100,000 Simulations a Day! AI Powered Simulation with PhysicsX
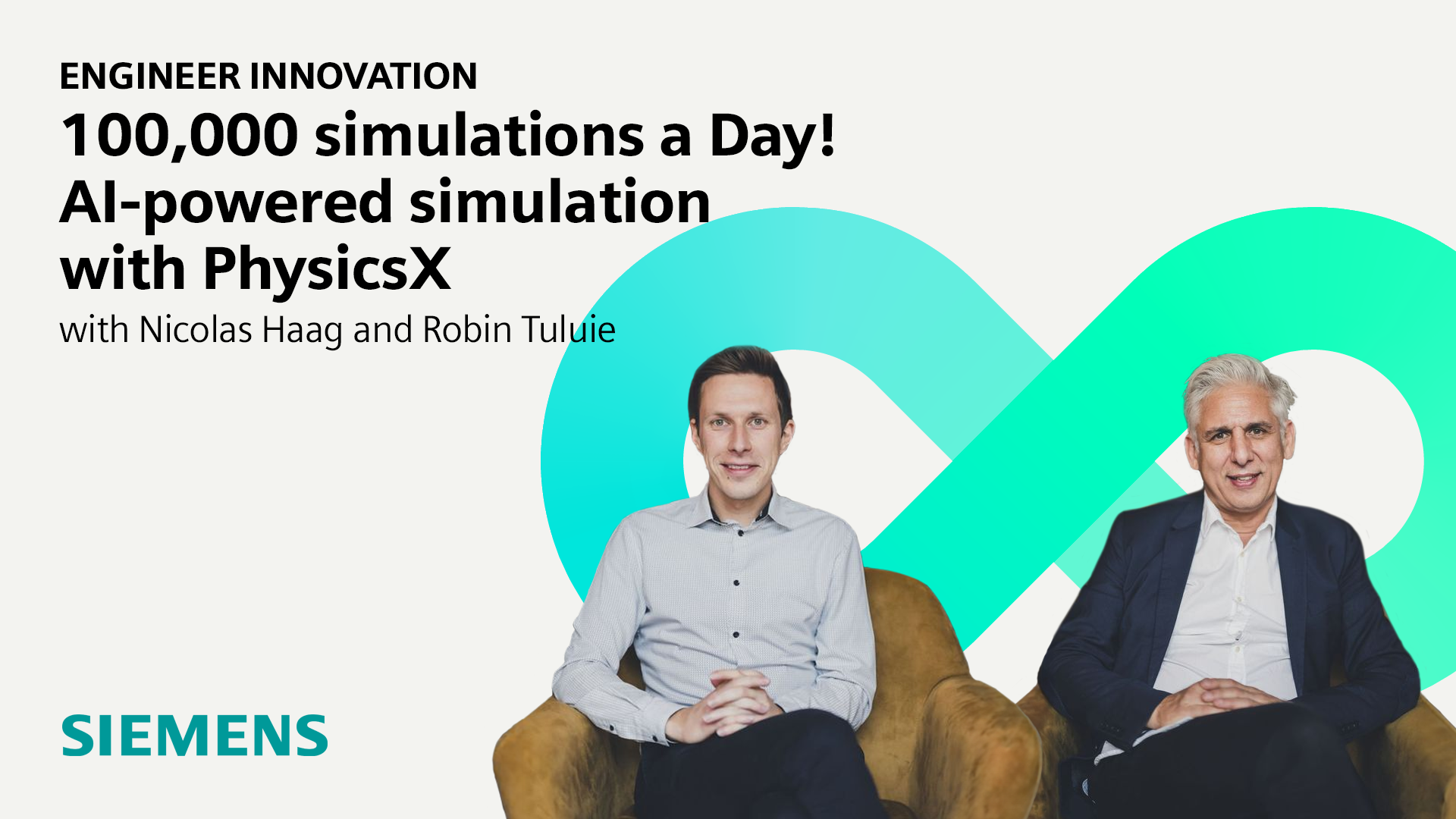
Guest Nicolas Hagg and Robin Tuluie
Listen on Apple
Listen on Spotify
Show Notes
In this episode, listeners are provided the clearest view of the future. And what’s better is that it’s available today.
Robin Tuluie and Nicolas Hagg explain it better than I can, but PhysicsX use “Deep Learning Surrogates”, which are AI models that are trained using engineering simulations (such as CFD or FEA), but are then capable of – almost instantly – producing thousands of “CFD quality” surrogate solutions. It’s the closest we’ve ever got to “real time CFD”.
I started off this interview by being slightly skeptical about some of the claims – after all there is an enormous amount of hype surrounding AI – but by the end I was utterly convinced that the sort of technology that Physics X is set to absolutely revolutionize, and massively increase the impact of engineering simulation, and its ability to solve the huge challenges that our species is facing.
This episode of the Engineer Innovation podcast is brought to you by Siemens Digital Industries Software — bringing electronics, engineering and manufacturing together to build a better digital future.
If you enjoyed this episode, please leave a 5-star review to help get the word out about the show and subscribe on Apple or Spotify so you never miss an episode.
