Sky’s not the limit: AI and data management redefining space exploration
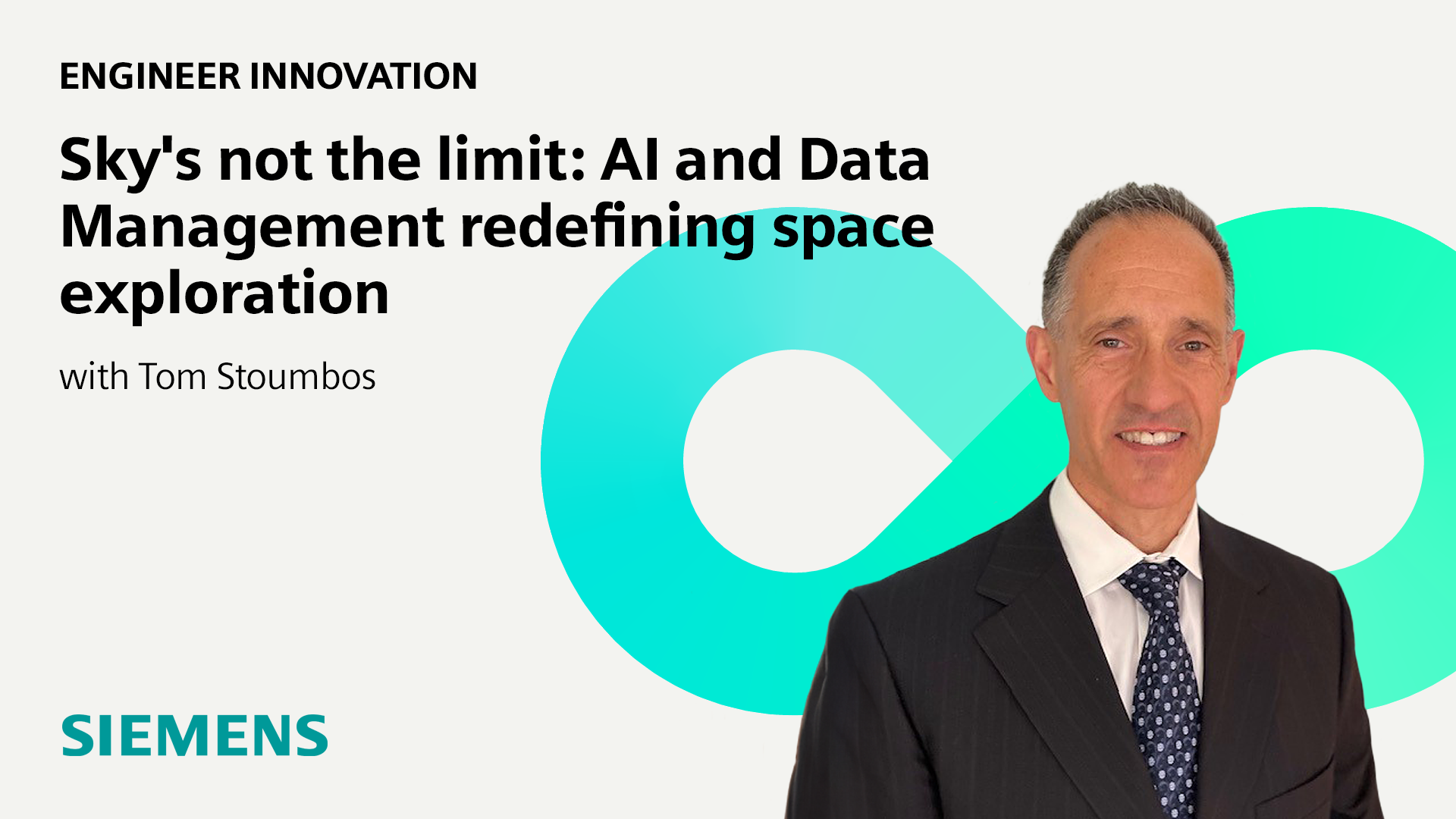
Guest Tom Stoumbos
Listen on Apple
Listen on Spotify
Show Notes
In this episode, recorded at Realize LIVE Americas 2024, we’re joined by Tom Stoumbos, Director of Engineering at Northrop Grumman. We delve into the fascinating world of space exploration and the intricate technologies behind space vehicles and satellite systems. Tom leads a team of simulation and test engineers and reveals the complexities of designing for the cosmos, from servicing commercial satellites to supporting ambitious lunar missions. Learn about the vital role of simulation in overcoming the challenges of deep space, the innovative refueling technologies in development, and the potential of AI and machine learning in pioneering the next era of space travel. Don’t miss the insights from a leading mind in aerospace engineering, where every mission pushes the boundaries of human achievement and ignites our imagination for the final frontier.
Key Takeaways:
- The role of simulation in the design and testing of space vehicles and satellite systems
- Establishing infrastructure on the moon is a critical steppingstone for deep space missions due to the reduced power required to launch from the moon compared to Earth
- The challenges associated with space exploration include the need for accurate simulations and the management of vast amounts of data. Machine learning and AI can help process and analyze this data more efficiently.
- The importance of collaboration between industry and academia
- Predicting the establishment of a lunar orbiting lab in the next few years and possible lunar infrastructure within the next 15 years.
