The Role of Simulation Engineers in Our AI Tomorrow

Guest Dr. Gabriele Pozzetti
Listen on Apple
Listen on Spotify
Show Notes
In this episode, join us as we explore the intersection of artificial intelligence, engineering and innovation with Dr. Gabriele Pozzetti, Reformed CFD Engineer and AI Expert. With a rich background in both the theoretical and practical aspects of AI, Gabriele provides insights into how AI is reshaping industries and what the future holds for engineers and technologists alike.
Key Takeaways:
- Gabriele explores AI’s transformative impact on engineering, highlighting innovation and the development of new solutions.
- Insights on integrating AI into digital strategies underscore its pivotal role in enhancing operational efficiencies and fostering innovation.
- Discussion on ethical AI and future trends emphasizes responsible innovation and the importance of ethics in AI’s evolution.
This episode of the Engineer Innovation podcast is brought to you by Siemens Digital Industries Software — bringing electronics, engineering and manufacturing together to build a better digital future.
If you enjoyed this episode, please leave a 5-star review to help get the word out about the show and subscribe on Apple or Spotify so you never miss an episode.
Watch on YouTube
Guest biographies and episode transcript
- The journey of AI from niche technology to mainstream application. (02:03)
- Simplifying complex problems with AI. (12:15)
- AI and machine learning in modern engineering. (19:32)
- Developing a successful digital strategy with AI. (25:01)
- The ethical considerations in AI deployment. (30:45)
- Predictive analytics and the future of AI in engineering. (35:14)
- The importance of interdisciplinary knowledge in AI advancements. (40:29)
Gabriele Pozzetti:
In a world of incredible technological abundance, like the one we live, I think it’s important to strike a little bit of a balance between this ability of doing things and generating a part and ability of filtering it out.
Speaker 2:
So Steven, you interviewed Gabriele Pozzetti. Tell me about that.
Steven:
So the best way of describing Gabriele, I think, is a reformed CFD engineer. So he’s somebody who started his career being a CFD simulation engineer and a CFD developer. But he saw the light and escaped, and is now managing AI transformation programs. And what’s really interesting is he’s seen both sides of the coin. He knows what it’s like being a simulation engineer, and he knows what it’s like doing AI transformation, and so he’s got a really good understanding of the intersection between those worlds, and also the areas in which simulation engineers are really strong in AI. But also what some of the deficiencies are, and some of the ways we can improve ourselves.
Speaker 2:
So did he have actual practical experience about using AI, or was it still in the test phase?
Steven:
He works for a company that makes really hard and resilient materials, and so he’s still involved in engineering, he’s just not involved in engineering simulation, as Simcenter people would understand it. He’s applying AI to transform all of their processes, and I think it’s a really interesting story.
Speaker 2:
That’s great, because we’ve had a lot of people come on the podcast to talk about AI, but not a lot of them have had the chance to apply it practically. So it must have been a very good conversation.
Steven:
Yeah, and I think that’s where we’re going, isn’t it? It’s going to stop being a novelty, and start going to be a practical tool everybody has to use. He has some really good advice on somebody who’s new to AI, and the strategy you need to implement it to go onto your digitization journey.
Speaker 2:
Let’s take a listen.
Steven:
Hello, and welcome to another episode of the Engineer Innovation Podcast. Today, I’m joined by Gabriele Pozzetti, who I’m going to describe as a reformed CFD engineer. Gabriele, would you mind introducing yourself for the audience, please?
Gabriele Pozzetti:
Thank you. Thank you for having me here, pretty excited about doing that. So about myself, I’m definitely an engineer with all the perks and downsides that this will look at. Sorry about my voice quality and the weird expression I will use. I actually fell in love with the whole field of computational technologies back in 2012. My first big project on that one was on a simulation of an abdominal aortic aneurysm, that’s a medical condition that affects quite a lot of people, unfortunately these days in the later part of their life. And that idea was to try to simulate how this aneurysm will evolve, which will be the relationship with the blood pressure, both on a three-dimensional and a multidimensional way.
And what really sparked me off that experience, what really made me fell in love, is that I was not really studying bioengineering, I was studying nuclear engineering. And the idea that with basically the same tool set that was actually used and developed to study nuclear reactors, one could bring it to such a different field, that really made me super excited. And it gave me this vibe as younger generation would say, of being a renaissance engineer, like this MacGyver guys that can apply their toolset to anything.
But I also had better [inaudible 00:03:17] to the renaissance, so it was actually a privileged position. So that’s why I went on with a PhD, at that time about multiphase flows and particle-laden multiphase flows, developing new multi-scale models to treat that problem. That landed me a job at CERATIZIT, that is a company specializing in high-tech material and high-resistant materials. And they offered me the possibility of managing a portfolio of projects all about feature prototyping.
So, the idea was to make their product development better, faster, cheaper, this kind of part. And there I learned a very important lesson, one of the most important lessons about my professional career, that is it’s way better to deploy simple and reasonable algorithms faster, rather than keep working on optimizing fancy algorithms that actually did not stood the test of reality. And this is what opened my mind from a more technical CFD guy to general, I say, digitalization role in which I was willing to use any computational technique that would make sense for the specific occasion.