Guest biographies and episode transcript
- Explore the future of engineering simulation, focusing on Machine Learning, Artificial Intelligence, and the Digital Twin.
- A clear vision of the future of engineering simulation, emphasizing that such advancements are already accessible.
- Robin Tuluie and Nicolas Hagg discuss PhysicsX’s use of “Deep Learning Surrogates,” AI models trained with engineering simulations like CFD or FEA.
- Discussions on how AI models can produce thousands of high-quality surrogate solutions almost instantly, approaching real-time CFD capabilities.
- PhysicsX’s technology promises to tackle significant global challenges through advanced engineering simulation solutions.
Robin Tuluie:
The difference is that these models are a lot faster. So, you show them a geometry or an operating condition of both, and you have a result at the level of a simulation result, so meaning scalars on pressure fields, velocity fields, vector fields in a second or less, not in hours. That just changes the way that you think about optimization and search completely. Our customers can do 100,000 simulations in a day. No problem.
Stephen Ferguson:
My name is Stephen Ferguson and you are listening to the Engineer Innovation Podcast. In this episode, I talk to Robin Tuluie and Nico Haag from PhysicsX who are in their own words on a mission to reimagine simulation for science and engineering using AI with a strong focus on applications impacting the climate and human health. Now, in the last 12 months on this podcast, we’ve talked a lot about the impact of artificial intelligence and machine learning on the future of engineering simulation, and often that’s included a fair amount of speculation about the shape that influence will actually take. So, hopefully, you’ve just heard that intro in which Robin Tuluie describes how PhysicsX customers can run over 100,000 simulations a day.
Now there’s a lot of hype around AI at the moment. So, obviously, I started off this interview by being rather skeptical about those claims, but by the end of the interview, I was utterly convinced that the technology that PhysicsX are developing is set to absolutely revolutionize and massively increase the impact of engineering simulation and its ability to solve the huge challenges that our species is facing. Before founding PhysicsX, both Robin and Nico reached what many of our listeners will regard as the absolute pinnacle of engineering simulation with prominent careers in Formula One. So, I started off by asking Robin and Nico to describe their career journeys to this point. The first voice you’ll hear is Robin Tuluie, who is the founder and co-CEO of PhysicsX.
Robin Tuluie:
My background is theoretical physics, in particular numerical physics applied to gravitation and related applications of gravitation and cosmology. That is a long journey and a slow development pace because you’re waiting for decades for observations to validate your models and your simulations. Being a bit impatient, I gravitated towards Formula One where you find out every weekend whether what you’ve developed beforehand was right or not. So, it’s a very fast learning cycle. Quite early on in that learning cycle, experience only gets you so far, so does lab or track testing. So, the development of simulation approaches across the whole car. So, really multi-physical simulation is really important.
Then in 2011, I joined Mercedes F-1 as chief scientist and head of R&D, and then 2016, Bentley as we call technology director. At Bentley, my responsibility was across our simulation strategy and activities, which means the engineering department operates over 30 different digital prototypes to inform the attributes of the car during that virtual development cycle. I left Bentley in 2019 to found PhysicsX, and Nico joined me in that journey. So, Nico, would you go ahead and introduce yourself?
Nicolas Haag:
Yes, of course. Very happy to. So, my background is basically in automotive racing and a bit of aerospace engineer by training and pretty soon, as Robin said, got into machine deep learning, what we’re basically doing today. What my role is mostly bridging delivery, research and product, taking care of our whole engineering workflow from an end-to-end perspective and basically bringing in the customer-centric perspective of doing that for the last four years and feeding that back into what we’re building today for our customers.
Stephen Ferguson:
So can you tell us about PhysicsX, who you are, and what you do?
Robin Tuluie:
Yes. We’re a company that’s trying to change the way engineering is practiced today and we know the pain points of that because this is the journey that we have lived over our careers that we have experienced. There are opportunities which are unfolding now. Simulations take time. These time constraints invariably lead to optimization constraints. Even the way that you perceive of a design now means that if you want to improve this design and verify that in your simulations, you are conceiving of quite a narrow design space. So, let’s say a few parameters or a few operating points rather than the whole operating cycle in order to test out and optimize your design.
That lack of blowing up the search space means that across the whole operating map and geometrical space, you don’t find the best-performing geometry or setup of your product. That is what we are changing and we’re using machine learning, particular deep learning to change that. We change that through a platform that we’re building through applications that sit on top of that platform that are sector-specific that allow us and our customers to build into the space.
Stephen Ferguson:
So lots of it is about accelerating the pace of engineering simulation, engineering development. How exactly do you do that? I’m sure you can’t tell us exactly because it’s probably a commercial secret, but can you give us some idea about how that process works?
Robin Tuluie:
Absolutely. We train deep learning models that replace physics as a whole. So, these large physics models are deep networks which are trained on simulation data and on experimental data. We have choice of training data. We require high quality data and there’s a validation process for that data that has to happen, of course, but then we use those archetypical data types to train these deep learning models. These deep learning models can then emulate the physics that happen within a simulation and they can do so to a high level of accuracy. So, over the years, we have now well over 40 proof points across advanced industries of how well they perform.