This episode of the Engineer Innovation podcast is brought to you by Siemens Digital Industries Software — bringing electronics, engineering and manufacturing together to build a better digital future.
If you enjoyed this episode, please leave a 5-star review to help get the word out about the show and subscribe on Apple or Spotify so you never miss an episode.
Guest biographies and episode transcript
- The role of simulation in the design and testing of space vehicles and satellite systems
- Establishing infrastructure on the moon is a critical steppingstone for deep space missions due to the reduced power required to launch from the moon compared to Earth
- The challenges associated with space exploration include the need for accurate simulations and the management of vast amounts of data. Machine learning and AI can help process and analyze this data more efficiently.
- The importance of collaboration between industry and academia
- Predicting the establishment of a lunar orbiting lab in the next few years and possible lunar infrastructure within the next 15 years.
Chad Ghalamzan:
Hello and welcome to the Engineer Innovation podcast. I’m your host, Chad Ghalamzan. In today’s episode, we have Tom Stoumbos from Northrop Grumann, who will be sharing his expertise on space exploration and the exciting advancements in the field. As the leader of a team of talented engineers, Tom is responsible for designing and building the infrastructure, satellites, and space vehicles that will pave the way for humanity’s journey to the moon and beyond.
Joining us from Realize Live Americas 2024 in Las Vegas, Nevada, Tom brings a wealth of knowledge and experience in this fascinating field. So, get ready to dive into the world of space exploration with us as we discuss the realities and possibilities of venturing into the final frontier.
Hi, Tom. Thank you for joining us.
Tom Stoumbos:
Hi, Chad. Thank you for hosting.
Chad Ghalamzan:
My pleasure. Why don’t you introduce yourself and the work you do at Northrop Grumman?
Tom Stoumbos:
Absolutely. My name is Tom Stoumbos. I manage a team of simulation and test engineers that do all the design analysis and tests of our space vehicles and satellite systems from our tactical space systems.
Chad Ghalamzan:
So you work on several different space vehicles. Can you maybe elaborate on some of the different types of vehicles you’re looking at right now?
Tom Stoumbos:
Yeah, that is correct. We have a number of different satellite structures, satellite designs, as well as space vehicles for different space exploration projects. So, from the simple science satellites that last a couple of years, science missions to collect useful data for NASA and the other government entities, as well as commercial communication satellites that is your DishNet or your DirecTV that orbit Earth in a geostationary form and transmit high definition data as well as space vehicles associated with space station resupply missions, which is very critical.
We’ve been doing that for over a decade, as well as now through the Artemis program for the Space Station for the lunar exploration, lunar orbiting lab, and the extension of that that goes into lunar landing, lunar terrain vehicles, and of course, long-term lunar infrastructure that will take us to Mars. So, there’s a number of programs actively designing and building space hardware as well as developing the groundwork for these future space and deep space missions.
Chad Ghalamzan:
So we talked with several guests recently about space exploration and designing vehicles for space, and you’re probably one of the few guests we have that can answer this question with the right qualifications behind it to explain why is going to the moon so important for pushing our boundaries in terms of exploring the universe?
Tom Stoumbos:
Yeah, generally, there’s a lot of limitations from the fact that launching from Earth, it requires a lot of power for the launch vehicles and the launch vehicles are limited with respect to the mass they can launch or even the volume of the payloads they can launch. So, having the infrastructure in the moon as a stepping stone for deep space missions is critical, being able to develop the infrastructure to reduce the fuel and also manufacture.
Actually, the launch vehicles in the moon or deep space vehicles will make it so much easier to do that trip into deep space, especially as we’re looking at being able to have some human colonies beyond Earth and the Mars. Of course, that is a high probability for the future. So, having infrastructure in the moon allows us to make that launch into Mars or other potential planets possible.
Chad Ghalamzan:
Maybe you could just elaborate a little bit more because I was actually talking about this with a few people yesterday because you presented here at Realize LIVE and I was sharing some of the insights you gave in and even help for me to understand, the moon is not that far away from Earth relative to what we’re talking about for deep space exploration. So, how does that help become a stepping stone because the relative distance there…
Tom Stoumbos:
Because the gravity of Earth, of course, makes it very difficult for a launch to be able to carry heavy payloads and then through the lunar orbit around Earth and the dynamics of the planets, it’s easier with less fuel to launch a deep space mission, especially if it involves humans from the lunar surface than the Earth itself.
Chad Ghalamzan:
So we’re looking at drastically scaling up the number of missions that would potentially be going deeper into space and then of course adding human beyond.
Tom Stoumbos:
Absolutely, because there’s so much exploration you can do with the instruments, and then at some point, you would need the human factor to be part of it, especially if you’re planning to go beyond just bringing samples back. So, at some point, there is a need to have a human into the deep space. So, that makes it challenging. Again, with that said, it is not just launching a launch vehicle onto the moon and that having the launch vehicle take off from there, right? It’s difficult to establish a launch site here on Earth, furthermore in the moon that has no infrastructure. So, developing all that stuff may require some manufacturing and refueling depots in space in between the moon and Earth.
So, it’s an extremely challenging task and there’s a lot of steps that we take before we can get to that point. That is of course with the all adverse environmental conditions in the moon, having the ability to develop the infrastructure, manufacturing facilities and fuel production facilities and even a launch site in the moon that we can launch satellite to understand the lunar surface and how the vehicles will traverse the lunar surface. So, there’s a lot of human factors along with engineering challenges that the teams collectively with the different governments and the different governments, their work to make sure they can accomplish this task.