And this was also how I got early in touch with the machine learning and those kinds of technology that are extremely powerful. So I started using them more and more because of that. That got noticed. I was offered the chance to build the first artificial intelligence team of CERATIZIT, and that was a bit my step out, my reformation outside the CFD world, and let me enter instead into the leadership role. That was something else that since early on I knew I wanted in my life, but I never of course had the chance. So I also needed to see could I go that direction or not?
So, that was what convinced me stepping out of CFD because they offered me leadership on the other side, another very important quality for me at least. And together with my team then we really went more hardcore on machine learning and artificial intelligence in general. And apparently we did a good enough job because when Plansee Group bought the majority stake of CERATIZIT, so we were acquired, my team was actually offered the leadership of artificial intelligence and data science over full Plansee Group. So on a service function for all the holdings of Plansee Group. And here we’re still talking about high-performance material, not just in wear but in a lot of different areas.
And this also gave the good feedback. Millennials love feedback, so that’s important. But also the opportunity to grow this team, have a bit more resources and really work on building stronger and better systems to integrate with the operation of Plansee. We are talking about a company that is around doing a fantastic job for more than 100 years. So of course we are talking about a transformation there as well and a lot of wonderful system that could be built to integrate complex algorithms in the way they produce value for their customers.
Steven:
It’s great to hear that these old industries that have been around for a long time are some of the ones that are adopting this technology the quickest as well. So that must be a fun and exciting part of your job, mustn’t it?
Gabriele Pozzetti:
Yeah. I always say we have 100 years of experience in innovation that’s not about the latest technologies, about the mindset of, yeah, things change and they should change I would say.
So yeah, so that’s actually what led me there in my personal profile becoming from a very technical engineering profile and moving a little bit more towards leadership and making amazing people do really cool things together. That is also an extremely exciting part of my career, I would say.
Steven:
Before we move on, do you miss Navier-Stokes equations or not? Is there any days you want to do some more CFD?
Gabriele Pozzetti:
Every day. That’s why I actually am happy listening to a lot of your episodes of this podcast, because they re-echoes in my mind the problems as you see it as a CFD engineer, and oh, my God, this is a lovely relationship that once you tap into it will never completely leave you.
Steven:
I’m glad to hear it. So having lived both in a numerical simulation world and the AI world, what do those things have in common, and what are the differences between the new AI generation and old-school CFD engineers and numerical engineers like myself? What do numerical simulation and AI have in common?
Gabriele Pozzetti:
That’s a great question and I think one that I was asked many times and the way I normally approach this is to introduce two fictional characters. So the professor and the expert. The professor is for me the representation of numerical simulation in general, and the expert is for me the representation of how machine learning in particular works.
So, imagine that you have a problem to solve that requires some prediction. So your boss is coming into your office and say we design a ship, is it going to float or sink? We design a nuclear reactor, is it going to be cooled according to specification? We design this new generation of car, are they actually going to perform better than the old ones? So you need to predict something. And what your boss tells you, you can go to different consultant because I know making prediction is always difficult.
And so those two figures I think could help you understand the difference between numerical simulation and AI because, on the one side, you have numerical simulation, so the professor, that you know that when you bring in the problem, what he is going to try to do is open the books of physics and try to describe the problem you’re telling him with physics equations. With the idea that if you manage to do that, so if you manage to write a system of equation that represents your problem, then I can solve those equations and there you have your prediction. This solve the equation in particular is numerical because we know that mathematician only know …
Steven:
Solve a simplified version of the equations, quite often. It’s not always solving the real equations or all the equations because we can’t.
Gabriele Pozzetti:
Exactly. I was putting that the mathematician only knows how to know if there is a solution but not which one is the solution. But okay, you saved me from being mean with the mathematician. Oops, I think I’ve done it anyway.
And then on the other side you have the expert. So the expert is more what artificial intelligence approach will be, machine learning in particular that is, I don’t know much about the question, so I’ll not open a book and see what people wrote about it. I will just have a lot of experience in your field. So if you are talking about boats, I’ve seen a lot of boats in my life sink and float. If you talk about nuclear reactors, I’ve seen correctly cooled and non-correctly cooled nuclear reactors. If you talk about cars, I’m a car geek, I always go to races. And so instead of writing equation, he will just look at past experience and come up with an answer for the specific field.
So, both of them are definitely computational technique, they to try to predict something that can be used to predict something. The really challenging part is that both of them in theory could be used for any problem, but they have different condition at the boundary. On one side, numerical simulation is going to be as good is the system of equation you can write about your problem. And this means the equations on one side and this means the equation system is simple enough, because complexity will always make your job more complicated. So equation and material properties in the case.
And on the other side on machine learning part, your approach will be as good as your data. As good as your ability of having a dataset that describes your problem. And to do the next step here is about how cheap and convenient is to run experiments. So, if you’re in a case in which you can run a lot of experiments about your problem in a repeatable way and it’s cheap, then that’s a place where machine learning can really do great things. That’s why they’re so great initially for social media. Social media are huge structure made to run experiments. And on the other side as low quality your data is and as expensive it is for you to gather data, the more looking back at system of equation would have the higher hand. So that’s how I would explain the difference of those two computational techniques.