We not only know how well they perform, we also know how to shape various types of models so that the outcome is the highest performance and fastest executing outcome. Now the difference is that these models are a lot faster. So, you show them a geometry or an operating condition of both and you have a result at the level of a simulation result, so meaning scalars on pressure fields, velocity fields, vector fields in a second or less, not in hours. That just changes the way that you think about optimization and search completely. Our customers can do 100,000 simulations in a day, no problem.
Stephen Ferguson:
That’s incredible. So, lots of our listeners will be familiar with surrogate models, things like response services and reduced older models, but this is something a bit more fundamental. So, you’re not just getting numerical quantities like coefficients of drag or pressure drops or forces or temperatures. You’re actually getting complete flow fields and scalar fields.
Robin Tuluie:
That’s absolutely right. These models are fundamentally different to the ones that you mentioned, like reduced order models, because again, in a reduced order model, you’re trading on a subset of parameters and you get out some scalars afterwards. These are geometric deep learners and these are relatively new constructs. They have been around for a handful of years and they’re being discovered in academia different approaches over time here. It’s a rapidly evolving and expanding field and we are very attuned to that. We build our own foundation models or we build on top of other foundation models in order to fight the best solution for a particular application, but they’re fundamentally different to traditional.
Stephen Ferguson:
What hardware is working behind the scenes for the deep learning stuff?
Robin Tuluie:
Nico, do you want maybe answer that though? Because I don’t want to hog the airspace.
Nicolas Haag:
No, of course. I mean like normal machine learning, we are using GPUs and channel like STAR-CCM+ now runs on GPUs. We use the same hardware. It depends of course on the model and what we’re training on, the number of measures and elements, but in general, it runs on a normal GPU.
Stephen Ferguson:
So from an engineer’s perspective, somebody like me who’s a gnarly old CFD engineer, is this a technology that people like me can access or is it only accessible by people who are data scientists and have newfangled AI and machine learning skills?
Nicolas Haag:
Very good point, Stephen. This is exactly what we’re setting out to do. This needs to be accessible to engineers without requiring a lot of coding expertise around these workflows. In the end, it’s the engineers doing the engineering, knowing everything about the application and the respective constraints. On the other side when there are data scientists, engineers bringing coding experience, awesome, especially when workflows require high level of customization, but it’s not a requirement and we can help on that as well.
Stephen Ferguson:
As the world of engineering becomes more and more AI focused, do you think the profile of engineers is going to change? Are engineers going to have to become more like data scientists or are those going to be two individual roles, do you think?
Robin Tuluie:
We certainly wouldn’t want engineers to have to become data scientists in order to operate our product. As Nico said, it’s really important that engineers can operate that and their robust integrated workflows. We love working with Siemens and Siemens’ simulation software because it’s extremely robust. It’s well integrated, so we’re aiming for the same type of quality in our products. Our product has to be accessible to both. The reason is that engineers are the main users of these products, but data scientists build the backbone of the methodologies and specific applications.
So, over time, companies are growing the data science pool and we offer both options. So, an approach where engineers can operate this without having to be data scientists and the ability for data scientists to build on top of that or alongside of that.
Nicolas Haag:
Just adding to that, there is a lot of good stuff from the software engineering world, from how data scientists or in channel software engineering has done today in terms of DevOps, ML ops, where we as engineers can learn a lot and what we’re also integrating into our platform to streamline processes, create workflows, have better version control aligned with what Teamcenter offers on the Siemens side, but to make this much more streamlined and accessible to everybody in the company, which currently is not always the case unfortunately.
Stephen Ferguson: Which is really important, isn’t it? Accessibility to this technology is one of the issues because I think there’s lots of dystopian fear that AI can replace engineers, but of course, there’s not enough engineers, enough engineering in the world to solve all the problems. So, I guess that’s one of your ambitions, to make engineering more accessible and more productive that we can start to tackle some of these big problems.
Robin Tuluie: That’s exactly right. This technology doesn’t just have the potential. It’s already starting to transform some of the important or meet and exceed some of the important challenges that we face today around renewables. We’ll talk a bit later on the medical application, in aerospace space, and other important sectors where we can have a real impact on the world and the quality of life within that world.
Stephen Ferguson:
Which is a very impressive ambition. So, in terms of the amount of simulation that goes into these deep learning surrogates, can you tell us a bit about how much simulation people have to do to train the models?
Robin Tuluie:
Very little, and this is another fundamental difference to say reduced order models. We need relatively little training data and by an order of magnitude less. The reason is because we learn from every mesh element. There’s always an association between that geometry and that locality and the pressures and flows and temperatures or stresses that that produces. So, while the number of simulations is far fewer, what the data generated by these simulations by each one is a much larger data set, because we’re now talking about volumetric and surface data. We exploit the size of that data set fully.
Stephen Ferguson:
Which is what is truly revolutionary about this approach and very different than anything I think that’s been before it. Are you familiar with computational irreducibility, Stephen Wolfram’s idea that in solving anything other than very simple problems, you have to go step by step? You can’t take big leaps forward. I guess your algorithms are still going on a step by step basis and iterating towards solution and not taking the big leaps, or am I wrong in that?