Chad Ghalamzan:
Even when it comes to the moon, I think it was only earlier this year, Japan was able to land a satellite on the far side of the moon. It was one of the first times we’ve ever been able to do that. So, even exploring the moon is still something we’re doing and it seems like a solved problem, right? Because people think back, “Well, the Apollo missions 50 years ago,” but space exploration is not a solved problem. It’s still one of the most difficult engineering problems that we’re trying to tackle.
Tom Stoumbos:
Absolutely. We’re talking about 50 years later we landed humans. Since then we haven’t done it. The last few attempts by India, Japan, that US private venture, we’re able to land a space vehicle on the moon, but it didn’t really go any for one trip. They couldn’t continue the mission. So, the first success criterion was to be able to land over one. So, 50 years later, talking about that as a successful attempt shows how difficult space.
Chad Ghalamzan:
Yeah, definitely challenging. So, you gave a presentation yesterday here at Realize LIVE and you had a quote on one of your slides. Simulation is critical to making mission operations and design decisions better. Can you elaborate on that? How come simulation and test is so critical to that for you at Northrop Grumman when you’re trying to tackle these problems?
Tom Stoumbos:
Yeah, historically, humankind trying to understand physics, combine it with math through developing prototypes. Developing prototypes is expensive and it’s limited and it takes a long time with the tools that we have at our disposal today and the tools that Siemens develops. In addition to that, the fact that Siemens works closely with the customers to develop tools that attend to the customer’s needs. Operating in the virtual world is much easier than the prototypes in the real world with all the constraints about volume, space, mass that that takes.
So, having the tools to develop very high fidelity models, models that are based on high order algorithms, it’s invaluable because it allows you to explore the space in areas that we don’t really understand very well in the virtual world and come close to that environment as opposed to try to make guesses through some limited prototyping that provide some information to start, but that’s where the simulation and the virtual world takes off.
So, being able to develop models, develop models quickly, develop models that are directly connected to the requirements and the concept of the operation is critical. Of course, once you develop the models, we have the computing power to be able to run thousands of simulations, generate enormous amount of results and of course enormous amount of data that we have to understand and post-process quickly.
Chad Ghalamzan:
So is it because there is that computing power now available, you feel that maybe the interest in doing these types of deeper space explorations and building a lunar economy, it is getting that scale to go with it? Is it because there’s now powerful tools that allow companies like Northrop Grumman to actually do these types of simulations virtually? There’s no prototyping in the sense of a vehicle. You can prototype it then go drive it, but space is space. Until you launch it and get it up there, there’s only so much testing you can do on earth. Is this the availability of tools that’s changing the game to some extent?
Tom Stoumbos:
We can develop virtual simulation models at scale, so we can do low-end cheaper prototypes that we can generate a lot of data to correlate our simulation models. It’s that after we have some confidence in those simulation models, then we can extrapolate and in the virtual world really explore that space environment that allow us to better understand how the actual hardware will behave the space. So, simulation gives us that ability to see beyond what the prototype does and everything that we cannot see through the limitations we have from just building and testing prototypes. In addition to that, we can explore the design space.
It allows us to reach optimized solution, then explore the feasibility of that optimized solution at the same time, engage manufacturing credibility, and see whether that is possible and then engage the launch vehicle infrastructure and see how that is applicable to really making that mission happen and then simulate the lunar surface and develop vehicles and infrastructure that can really work in the lunar surface and make the whole operation sustainable.
Chad Ghalamzan:
So you talked about several space vehicles or satellites that have been developed or being developed. One of them was a refueling technology that you have been working on. Maybe give us a sense with just that particular technology, that particular satellite refueler, how much data and time simulating something like that would be involved in a project like that? Because I’m assuming you’re not just doing a structural simulation and that’s it. You’re doing multiple types of simulation, looking at multiple physics and you’re doing this, like you said, with design exploration. What’s the scale here for just that one project?
Tom Stoumbos:
There are different levels of simulations that can take place, and incrementally, you add fidelity by going from your one-dimensional assumptions to the two dimensions, to the three dimensions, and to your fully executable six degree of freedom system. That also includes not only your linear assumptions, your high order algorithms to simulate everything that that space system will do. In the case of a refueling vehicle, it’s not only a vehicle that is just in orbit waiting for a client vehicle to dock and be able to refuel, but it’s simulating the refueling event itself, the refueling hardware. You need some active and passive systems that will allow the two vehicles to engage, will allow the fuel pump to engage and all this without any issues because any little incident in space is not recoverable.
So, by using a series of tools that allows us to do the linear stress analysis, identify the dynamics of the vehicle through normal modes to use the nonlinear simulations, go into the dynamics of how the vehicles interact with each other would allow us to develop a very high fidelity flexible model of the different subsystems that come together and then simulate also the operation of the systems coming together, performing the operation, and then everything else in between, because I would say we have an infinite number of configurations that those vehicles can be in while that operation is taking place, right? You’re designing these systems to be able to facilitate any client vehicle and any configuration.