Steven:
Do you think that there is a risk to those of us who work in numerical simulation that the AI is going to replace what we do? That’s a big question. But looking at some of the claims that are being made by AI algorithm or AI companies, one company which I’ve seen, is saying that they can predict the weather in a year’s time. As a professor, equations guy that seems to me to be an extraordinary claim because there’s this whole thing called computational irreducibility that Steven Wolfram talks about, which basically says: we can’t take large leaps ahead, we can only take small steps for any realistic problem. For all but the simplest problems you have to go step by step and in CFD and in FEA, that’s iterations or time steps, and we can’t take these large leaps ahead. Do you think that AI has the potential to do that? Or do you think we’re still going to have to move forward in these slow increments or small increments?
Gabriele Pozzetti:
Very interesting question. So I would take it on two sides. On one side, indeed, remember what has already been discovered. When you have a system, your system is always associated with a certain amount of, let’s say, intrinsic computational costs. If you want to capture all the variable of the systems, you will have to make at least those computations and this is something you cannot run away by attaching a flag or a new board on it. I think that this should be always taught in both numerical simulation courses, but in general engineering courses, about realize that there are no magic wands. Because oftentimes both in conferences and in sales pitch from new AI companies, you get into the same environment that I have when I watch a very cheap thriller movie in which they’re taking the assassin by zooming indefinitely inside a picture and showing of the reflection of the reflection and they got it.
The information is clearly not there. These things is clearly an impossible. And sometimes AI in conjunction with numerical simulation is actually using this way, like, I can solve turbulence because I can make a generative AI model that create patterns that look like turbulent patterns that you are not going in the current way.
So, that’s I think absolutely agree is one thing that we should always repeat to our ourself, especially when technology gets very exciting, to understand that there are boundaries and not work too many hard on problems that do not make sense.
On the other side, let’s say in terms of the computational complexity and reducibility of a system, this as we said is something absolutely true. What I think simulation engineers should be good at understanding is that their role is not just to go at a system and find a computational technique to all the system. Their role is actually to choose the system, the model that they use to model reality, because even though a system that you want to solve, again, we can think about a turbulent flow if you want, that is a lot of information that you need to fix. I talk turbulent flow because I’m talking about weather forecast. There is intrinsic problem, there is turbulence. It cannot be solved entirely because of that one.
In certain cases you don’t need to have the full solution of the problem. You can look at a different scale, and this is actually the art of being a computational engineer, that is, don’t go brute force on it. Brute force approaches are normally a very bad idea both in numerical simulation and in AI to just throw in computational at a problem and hope this will improve. And if you think about weather forecast, as you said, brute force is both, doesn’t make sense on an AI sense, but also doesn’t make sense on a computational sense because if you look about the minimal length, and that’s something always stuck in my head, that you should be able to resolve planetary level. Should be able to resolve in order to exactly solve with a dark numerical simulation the problem of weather forecast of the moment, you’re looking at millimeters. So that’s a length scale you should be able to resolve.
And this means that not only you need a huge computational power, that’s clear, but you can say, okay, now we will find new leaps that will give you this computational power. But you also need to put initial condition to that port. So you need to have a map up to the millimeter of the entire world, and this is really what you should learn how to do. What is my problem and what’s the size of the model that is correct for this problem? And only then you choose the computational technique that you should actually deploy. That’s something that should really be engraved on our brain as the simulation people.
Steven:
That’s part of the skill of being an engineer, I guess, isn’t it? Is making those compromises, to turn intractable problems into ones that you can actually solve. Predicting the future is always difficult, and the fact that we managed to do it because we make lots of compromises along the way. One of the criticisms that you’ll have had as a numerical engineer or when you’re working in CFD is that CFD is a black box. But as you described in the beginning with your analogy between professor, CFD compared to AI isn’t really a black box, isn’t it? Because we can always go back and look at the equations and understand how they’re solved, or most of us can try to do that at least. Whereas with AI and machine learning, neural networks in particular, it’s sometimes difficult to see what’s going on underneath the hood. Do you think that’s a problem?
Gabriele Pozzetti:
Yeah, I think that there was a lot of work in the past. I mean, the easy answer to your question is yes, right? So yes, that’s the black box characteristic of AI is something that is well known to everybody that ever used it and is not as interpretable as a set of equation. But this concept of interpretability has been studied quite a lot in the machine learning community, and I think there are quite a lot of tools that can help in having not only a better understanding of the system that you’re putting in place, but also a better confidence of seeing how a system really works on the machine learning side.
I was actually thinking about this topic relative to the 2023 ‘topic of choice’ that is generative AI. So here we’re talking about AI at its biggest expression in terms of both capabilities but also system complexity. So you are looking at stack of transformers that look like they never end. It really embodies both the wow effect that AI knows how to give, incredible it can do this, but also the black box aspect that you were referring to. And these in particular gives, let’s say, to me, a really good point in which numerical engineers can see themselves closer to machine learning people.
