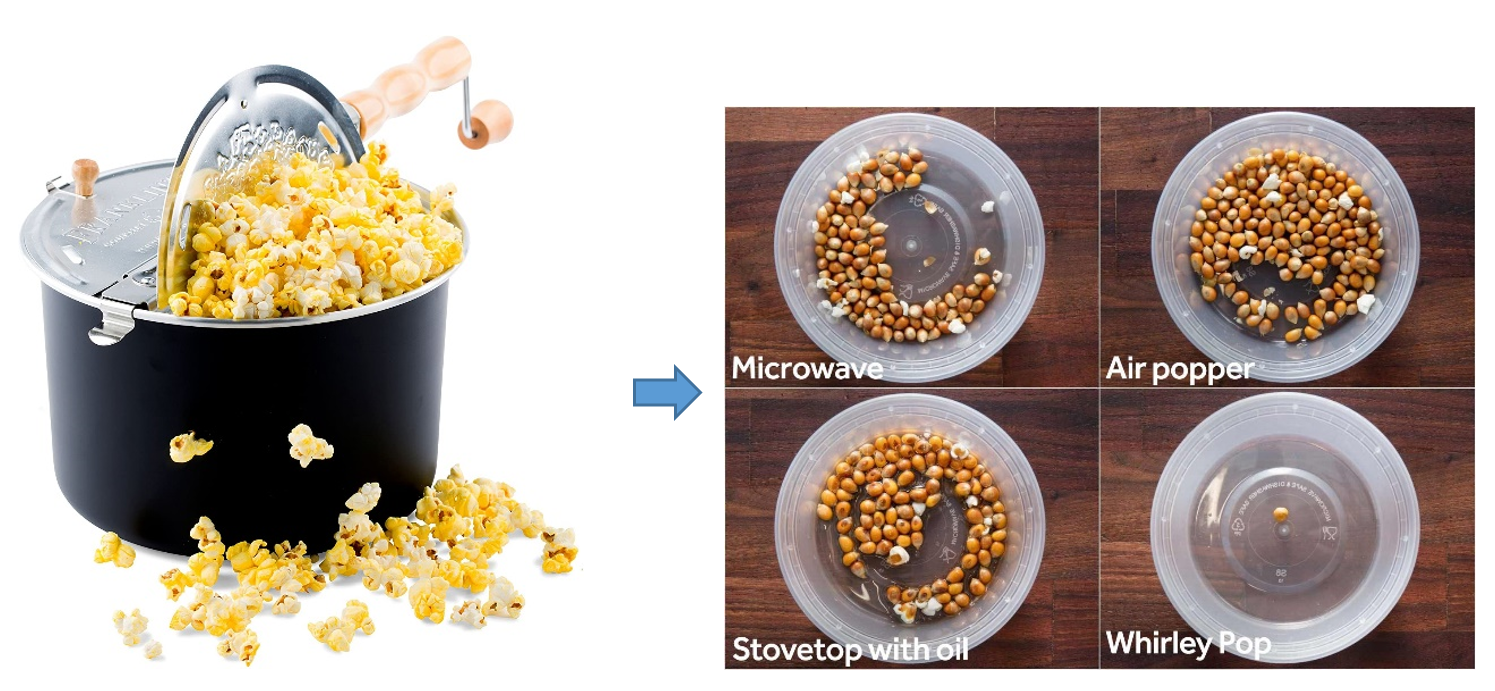
Enjoying my fully-popped popcorn had me thinking about library characterization: Although there’s an unmistakable difference in magnitude of severity, on a conceptual level, finding un-popped kernels in my bowl of popcorn is like finding issues in my timing .libs. I’m very unhappy when I find one, and it makes me wonder how many other defects are hidden in this batch of results.