Robin Tuluie:
So absolutely, we’re going step by step, Stephen. This is a journey, this is a transformation. If you think about the transformation that we all witnessed from physical testing to virtual testing, multiple decades, but now you couldn’t lead product development unless you’re doing virtual testing, unless you’re using simulation to develop your products. We’re witnessing the same thing. Again, I was fortunate enough to witness that transformation and now very fortunate to be co-leading together with a lot of other entities in this world that we are co-leading this next transformation, which is one, from explicit simulation to machine learning and deep learning replacements of those things. It takes time.
There are a number of great challenges that we can solve right now, and there is a number that we can’t. We want to reduce training data requirements. We want to reduce the computational workload during training. We want to increase inference speed. We want to increase the geometric source space. All of these things are fundamentally important and that’s an incremental journey, but it’s fast. This isn’t going to take decades. This is a journey where we get to some really valuable outcomes within years.
Stephen Ferguson:
Because the nature of the problems we’re trying to solve, climate change and human health, we don’t really have decades, do we? That’s part of the reason why we need to do these things. It did strike me when I was learning about what you do and listening to some of your other podcasts and appearances is that as simulation engineers, we reduced or we demoted engineering testing sometimes to being a validation tool for our simulations. You did all simulation, and at the end, you tested your simulation results using testing. It occurred to me actually that simulation, you’re going to be using that to validate the outputs of some of your model. I guess you go back and check quite often to make sure the deep learning algorithms producing results which are consistent with doing simulation.
Robin Tuluie:
Absolutely. As a starting point, you can use simulation to a well-validated simulation model and train on that and your model will be as accurate as that simulation model or close to. There’s high correlation but not perfect correlation obviously. But even those simulation models, the proof in the pudding is that they’re validated against real world data, whether it’s test lab data or in-service data. The beautiful thing about machine learning, deep learning is that you can train on both data sets, so you can also train on that experimental data and that gives you a path to validation, which is different than that of a simulation model validation path, which involves a lot of recipe tuning and fine tuning and expert knowledge.
Stephen Ferguson:
So before we move on to talking about the applications, is there anything else you’d like to add about what you’re doing?
Nicolas Haag:
There are some more applications. Of course, the closest one is high dimensional optimization, but if you’re getting into what Robin explained on getting to fractions of second of inference time, you can even think about getting into control, even to some extent real-time control. That’s one big thing. Of course, design optimization, a big part of that is manufacturing. We are now building end-to-end workflows, including manufacturing in the loop, which is of course mostly important. It’s all about solving real world problems. From the world we are coming, we do that for four years and we learned a lot and all that feeds back into what we’re building now with platform. This is so important for us.
It’s not just something that looks cool like a lot of other tools offer but in the end doesn’t work. It’s really we’re doing something that actually makes a difference and impact. You mentioned step change, and in some of the application, we’ve seen a huge step change. While of course the evolvements in AI for engineering are going step-by-step, but adding up, this becomes pretty huge if you look at the applications.
Stephen Ferguson:
So because you can run these models in almost real time, I guess you need quite a lot of computational power to train the models, but when you are deploying them, I guess that’s quite a lot smaller. So, is one application of this in things like the Executable Digital Twin where we can run the surrogate models on edge devices to perform in the loop applications as you mentioned?
Robin Tuluie:
We’re still operating on GPUs, which you wouldn’t have, let’s say, a car ECU, right? You wouldn’t have an 8100 in your car ECU. So, there there’s some limitations to edge compute, but over time, these things will merge. These models will become more easily handleable. For certainly industrial applications, control systems can carry a higher amount of compute than your average car ECU does. That’s a really fantastic thing. If you think about model in the loop, the types of models that are being operated now are really simple models, aren’t they? For control systems. Here we can have a representation at the level of quality of the highest fidelity simulation that you can imagine.
Stephen Ferguson:
Yeah, that’s really, really exciting. I’ve been in the industry for 30 years. Thirty years ago, real time simulation seemed like a pipe dream, didn’t it? But the fact that during our careers we’re not very far away or maybe even achieving that is an incredible, incredible thing.
Nicolas Haag:
Yes, yeah, exactly right. As you said, there’s also difference between training and inference, of course. Of course, for the training, you want to have GPUs. Usually, you get this on cloud or on-prem, but the big point is for inference, you can run on much leaner hardware, say even to the point where you can run on a CPU. We can run models on a little laptop, also running it about seconds. Exactly. So, you don’t need these high-end GPUs always, especially on inference and getting into real time control. You can run it much leaner hardware.
Stephen Ferguson:
So you’ve both come from the Formula One industry. One of my favorite books is by a guy called Nate Silver who predicts the outcome of elections. He’s written a book called The Signal and The Noise where he looks at different types of forecast and looks at volcanologists and sport betters and economists and meteorologists. He comes to the conclusion that meteorologists are the best forecasters because if they predict it’s going to snow and you walk out the next day and it’s sunny, then they know that there’s something wrong with their model and they have to try harder as well.
I guess you come from that in a Formula One world, and I guess what this is going to give us is the opportunity to make predictions much more quickly and give us more information to adjust our models and move quicker. So, basically, are you bringing that extreme forecasting ability from Formula One to more different real world applications?