Why that? Because if I think about it, look at how ChatGPT is being used. In great part, there are more and more products that have the same algorithm at the basis and they just have a little bit of prompting abilities or difference in the way you use those product, and you actually say, okay, now I’m reusing it. This is not that different, if you think about it from what you were referring as Navier-Stokes equation.
So, what is an equation from physics books? This is a collection done not by an algorithm but by the human race of a lot of experiences that we encoded in something that is an equation. And so, the way we are actually using equations start getting more and more similar to the way we are using these very complex models that we use as a pre-trained one, in which is you have a huge dataset that you somehow condense into a form that is easier to use. So, that’s a training process for ChatGPT, for example, and is the physics of humanity that looks at experiment to run experiment and then write equation down.
And then you will get this additional step of saying, okay, now how do I use this equation? It’s a general-purpose tool, Navier-Stokes equation or a large language model. Now, how do I embed it into actually getting a prescription?
I think yeah, there is definitely the aspect of black-box thinking for AI. I think it’s not as severe as many people think it is because this is often a good way to realize if somebody really is worth his salt in machine learning or not, if he’s just throwing data at an algorithm and hoping that something goes well, or if he knows how to handle the algorithm. So, I think there are a lot of ways to mitigate the black boxiness of your algorithm. But at a certain level I think we are getting to a point in which algorithms echoes our natural way of condensing information in different objects, that for us was equations before and now they are very complex pre-trained networks. Of course, I would not give away Navier-Stokes equation for anything in my life, so I’ll still took them over ChatGPT and ask them about fluid dynamics and not ChatGPT, but I think the cognitive process that we are observing starts having some resonance between the two.
Steven:
Yeah. No, I agree completely and I think that’s a good analogy. So you’ve made this transition in your career from being a simulation engineer into digitalization. What role do you think that engineers play in this new digitalized world? Do we still have a role? Is the first part of that question. And will that role increase?
Gabriele Pozzetti:
Absolutely. I’m not biased at all on giving this answer being an engineer, but absolutely engineers do have a role. No, and I think actually one aspect that especially simulation engineers, that if I understood correctly is a quite portion of your audience. often feel about is betrayed because they were the hot kid on the road 10, 20 years ago and now everybody’s always talking about AI and it’s, oh my god, everybody cannot shut up about just AI. Do you know how much work there is in the simulation? And so they feel a little bit like am I going to be replaced? But I think one of the things AI is doing is to pick up a lot of the small details, challenges like implementing a function in Python for example, and abstracting it away by giving you an agent that can do that relatively well.
And it’s making more and more important those roles that are more about design thinking, system thinking and code architecture, if we keep the programming level in mind. And this is, I think it’s something for which the specific knowledge of programming techniques or programming languages will become less and less important because if you have an idea, translating it to Python or Roster or Angular is going to get easier and easier. But at the same time, since your ability of generating small pieces of codes will be much higher, also the struggle of putting everything together will be much more complex.
So, this more architectural design thinking, that will be way more important. And I think engineers have a particularly interesting capacity in doing that one because what we do as engineers is really put things in boxes and label them. So that’s how our mindsets are, how we actually work. And this is really fundamental to approach a world that becomes more and more complex. You need to simplify by building boxes, and know how to build new boxes tomorrow because the old boxes don’t work anymore.
And so I think that the actual profile of an engineer was never as useful. So absolutely I would still recommend engineering as a career path. And it’s getting even more exciting right now because the potential that complexity gives you are more and more as long as you know how to end the complexity. That’s it.
Steven:
Yeah, I recently attended a careers fair where Siemens was recruiting. I was on the stand as well and I expected to be approached by lots and lots of engineers. But in fact it seemed to me that all the graduates and postgraduates, the vast majority of them were now data scientists as well. And I must admit, that made me feel a little bit uneasy but also reassured because as a simulation engineer, lots of the bread and butter parts of AI machine learning is providing a consistent clean set of data for the algorithms to train on as well. And we’ve been doing that forever, haven’t we? In terms of handling big data sets we were doing that before it was fashionable and trendy. So I think we’ve still got lots of the skill sets.
Your points about ChatGPT and LLMs enabling you to write Python and write code is, my experience completely is that for all of my career, I’m not a programmer. I like to do programming, I have to program, but it’s not my core skillset and it’s held me back in solving some of the problems I’d like to solve. And not anymore because now every single problem that I’ve ever wanted to solve, I can get ChatGPT to do most of the donkey work in writing the code much better than I could, ever, and then we can work together to solve the problem.
So, I think the truth of it is that there’s not enough engineers in the world. There’s so much engineering that still needs to be done, especially considering some of the problems that the whole of society are facing that can only be solved through lots of engineering. So, I think it’s just going to increase productivity and let us concentrate on the important bit of engineering, which is using data to make decisions. And yeah, I can only see it’s going to improve and enhance the role of engineers in society.