For example, you can have the first engagement with all your deep fuel tanks full. Once you did refuel on a few vehicles, those tanks are not going to be full. So, you’re going to have a fuel mass slosh, which means the dynamics are going to change. Your mass and center of mass of the system and the subsystem is going to be changed. So, the dynamics are going to be changed. So, in order to be able to do that design exploration efficiently and accurately, you need to engage the virtual world of high fidelity digital trends, because as you understand, it is not feasible to do that with the actual hardware and prototype test.
So, the small scale prototype testing is beneficial to obtain some data and then I would say near infinite number of iterations and simulations that we need to run to have high confidence that both the hardware they’re going to build is going to behave and perform as design as well as all the systems that come together are going to engage the right way and we’re going to have successful mission is by default we need to gain the confidence. So, the data we’re going to produce with thousands of simulations. I’m pretty confident. So, that following the digital simulation, the virtual simulation route is much more efficient and faster, because with the prototyping, it takes a long time to develop a concept, to identify what is missing from the concept and go back and build the hardware.
In the virtual world, you can do design updates quickly and you don’t need to engage the engineers anymore. Once you have a baseline prototype in the virtual world, you can engage AI through custom algorithms that search for the optimum solutions based on the design constraints and the optimization algorithms that we have. We continue to train in order to be able to generate this optimum design and continue to develop that concept, but ultimately, we need to identify what is the confidence level that we need to have to be able to have a successful mission.
Chad Ghalamzan:
Right. I would assume that in some of your examination of this, you have to look at risk. You have to look at recovering from failures or what happens as well. It’s not just a question of determining what makes a success, but also understanding when failure occurs, how you recover from it, if you can recover from it or what to do in those instances.
Tom Stoumbos:
Absolutely, and then it’s up to the team to identify what is the risk level that makes this mission feasible. Beyond that, based on the simulation data and the information produced by the simulation data, we have high confidence first on the team and the algorithms to make sure the data gives us reasonable information. We make these decisions because you need to quantify the risk you’re taking and also understand what the mission constraints are. But beyond that, we try to also reduce and offset the risk by engaging with academia that are much more flexible. Because there’s a lot of applications in the everyday environment that are similar to the space operations. For example, the drones that we have or the various air vehicles, serial manipulators.
We are able with small scale problems like using drones, drones with robotic arms, drones with robotic arms and end effectors and tools to simulate in a relatively simple way the space operation and do both the testing, which is at a smaller scale, and the high fidelity executable digital twin of that application, like having a drone with a robotic arm and a tool. It’s similar to having a space vehicle with a robotic arm and a tool operate in space because I can have the drone in a small rule, collect all the data, instrument it very cheaply, and correlate that model. Then all these things we learned from that correlation, take it into a space vehicle, develop a high fidelity space vehicle, and have high confidence that the mission operations simulation is going to give me good results, that I can have a calculated risk assessment and a high probability of having a successful mission.
Chad Ghalamzan:
You’ve talked a lot about the challenges here. Are there other challenges in these complex structures for space that we haven’t had a chance to talk about yet?
Tom Stoumbos:
Well, the models are complex, which means-
Chad Ghalamzan:
By models, you mean the simulation model?
Tom Stoumbos:
Correct.
Chad Ghalamzan:
So there’s a lot of data.
Tom Stoumbos:
There’s a lot of fidelity. For example, a space vehicle, it comprises of a lot of subsystems. So, it’s just not the structure. We have motors, we have mechanisms, we have complex joints that we need to simulate or operate. We have software that controls robotic arms. So, we need to make sure we understand how these things are controlled from the control system of that space vehicle.
Chad Ghalamzan:
The right environment because presuming that the environment here plays a drastic role in the performance of all these things.
Tom Stoumbos:
Absolutely. That’s why we need to just not drive the operation with the as planned mission operation, but based on what the operation description is, then see how the hardware behaves and how close they come to that. For example, the robotic arm projector, right? It’s not going to be 100% what is designed on paper. So, we need to use multi-physics tools. We need to use the control algorithms used for those mechanisms, even the motor physical model into our simulation such that we understand also all the uncertainties that go into that operation, because every time you perform an operation, the same thing with every time you build something, it’s going to be a little bit different.
That’s why we have the tolerances. So, we need to understand with all the combination of the tolerances and all the combination of the control system behavior based on the hardware that is being used, what are all those simulations going to show us for a probability of success? So when I’m talking about high fidelity model, I’m talking about having the flexible properties of the model, having all the information on the tolerance. For example, every time we build a spring and we put it into a mechanism, it’s going to have a slight different stiffness. Does that stiffness compromise the functionality of that system and space? Any space vehicle has extreme temperature gradients across the system itself.
So, we need to make sure we understand once that vehicle is its space and it operates at different instances with different gradients, what is that system performance going to be and is it going to be within the envelope that’s going to lead us to an operational success? So with that said, this is hundreds and even thousands for a complex missions terabyte of data that we need to sort and it cannot be done by just an analyst or an engineer just sorting through the data. So, that brings us to the value of artificial intelligence and machine learning as it applies to simulation.
Chad Ghalamzan:
Yeah, you took the words right out of my mouth. I can imagine these are enablers for having that much data. We met a couple of years ago and we spoke, it was prior to the recent boom in AI that we’ve seen for the last couple of years mainly because of the LLM side, but still it’s coming up a lot at this conference. It’s coming a lot in all of our conversations. How is artificial intelligence and machine learning becoming a game changer for companies? So you’re leveraging this now to help process the data. Where does AI and machine learning fit into your strategy as we are talking about these space vehicles and your desire to launch them?