Robin Tuluie:
Stephen, Nate Silver is awesome, and I followed him since his FiveThirtyEight website many, many years ago. I love the analogy of meteorologists because they’re proven right or wrong every day. Formula One is a bit like that, but there are limitations to what you can do in Formula One at the level of compute, at the level of resources that are part of the regulations of Formula One. What we find is that in industry, those restrictions don’t exist. Still compared to large language models, our training efforts are minuscule relative to that. So, that’s really not a driver of anything. It’s that rapid experimental testbed that exists at Formula One but can also exist when you work with the right partners across industries where we learn together.
That’s important because we as a company have learning to do still and it’s a never-ending journey of learning, learning about our customers, learning about their pain points, learning what works, what doesn’t work, how our products can be better. That’s what we extract. They have the ultimate proof in their experiments and their product performance when they measure it or on their simulation models when we compare against those.
Stephen Ferguson:
Excellent. So, I’m sure that there’s lots of applications of this technology that you can’t tell us about, but one which you can talk about which addresses one of your core motivations is a healthcare example, isn’t it? Which is I think a mechanical heart. What can you tell us about PhysicsX and the mechanical heart?
Robin Tuluie:
This is a company that we’ve been working with for three years now. We started the journey using STAR-CCM+ to simulate the blood flow through this artificial heart, which is just a mini Francis pump, so essentially an impeller that spins and replaces the pumping heart. You still have the heart, but the heart muscle is diseased. That doesn’t pump anymore. So, this little pump, which is about the size of your thumb, takes over. People survive without a heartbeat. Now this is a development. This is not the very first one. There’s one currently in existence, but this is a development that really changes the whole spectrum of patient quality and patient care. These things have a cable coming out of your skin which leads to infections.
While they achieve the necessary flow to pump the blood, the blood gets damaged, the blood can get damaged in a number of ways. It can get sheared, and that leads to gastrointestinal bleeding, which is another trip to the hospital. Or worse yet the blood is not agitated sufficiently, there’s not enough circulation and you get thrombosis, blood clotting, which can lead to a stroke and death. So, it’s the aftercare cost that is driving the cost of these artificial heart implants. In the words of Steven Westaby, who is one of the co-founders of this company, it’s a bit insane that somebody has to die in order for someone else to live. We are a heart transplant and this is what this company Calon Cardio is seeking to change.
What we’ve been able to do is help them achieve a significant step-up in the performance of this pump while reducing the blood damage metrics. We’ve been able to do that by replacing the simulation with our deep learning model and running that against a number of target metrics, which include flow performance, which include all the blood damage metrics and optimize this. The overall gain across the number of different performance metrics equates out to about 42% over the baseline. So, now this pump can be implanted without a cable, because it has lower power requirements, it is charged. We are transcutaneous power transfer device, essentially like your microwave charging device for your foot. That means no infections, it has less blood damage, and therefore also lower aftercare cost, causing much better patient quality of life.
Stephen Ferguson:
Which is an absolute game changer, isn’t it? That makes that technology much more feasible and will save I guess many thousands of lives and improve the quality of lives of people who do have heart disease. So, what specifically was PhysicsX’s contribution to that project?
Robin Tuluie:
I think Nico should answer that. I’ll just make one more point, which you just said. There is a step that you can’t achieve traditionally and that step up that we can achieve with deep learning with this mass optimization, we’ve seen that in other industries where all of a sudden something that wasn’t viable before now becomes viable. Let’s say at either cost viability or performance viability now puts this product on the map and that’s truly transformational.
Nicolas Haag:
So from an engineering perspective, as Robin said, multi-objective optimization is highly complex, of course. To get to the maximum, you need to optimize the whole system, which is about, I think, 50 to 100 of parameters, something you can’t optimize otherwise, especially not using a safety workflow. Even if it’s fully automated, it’s a lot about efficiency, but also, you want to make the pump as small as possible. In the end, you also want this to implant into kids and you don’t want to change the pumps if they grow up. Blood damage is a very important point and also something where STAR-CCM, for example, really helped us to make that really easy and fast to simulate and solve and create high-quality training data.
As Robin said, another one also, stagnation. We need to limit blood heating, limit vibrations, and there’s a lot of more things, a lot of constraints. It just gets extremely complex and the only way here is to use deep learning models to help us to look through that whole design space, go through millions of different design combinations, and really find the global optimum in something that is not violating any of these very important constraints.
Stephen Ferguson:
How much faster were those simulations using your PhysicsX platform than would be somebody who was just using traditional numerical simulation?
Nicolas Haag:
So we actually run a lot of CFD simulations over… We had some holiday, so we did I think 1,500 of that. But if we came back, we were still not near any optimum and this was the reason basically we started on let’s apply the deep learning model. Just to give you an idea, I think the holiday was two weeks long. We’ve got our own HPC 500 cores. We got the simulation time down a lot, fully, fully automated using heats in the loop and everything, but even that was not enough. Then with the deep learning model, it took us, I think, about 6 to 12 hours to really find the global optimum and this is really the power of these tools. As soon as you’ve got a good model, you are there in a couple hours really, and this is still pretty amazing.