Gabriele Pozzetti:
Yeah, we do agree. Again, we are both engineers, so a little bit biased on the topic. It will be interesting to get somebody else to crush us on the topic and say, “No, you’re completely useless from now on.”
Steven:
So, your company has completely invested in this path, digitalization, but I guess lots of people listening to this podcast will be at an earlier stage of that journey or maybe haven’t started that journey at all. What tips can you give to people about this journey to digitalization, and how they can start out on about it? And how they can put together maybe a coherent strategy so they don’t take the wrong steps in the beginning and they move towards the final goal?
Gabriele Pozzetti:
Yeah, indeed. So a couple of thoughts about that. To start with, very easy advices that could be the grandmother advice. You didn’t need to have experience in artificial intelligence to know that, but it’s always good to remember it, is what will be fundamental and is fundamental is the importance of collaborations and partners. But even before that we say is a fundamental second step. But the first step is really understanding as a company the importance of understanding. So, not following these trends and just offloading to a partner and say, “We need a AI strategy. We need a digital strategy,” because there is nothing that is going to change the fundamental laws of, if you don’t understand something, it will not work well for you. This doesn’t matter how good your partner is.
And the problem I see in specifically the AI topic is that a lot of sales pitch are given as, “We all know that you have to do AI, we all know that you don’t want to bother about it, we just want to have it, so let us do that.” This collaboration will never work and it’s something that needs to be clear. It’s bad for both the company that gets the overall, let’s say, service and is also I believe bad for the company providing services.
So, let me give you a little picture of this one. So imagine that you say, “Okay, we have too much on our plate, we want AI, but we do not need to have any of us understanding AI. We just go with this external provider.” Now, I argue there are two possible scenarios out of this one. So, the first scenario that is the most likely is that you are just spending money in a project that you don’t understand, you don’t know what you want and you’re just in a floating mode, and therefore your project will fail. That’s the first outcome.
Now imagine a second outcome, that you find a partner that is so good that is completely revolutionizing everything that you do and everything that you want to do. It takes your data, reuse them to give instruction to your production, instruction to the people and to your clients, how to treat clients. Everything. All the budget, they get it covered because they are so good. And you are amazing as the C-suite that hired them, you are super happy. “Look, I managed to work it without hiring any people.” Yeah. How long you will think you will have until you will realize that you’re out of business? Because what this showed is that company knows how to do your job without knowing your industry better than you. So there is no really way out, no scenarios in which offloading the data problem to somebody else completely is going to be a solution.
On the other side, when you realize this, when you start crafting your own digital strategy, that’s what you need good people for and in particular people that can explain to you things in a way you understand it and not just say, throw out the buzzwords out of that one, then partners become really important. And the ability of differentiating the good partners for the bad one, that’s going to be really fundamental. But I really would advise companies not to jump to the second one before doing the first one. It’s the old Sun Tzu way. Strategy without tactics is the slowest path to victory. Tactics without strategy is noise before the defeat. It definitely applies here. And in this case, a good partner is a good tactic. If you have it together with a good strategy, you will do miracles. If you do not have a strategy in the first place, there is no way you are going to win that battle.
Steven:
And part of that strategy has got to take account of the fact, of course, that the whole field is changing incredibly quickly, isn’t it? I mean, I think that the LLM, ChatGPT, when I first started this podcast just over a year ago and we were talking to people about ChatGPT, it seemed like a toy at the time when I was using it to try and be provocative. But actually it’s just one element of AI, but it has changed everything and I think not many people saw that coming. And so we don’t know what the AI developments of next year or five years, 10 years. So we have to have a strategy that’s going to account. Is that difficult to do as well because the whole area is-
Gabriele Pozzetti:
If I can fire back one question before is, do you remember the first question you asked to ChatGPT?
Steven:
I would have used it like a search engine and asked it something probably about myself because my ego is so big. I might have asked it about the Engineering Innovation Podcast. But I think I looked at it, I typed it in and I thought, “Oh, that’s interesting,” and then put it down. And then I used it to write poems and limericks and funny stuff and I put it down again.
And then, I think I just talked to people at Siemens, I started listening to Lex Fridman’s podcast as well and realized that people were using it seriously. And then I saw people were starting it to use to write code and I thought that can’t be the case. That seems like magic. And I started writing some Python and it took away my whole problem, because I’m a before [inaudible 00:29:54] 77 guy, so the whole object-oriented world was a bit of a challenge for me. But suddenly I could write all the code I needed to write and I solved so many problems. So yeah, I went on a very quick journey and I now think it’s incredibly part of human society. I don’t use it every day, but it feels like I use it most days, I think now.
Gabriele Pozzetti:
Yeah, that’s an incredible … I think what ChatGPT just is, and it was when it got out, is really an incredible product because it’s a technology that has something new within it. It’s an expression of this power of the transformer architecture really embodied then in this technology. But it was also extremely well packaged, because it really gave you the idea of, look, algorithms could behave like a sparring partner, could behave like a person you want to brainstorm with. And I think that’s what this in particular ChatGPT is fantastic at doing is keep the conversation flow and give you some sort of a feedback like a human could do.