Digital design and signoff flows are completely dependent on having correct and accurate .libs for all process, voltage, and temperature (PVT) corners within design specification. With more PVTs needed for each newer process node, the size of a full set of timing .libs has grown into gigabytes of data. If we look deeper into the library characterization process, we can find many reasons why characterized results could contain errors or inaccuracies, like having incorrect SPICE simulation settings, or characterization environment settings. In other words, there’s a really big bowl of popcorn, and without the right tools, we won’t know how many un-popped kernels are hidden in there, waiting to make our lives difficult.
In the case of library characterization, the consequences of incorrect or inaccurate data in .libs are quite serious – overdesign and tape-out delays are the less severe outcomes, while worse outcomes include non-functional silicon and re-spins.
This is where Solido Analytics comes in. Analytics is a timing .lib sign-off verification tool that uses machine learning to automatically identify all issues and outliers in the library, in addition to rule-based checks. This helps library teams ensure correctness and accuracy of their .libs, and helps digital teams save valuable engineering time on timing closure iterations.
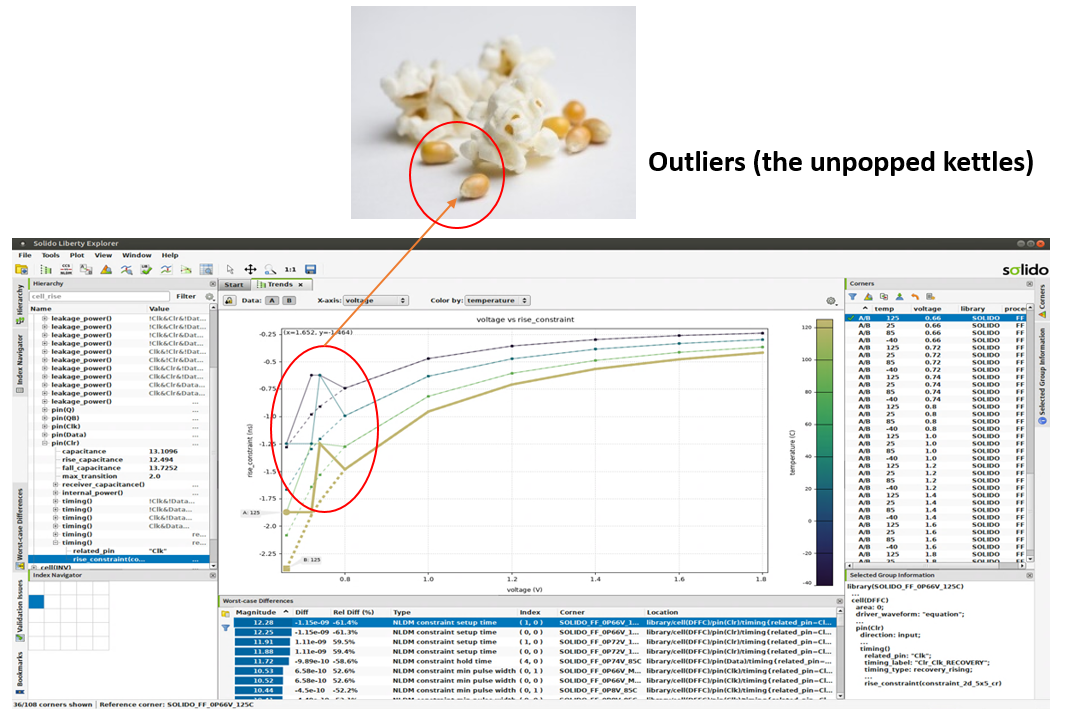
One of Solido Analytics advantages is the machine learning engine that enables it to automatically identify any outliers in .lib data. This allows the tool to generate its own set of “expected” results that can be compared against actual .lib data, and flag any discrepancies it finds, completely independent of static rules. The result: Analytics will reliably identify a whole new set of issues previously undetectable by traditional .lib checkers, and in less runtime than traditional .lib checking tools.
If you’re interested, you can learn more about this in our On-demand Webinar
BTW, you can watch the webinar with a bucket full of whirley-pop popped popcorns 🙂