Of course, then we are still engineers. We are running things back into CFD and it matched up nearly perfectly and created a geometry or a configuration, which none of us would’ve came up with. But for some reason, it works, and this is the amazing thing of using these AI models is really beyond what an engineer could come up with and explore the whole design space.
Stephen Ferguson:
Talking about quality of life, when you’re talking about going on holiday for two weeks and running hundreds of simulations, that’s not any real holiday, is it? Because you have to check in and make sure everything works. I guess being able to run these huge design exploration examples during a working day is a massive step forward for the quality of life of engineers as well, I guess.
Nicolas Haag:
Yeah, definitely. I think here that’s why we are using Siemens STAR-CCM+, because for us, it’s the most reliable. I think with these 1,500 runs, which by the way, we didn’t need to train the model. In this case, that’s much lower. As Robin said, we usually need 50 of them or something for high dimensional design space. But this run all fully automated. I think there were no errors at all annexed or connected to STAR, but in general, if you look at legacy workflows or even workflows today, there’s a lot of things, meshing errors, solving errors, geometry errors and internal hardware, hardware issues. Using our platform, you can completely bypass these issues.
Stephen Ferguson:
So we’ve talked a lot about fluid dynamics examples and computational fluid dynamics, but I guess this technology is applicable to all sorts of numerical simulation, isn’t it?
Robin Tuluie:
Absolutely. We’ve deployed this across the advanced industries, so across multi-physics and even into chemistry now. We are learning about proof points every week and it’s surprising. The really surprising thing is that there’s a generality to these models across physics. The way I solve a CFD finite volume model is very different than I saw those stress finite element model if I want optimum solutions, but that’s not true. We can have the same model architecture solve a CFD problem and solve an FV problem.
Nicolas Haag:
Even going further than at this point where you’re not constrained to one physics like a solver is and you need to do some tight coupling of different solvers if you talk about [inaudible 00:31:35]. With the deep learning model, as Robin said, you just need one architecture and you can predict both. You can have true multi-physics models, which can cover all sorts of different physics and applications.
Stephen Ferguson:
Humanity is facing some really big challenges. If you look at your company statement, very specifically you talk about healthcare and climate change, which are two of the biggest problems we are facing. I think the two biggest. I guess agriculture is another one, feeding 10 billion people. This is generally just a philosophical question about how much you think that engineers and humanity are going to be able to solve these problems without using laws of AI and ML. Is AI and ML essential in solving these big challenges in your views?
Robin Tuluie:
Certainly an urgent problem, isn’t it? What you mentioned around the climate challenge, around human health and feeding the world’s population, there’s a real urgency to that. AI accelerates our solutions, accelerates the efficiency of wind farms, accelerates the ability to create synthetic fuels, to replace fuels in technologies or sectors where it’s difficult like aviation for example. So, it’s an accelerator, just like simulation is an accelerator. This is the next click of the throttle pedal. It’s the dial 211 of Spinal Tap.
Stephen Ferguson:
Thank you for that reference. That’s definitely staying in. So, finally, obviously, you’re a company which is trying to solve some of the biggest problems using the most advanced technology that I think that our species has to offer. How do you find the talented people to help you on that journey?
Robin Tuluie:
It is a challenge, but we’re very fortunate that people are excited about our mission and they’re excited to work with like-minded peers. All profiles tend to be around data scientists, machine learning engineers, simulation engineers, software developers, and this composes the majority of our technical practitioners. We like that people have a multidisciplinary background. Having an engineering background and finding yourself working in machine learning and deep learning by a way of necessity is a common profile of people that apply to PhysicsX because indeed it’s another great tool in order to help us achieve better products and better performance of those products.
Stephen Ferguson:
So people can find PhysicsX at their website, which we’ll drop in the show notes. I’ll also, if you don’t mind, drop your LinkedIn profiles so they can keep in touch with what both of you guys are doing. I think we’re out of time. So, it just remains for me to thank you both for being such excellent guests. I feel utterly inspired by that. Thank you to everybody for listening to the Engineer Innovation Podcast.
Robin Tuluie:
Thank you, Stephen.
Nicolas Haag:
Thanks for having us.
Stephen Ferguson:
Thank you for listening to the Engineer Innovation Podcast, powered by Simcenter. If you enjoyed this episode, please leave a five-star review and be sure to subscribe so you’ll never miss an episode.