Tom Stoumbos:
Yeah, it’s the next step from our side on space exploration because the more data we have, the easier it is for us to assess the risk when we try and make a decision about space-
Chad Ghalamzan:
I guess there’s never too much data for that purpose, but there’s too much data for us to consume or for a human to consume.
Tom Stoumbos:
Correct. So, having a constant stream of data coming in that I see it as an opportunity to reduce risk and being able to process it quickly brings us to these algorithms that I think with time they’re going to continue to evolve and help us even more because they need to search through the data. They need to understand what the data represents and ultimately work with us to help us understand what the optimum solution is. But we need to understand as the algorithms are being developed, whether they do lead to the optimum solution.
So, we have to do the homework ourselves. We have to work with our tool provider, in this case Siemens. We have to work with academia that we engage a lot and understanding what these algorithms are, what is the optimum way to use them in order to be able to post-process the data. Because it’s going to take a long time and it’s prone to a human error if we’re relying on the analysts to try to sort through the data and understand what’s the right path.
Chad Ghalamzan:
I guess scale’s a big part of it too. I mean, I just came out of this morning’s keynote, I don’t know if you were in it, but the last presenter mentioned how digitalization can’t scale without AI to some effect. There’s truth in that, right, with all that data.
Tom Stoumbos:
With a computing power exponential increase, now the introduction of these high-end graphics cards that allow us to both visualize and also process data quickly, it doesn’t make sense now to engage more with AI and machine learning, but we do need to understand what the algorithm represent. There is a misconception that AI is the same across the different areas, whether it’s searching data on the World Wide Web or sorting through simulation data. So, we need to make sure the algorithms are properly conceived and they help us reach that optimum solution, but it’s definitely much faster.
I believe we can say 50% of the time to market time, assuming time to market is with the hardware being fully verified and validated and ready to launch by engaging as soon as we can with AI and ML, understanding the algorithms, push all the data through these data processing scripts, assess and validate and verify what the optimum solution show, and ultimately demonstrate that we have a high probability of being successful in that operation.
So, without a doubt, it’s a game changer. The sooner we engage with it, the better we’re off. That’s why as soon as we found out that Siemens is basically applying across all their tools a different form of AI, we’re engaging with Siemens. We have frequent meetings to understand and be next to Siemens as they develop these algorithms and in the process see how we can benefit.
Chad Ghalamzan:
Could you be a bit more specific about some of the AI tools that you are using right now and how they’re helping with your…
Tom Stoumbos:
We’re basing a lot of the work we do on HEEDS. We think it’s an invaluable tool to do that first step of tying all the solvers that we’re using together and allowing us to do these design of experiments or Monte Carlo simulations for our missions quickly and efficiently. There is an AI tool that has been added to HEEDS that will allow us the enormous amount of data, talking about thousand simulations per operation, running through HEEDS and organizing the data and post-processing it through the AI algorithm that the Siemens team has built and enhancing is invaluable. So, we’re working closely with the HEEDS team to take full advantage of the AI module.
The same thing with Teamcenter Simulation, because of the enormous amount of data that we’re using, it’s silly to think that we can continue to manually manage the data. So, we’re engaging on a pilot program with DCSIM to be able to get this data processing for our fully executable digital twin through our multibody dynamics team to be able to use all the tools and the data and connected the requirements and downstream with [inaudible 00:26:57] and manufacturing inputs to make sure that designs we come up with is really feasible.
So, we’re really counting on DCSIM to help us organize the data, so we reduce the probability of errors and also we’re able to connect all the requirements with all the design notes, reports, simulation, and test data that we produce from now on into the future, talking about these complex lunar exploration, deep space exploration missions. The sooner we engage with these tools, the easier it will be to be able to come up with the optimal solution and reduce the errors, reduce the time to be able to start building hardware, testing hardware.
So, we’re excited. In addition to that, because we’re engaging with academia, like I mentioned before, on some projects with ASU and Virginia Tech and building algorithms and doing some small-scale models to verify and validate our processes, in our space vehicles, they are also developing artificial intelligence and machine learning algorithms to post-process their data. We have created this team between Siemens, Northrop Grumman, and the universities we work with to make sure that we all take advantage of each other’s work where it has to do with these algorithms. So, ultimately, we have the same target to basically develop simulation tools that are accurate and give us the optimal solution.
So, we’re tackling it from both angles from the university experience with producing and post-processing enormous amount of data and with a Siemens experience working with a number of companies and helping them solve their challenges associated with simulation, digital transformation, and data processing with AI.
Chad Ghalamzan:
So you talk a lot about how maybe AI is helping you enhance the process of analyzing your simulation data, of going through all that information and making sense of it or exploring with HEEDS, but you talked about Teamcenter Simulation and I’m mindful that not everyone is familiar with what that is, and I believe you were an adopter of this tool of ours. We’re talking about AI now, but before even AI, you were using Teamcenter Simulation to help manage the data, and I think that was also something you’re a firm believer. Maybe you could just elaborate a little bit for our listeners, what is Teamcenter Simulation and how is that an enabler for you to do all this work?