I think on the other side, as we often do in recent years, we got a little bit carried away with the potentialities of this one. And now everybody was hyping the subject a little bit more than it actually would be good for the technology. I think large language models are a beautiful invention that we just started exploring what they could do. But they often have been marketed in a way that actually makes them a disservice because now they ingrain in people’s brain expectations about this technology that are completely unrealistic, and now you see people try to sneak them everywhere doing things that they were not really meant to do in the first place.
And so I’m a little bit afraid. They definitely did very well the past year, so they can have a downwind here. But I’m afraid that we will wake up to the reality of they are an amazing tool but just a tool. And some of them were definitely marketed a little bit too much. And again, the possibility that they have is incredible. I think that their capacity of understanding patterns with their dimensions is not completely explored, and definitely language is only one of the fields in which we will see them.
On the other side to keep on the thread of our discussion, when I think about generative AI, I always think back as a safety engineer to turbulence modeling. That was another thing that when started pushing, everybody thought it’s just a matter of computational power and then everything will be solved. And you will also see these new prompt engineers figures that said, okay, the only thing you have to care about in a simulation is turbulence. That’s the only thing that exists. And I see a little bit of parallel with gen AI because in that case as well, you have something that is an incredibly valuable tool, so a genuine step forward.
On the other part of the equations, you have something that is extremely expensive and this is also what did it for turbulence modeling. Being so expensive and so fragile makes it that very good simulation engineers normally try to step away from complex turbulence modelings because they know that if I don’t really need to solve that aspects of the turbulence of my flow, it’s better if I get out because this could make all my system mad.
And these I think is something that generative AI shares, at least up to now with the transformer technology as its core as a feature. So it’s very expensive. We’re talking about I think hundred millions cost of training GPT-4. So that’s extremely expensive. Clearly you don’t want to do that many times. Even if you are a company that does that, you don’t want to do that many times. This is normally a recipe for not exploring too much. They’re fragile because you see that you can prompt those tool in various different way, but this is really the outer shell of what a large language model can do. The actual people that know how to train are very few, again, because it’s not something you normally do on your laptop. So could be very fragile.
And despite being a genuine discovery, I think we could learn as simulation engineers, what was for turbulence. In the last 20 year, turbulence model, we made incredible jumps. So, from we can now accurately simulate the coolant of liquid metals into nuclear reactors with a low front number. That’s incredible. You can do multi-phase turbulent flows and study optimization in a really effective way. Challenges that in the past were really incredibly mind blowing, but it never became mainstream as some people would have said. The whole simulation would be turbulent right now because it shared those characteristics.
So I don’t know. We’ll see there is definitely going to be a lot of money there, probably more than there was in turbulence. So maybe I will be proven wrong, but I would be a little bit cautious because when you get something that by intrinsic nature is so expensive and so fragile, normally it is difficult to get big leaps because then it gets a bit of chance. Because when you never know how to try, you don’t have the budget to try too many times, you get stuck into a wall relatively soon. So we’ll see, but I think we should be a little bit cautious with over-hyping them because maybe they are just a really good tool for something and we need different technology for something else.
Steven:
And other than LLMs, what other emerging AI technology would you say is going to change the world?
Gabriele Pozzetti:
Good questions. I think one interesting subject, that definitely Meta is exploring quite vocally, is the interaction between advanced machine learning technologies, AI system, and these three-dimensional special computation, let’s say virtual augmented realities. And that’s something that I think is a bit more on the far front before becoming a really mainstream product like ChatGPT actually is as a sparring partner, not as a numerical simulation, but as the conversational, let’s say, ‘buddy’ that is what ChatGPT is. Because I think it’s good to have a company or a set of companies that are so active in the two realms. Virtual reality on one side and artificial intelligence on the other one, because they probably are in the place to explore what this can do. But I still think it’s very young. I still think this is another part that simulation engineers like us could relate to because this shift to the metaverse that will also be industrial metaverse or private metaverse, it’s something that to me echos a lot the shift between 2D and 3D simulation.
When 3D simulation started, meaning we had enough computational power to actually get it done, that is what they’re doing with the headset in virtual reality right now. The first time you made a 3D simulation, you felt like in a different world. It felt real, “I have it in my eye, look at how cool, those poor peasants that still do 2D simulations,” but 2D simulations still didn’t disappear. Why? Again, for the reason of we are creatures used to live in a 2D-plus environment. And that’s why I think bringing us out of screens that are our 2D interaction with the digital world will definitely require quite of a profound transformation because just designing these things requires to really think in three dimension. Everybody thinks they can think in three dimension. I don’t 100% agree with that.
And if somebody can, definitely they’re engineers. But even as a user, this understanding how something would happen in three dimension, it’s a very interesting problem, very interesting to know that there are companies putting a lot of their money on it, so that we can arrive and observe the benefit of this shift. I’m pretty excited about that to see if these bring something, I think it could be for us shift to this 3D and this definitely brings a lot of excitement. But I think we all still have to understand its limitation.