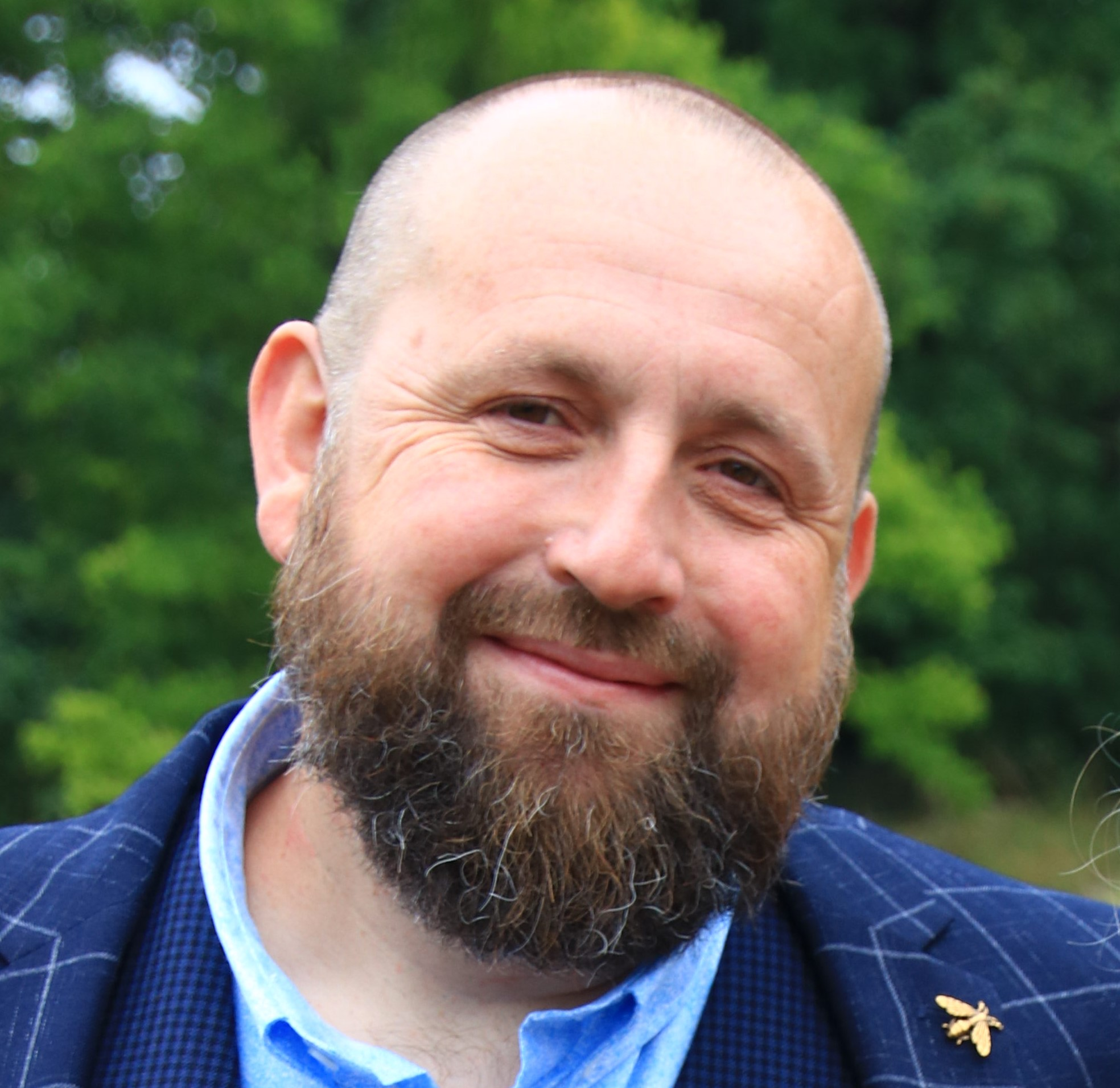
Stephen Ferguson – Host
Stephen Ferguson is a fluid-dynamicist with more than 30 years of experience in applying advanced simulation to the most challenging problems that engineering has to offer for companies such as WS Atkins, BMW and CD-adapco and Siemens.
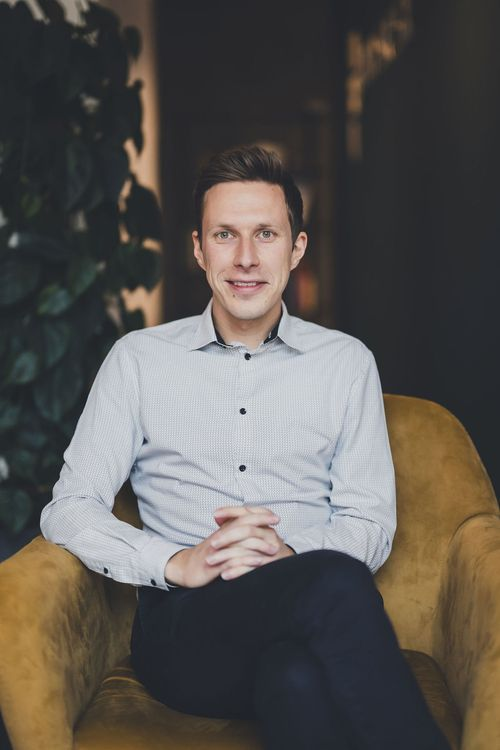
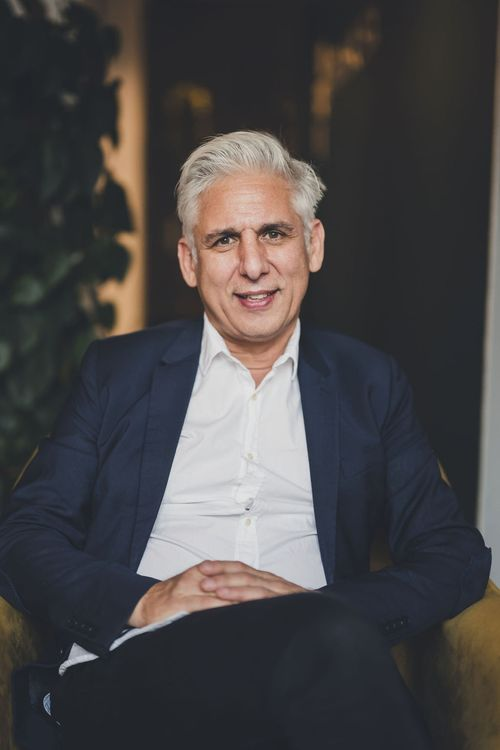
Robin Tuluie
Robin is the founder and vice chairman of PhysicsX. He leads the business across science and engineering in his role as CSEO, and our board’s technology strategy. A theoretical physicist by background, Robin left academia for the fastest development cycle on earth, Formula One. He was Head of R&D at Renault (Alpine) F1, where he developed groundbreaking innovations that helped the team win back-to-back double World Championships. In 2011 he joined the Mercedes F1 team as Chief Scientist and Head of R&D, developing innovations with multi-physics simulation tools and machine learning optimizations. After two more world titles with Mercedes, Robin joined Bentley Motors as Vehicle Technology Director, responsible for the overall simulation strategy and digital twin roadmap, while advancing simulation with Ducati MotoGP and across the Volkswagen Group, before founding PhysicsX in 2019.