Tom Stoumbos:
Oh, absolutely. We have been engaging with Teamcenter Simulation of Siemens for, I would say myself, almost 10 years now. Working with Rick Licursi and Raghav to understand how it can apply to our applications and of course working together through the years allows us to not only understand the tool changes and improvements, but also from Siemens side, understand what the customer needs is and adjust it accordingly.
Chad Ghalamzan:
But this tool allows you to manage your data, allows you to manage your workflows. How does the Teamcenter Simulation work for you exactly? It’s helping you manage all these different types of conditions. When we’re looking at a space vehicle, is it helping you to make sense of all the different failure tests you need to keep? Maybe you could just elaborate.
Tom Stoumbos:
Yeah. It allows the engineer to operate within the Teamcenter Simulation active workspace where you engage the simulation tools through the simulation, which allows you to track what tools you use, what requirements you tied those to, and all the data that has been generated, like I mentioned, the thousands of simulations we’re under. It’s all labeled, it’s all structured, and it all resides within that network. So, it’s easily searchable. Downstream, we can tie it to all the information that comes from the systems engineering team, the IT patrol systems, the space dynamics team, that it’s basically reciprocal.
We provide to them information from our simulations and they feed us back conditions that we need to use for our simulation. So, having all these initial conditions, reports, test data, traceable basically in a single thread where you could find out exactly from each functional team what is the input and the output, it’s invaluable.
Chad Ghalamzan:
I mean, just one of these vehicles would be a massive project, but we’ve talked about several different ones that you’re working on. I can only imagine that if you didn’t have tools like Teamcenter Simulation and now AI and machine learning to help you organize the data, keep it structured, keep it retrievable, keep it traceable, keep it up to date and shareable across the organization, and then actually do some of the analysis, how would you scale your projects and try to go to faster without some of this technology?
Tom Stoumbos:
It would take twice as long and it would increase the probability of having errors in the process by, I would say, tenfold.
Chad Ghalamzan:
Tenfold.
Tom Stoumbos:
Because when you automate, you have a verified and validated script that you know it is not going to introduce any error in the process. So, every time a human touches any part of that process besides the occasional check, like the auditing of the data to make sure the process is correct, there is a chance of human error being introduced, that it’s going to basically follow that whole thread all the way to the end, that it’s going to be very expensive. Of course, it’s going to cause delays and the cost of a major fix. So, automating the process with having all those tools talk to each other, having all the functional teams through the tools talk to each other is invaluable.
So, as we increase the power of the computers through the computer processors, through the graphics processor and the allowable space within the computer hard drive that’s scalable into the cloud and with high power computer, you want to generate more data and reduce the risk of having emission that will fail or hardware that will not be able to perform. With that magnitude of data that you have to deal with these complex missions, you need to rely on the tools and the algorithms that you’re going to use to process timely and have a high probability of getting to an optimum solution or else you’re not going to be able to complete the project.
So, we see it as one-way street that there’s no going back to the traditional ways of doing. So, technology is enabling us to produce things better, faster, and we should stay the course.
Chad Ghalamzan:
Why space? What is it about space vehicle, space exploration that appeals to you?
Tom Stoumbos:
It’s because it’s so difficult. One stepping stone doesn’t necessarily lead to an easy step forward. Like we mentioned, lunar exploration was a success of the ’60s. We worked hard together with a lot less resources to accomplish something inconceivable and now 50 years later it’s still difficult. So, exploring space, it’s extremely hard. In addition to that, the ability that the space or using space is giving us with internet of things with remote connectivity, right? Having the ability to reach even remote areas with data and information and it’s exploring even within Earth, it gives us that ability.
Of course, it needs to be properly managed and needs to be regulated and controlled. So, it doesn’t get out of control, but for good reasons, space is a challenge to explore and a challenge to develop a mission that you can be 100% sure that it’s going to be successful. That makes it exciting.
Chad Ghalamzan:
I have a four and five-year-old boy, and in their room, I have a solar system on their wall. They’re very fascinated with the planets and space and rockets and all that right now. I try to talk about my work with them in a way that they can understand. I sometimes point to the moon and I say, “Daddy’s going to talk to someone who’s going to maybe one day put people on there.” They ask, “Tomorrow?”, and I’m like, “No, not tomorrow.” But do you think that’s something that’s going to happen in our lifetime, my kid’s lifetime? Whose lifetime do you think it’ll be before we see people colonizing or at least some permanent structure on the moon, which is an enabler for, like you said, going further in the…
Tom Stoumbos:
I think it’s going to be challenging because of the adverse conditions. We don’t know what the challenge is going to be once we start building the infrastructure there, but I’m pretty confident in the next few years we’ll be able to complete that lunar orbiting lab, which is going to be the stepping stone to start a little bit more easy missions, instead of launching from Earth all the way to the moon, to have that lab as a station where crew can go do experiments.
At the same time when they need to land to the moon, they can just land and go back to the station and better understand the conditions of the lunar surface. That would help the engineers here on Earth to engineer better products that maybe in the next 15 years we’ll be able to talk about starting that infrastructure that will take us beyond.
Chad Ghalamzan:
Before we end our conversation, is there anything else about Realize LIVE so far that you’d like to share? Have you enjoyed the experience? Has it been a good conference for you so far? I know it’s still only day two.