Steven:
Yeah, I was on the cusp of that change. So I started working in 1993 doing CFD calculations with the first release or one of the first commercial releases of what was then called STAR-CD as well. And the generation before me, you’d go through the files and they’d been like 2D simulation of flow across on Ulrich and I would laugh at those things. But like you say, 2D simulations haven’t gone away because, as engineers, we are pragmatic and we have to solve difficult problems. And throwing massive cell counts and physics at problems that can be solved more simply isn’t a good idea, is it? And I think that’s part of your message with AI and machine learning is that you have to be pragmatic in the way you adopt it and you can’t do everything by just throwing lots and lots of CPUs and calls at things.
Gabriele Pozzetti:
We naturally fell in love, especially as engineers, with the cool tech stuff, but we should also have some of our own emotional regulation about it and understand as they are right now, primarily tools. And even if you think about them as complex systems, they’re still system that will have their shortcomings. And in a world of incredible technological abundance like the one we live, I think is important to strike a little bit of a balance between this ability of doing things and generating part and ability of filtering it out.
So that’s something else that I think we have to keep learning the more we advance this type of technologies, because otherwise we really risk to be in this hell of abundance in which there is just too much fluff going around. Everybody sends 2,000 emails every day because ChatGPT can write them for you and everybody is caught into these ping pong between machines and we just lose the meaning of everything that we are doing. And I think that’s a really important skill that we have to keep cultivating, for sure.
Steven:
So that’s a brilliant place to end this interview, I think. I love your perspective. I love the fact that you’ve completely fallen in love with all this technology and all the technology you used before, but you keep this pragmatic and honest view of these things and their limitations as well. So I think that’s a really helpful place for people to start. So thank you very much, Gabriele, for your contributions and thank you for listening to the Engineer Innovation Podcast.
Gabriele Pozzetti:
Thank you, Steven. Bye.
Speaker 4:
This episode of the Engineer Innovation Podcast is powered by Simcenter. Turn product complexity into a competitive advantage with Simcenter solutions that empower your engineering teams to push the boundaries, solve the toughest problems, and bring innovations to market faster.
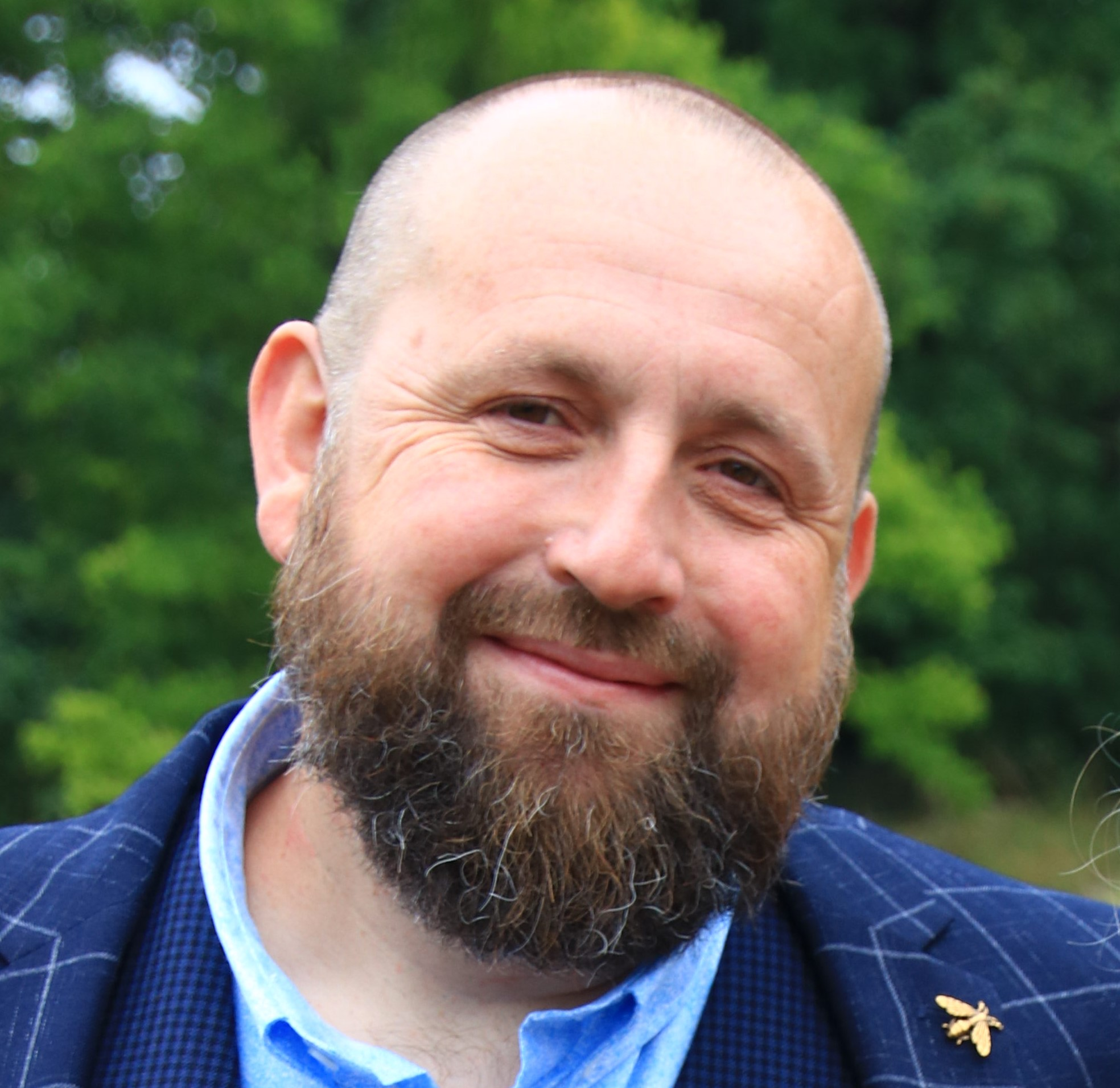
Stephen Ferguson – Host
Stephen Ferguson is a fluid-dynamicist with more than 30 years of experience in applying advanced simulation to the most challenging problems that engineering has to offer for companies such as WS Atkins, BMW and CD-adapco and Siemens.