Take a listen to a previous episode of the Engineer Innovation Podcast: Engineer Innovation: Advancing Healthcare Technology Through Simulation with André Gasko of B&W Engineering on Apple Podcasts
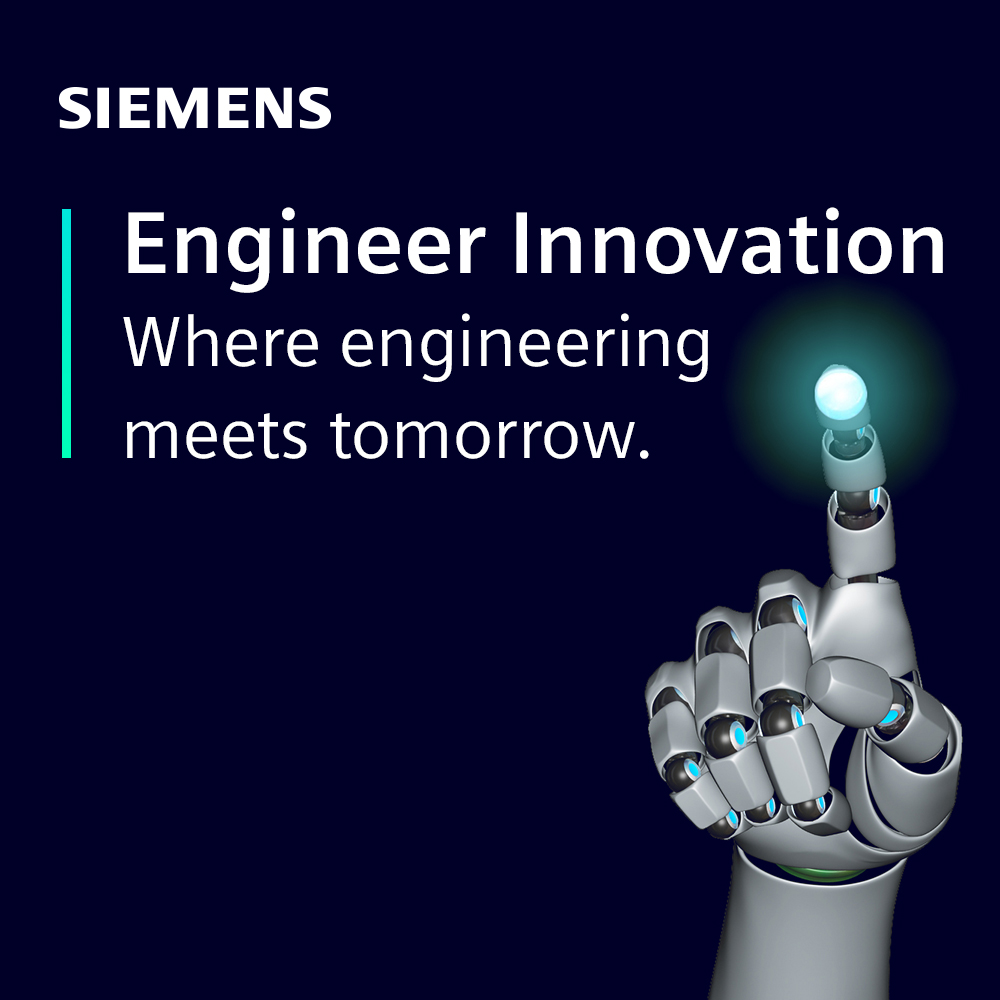
Engineer Innovation Podcast
A podcast series for engineers by engineers, Engineer Innovation focuses on how simulation and testing can help you drive innovation into your products and deliver the products of tomorrow, today.