Tom Stoumbos:
I attended Realize LIVE a few years back for the first time, and I think the idea of bringing people from different industries, using similar applications, and engaging into exchanging ideas about their accomplishments, about their challenges is invaluable. There’s so much you can do from the office. I’m a firm believer that when you bring people together, great things happen.
The environment like Realize LIVE where you can really engage with the experience and the challenges people from other companies have had using same or similar tools is a game changer. It allows you to go back, rethink the way you’re doing things, and introduce processes, tools that you did not even know exist. So, events like Realize LIVE are invaluable. Bringing the technical and science community together is invaluable.
Chad Ghalamzan:
Well, it’s also because of great presenters like yourself that make it such a great event. Thank you so much for your time and insights today. It’s been a wonderful conversation. I really enjoyed it and I look forward to having you come back on when we have more developments. Maybe when we get that lunar lab, that would be great to talk to. Yeah. Thank you so much for listening to this episode of the Engineer Innovation Podcast. Please click follow to never miss a new episode and leave a rating if you’ve enjoyed what you’ve heard. I’m Chad Ghalamzan and I look forward to speaking to you again next time.
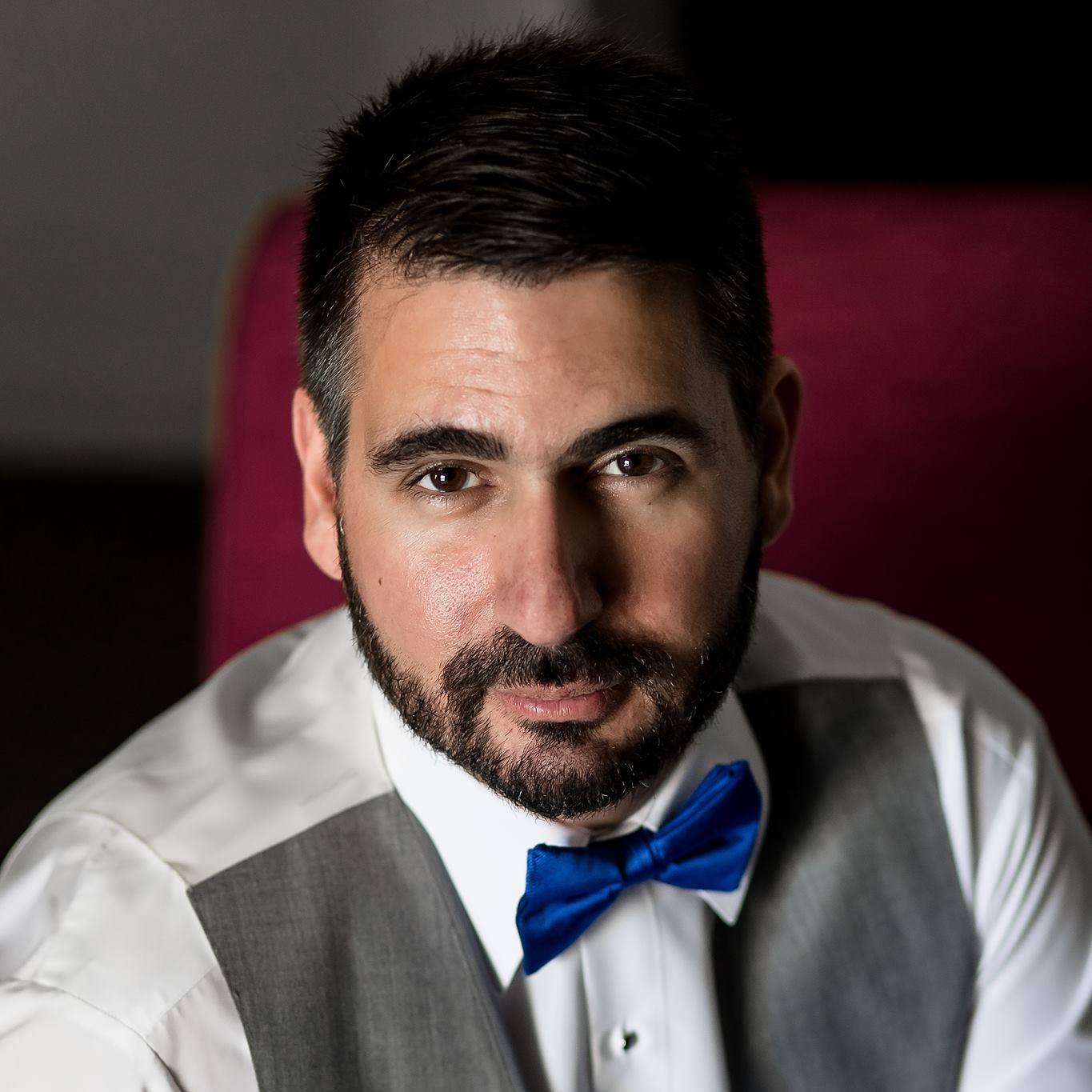
Chad Ghalamzan – Host
Chad Ghalamzan is a computer engineer with over two decades of experience in sales and marketing for the simulation and test industry. He co-hosts the Engineer Innovation podcast and creates content for Siemens Digital Industries Software. He’s tired of people calling him ChadGPT.