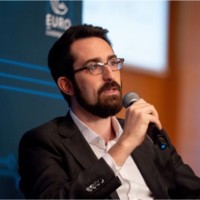
Gabriele Pozzetti
Gabriele Pozzetti is a manager of AI and Data Science with a Ph.D. in computational engineering and experience in vertical and lateral leadership of international development teams. He is passionate about human-centered digital solutions and believe they are the key to a more sustainable and even more exciting future.
Take a listen to a previous episode of the Engineer Innovation Podcast: Engineer Innovation: Energica’s Electric Vision with Giampiero Testoni on Apple Podcasts
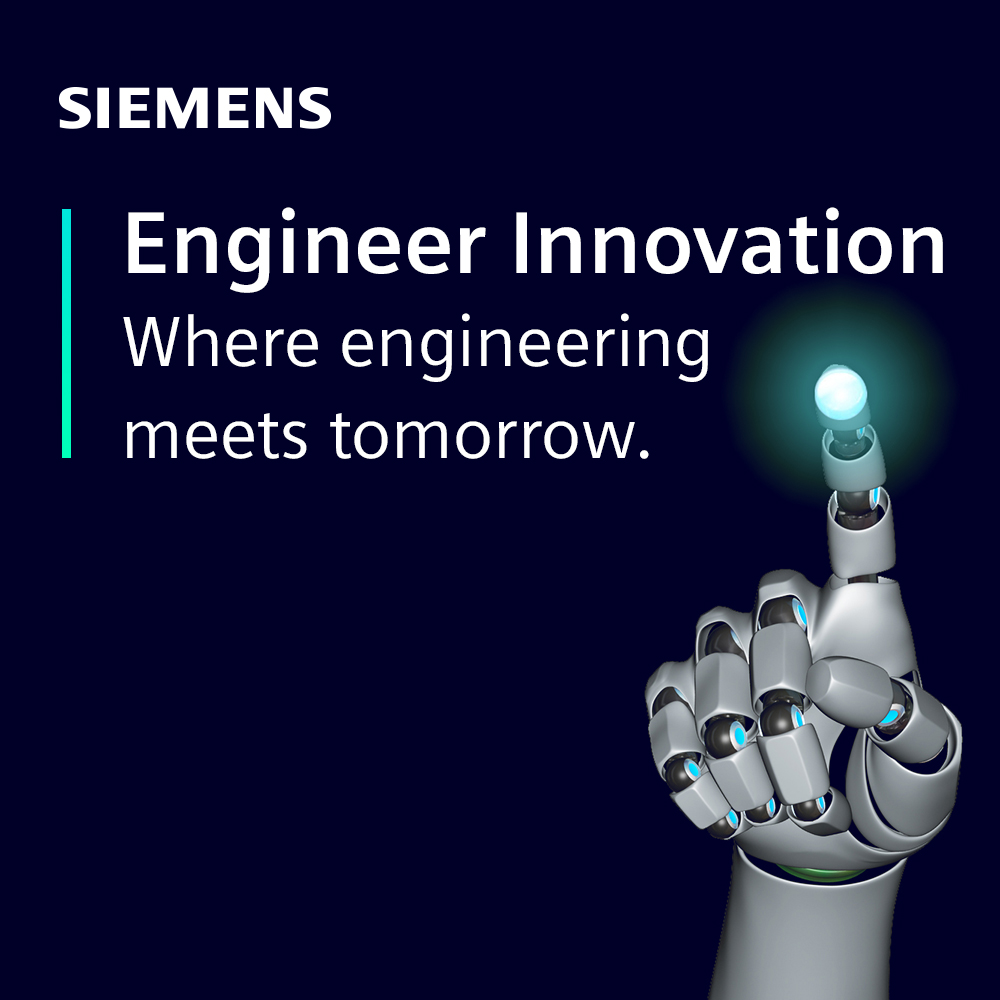
Engineer Innovation Podcast
A podcast series for engineers by engineers, Engineer Innovation focuses on how simulation and testing can help you drive innovation into your products and deliver the products of tomorrow, today.