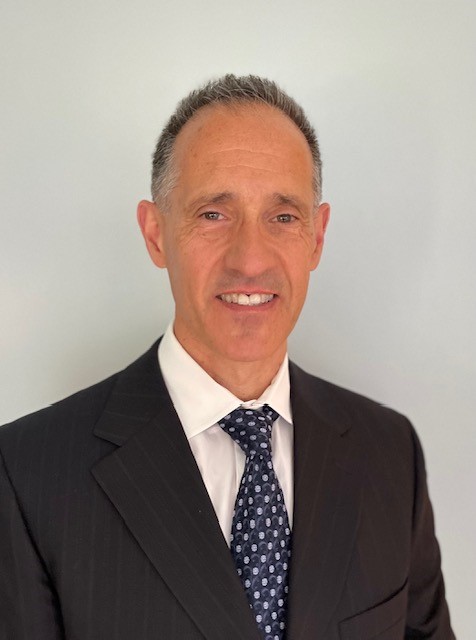
Tom Stoumbos
Dr. Tom Stoumbos has over 25 years of experience managing challenging projects in the Marine, Space and Defense Vehicle industry. Offering a rare blend of technical expertise and team leadership, Dr. Stoumbos achieved great success throughout his academic career and continues to do so in his professional career. Areas of expertise include product management, business development, program planning and project management, evaluating cost/risk, and earned value assessment. Dr. Stoumbos has a background in Computational Structural Dynamics and Composite materials, and works with high profile clients such as DoD, NASA, NRL and ARL. Dr. Stoumbos is with Northrop Grumman Tactical Space Systems since 2004 where he currently leads the Simulation & Test teams responsible for Space Vehicle Engineering Simulation Design Analysis & Test for IRAD, commercial, defense and science satellite programs
Take a listen to a previous episode of the Engineer Innovation Podcast:Engineer Innovation: Advancing corrosion analysis in aerospace with Alan Rose of Corrdesa LLC on Apple Podcasts
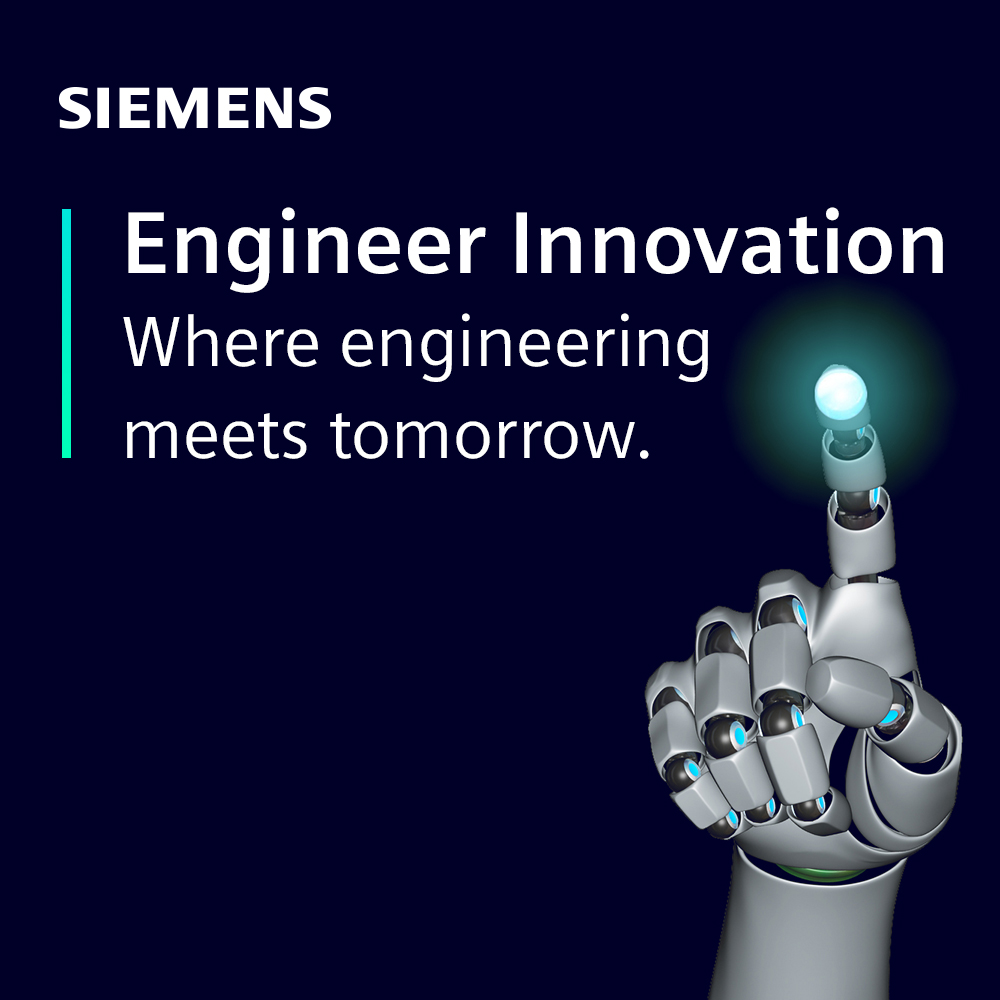
Engineer Innovation Podcast
A podcast series for engineers by engineers, Engineer Innovation focuses on how simulation and testing can help you drive innovation into your products and deliver the products of tomorrow, today.