The truth about AI in engineering with Jousef Murad (Season 2 Bonus Episode)
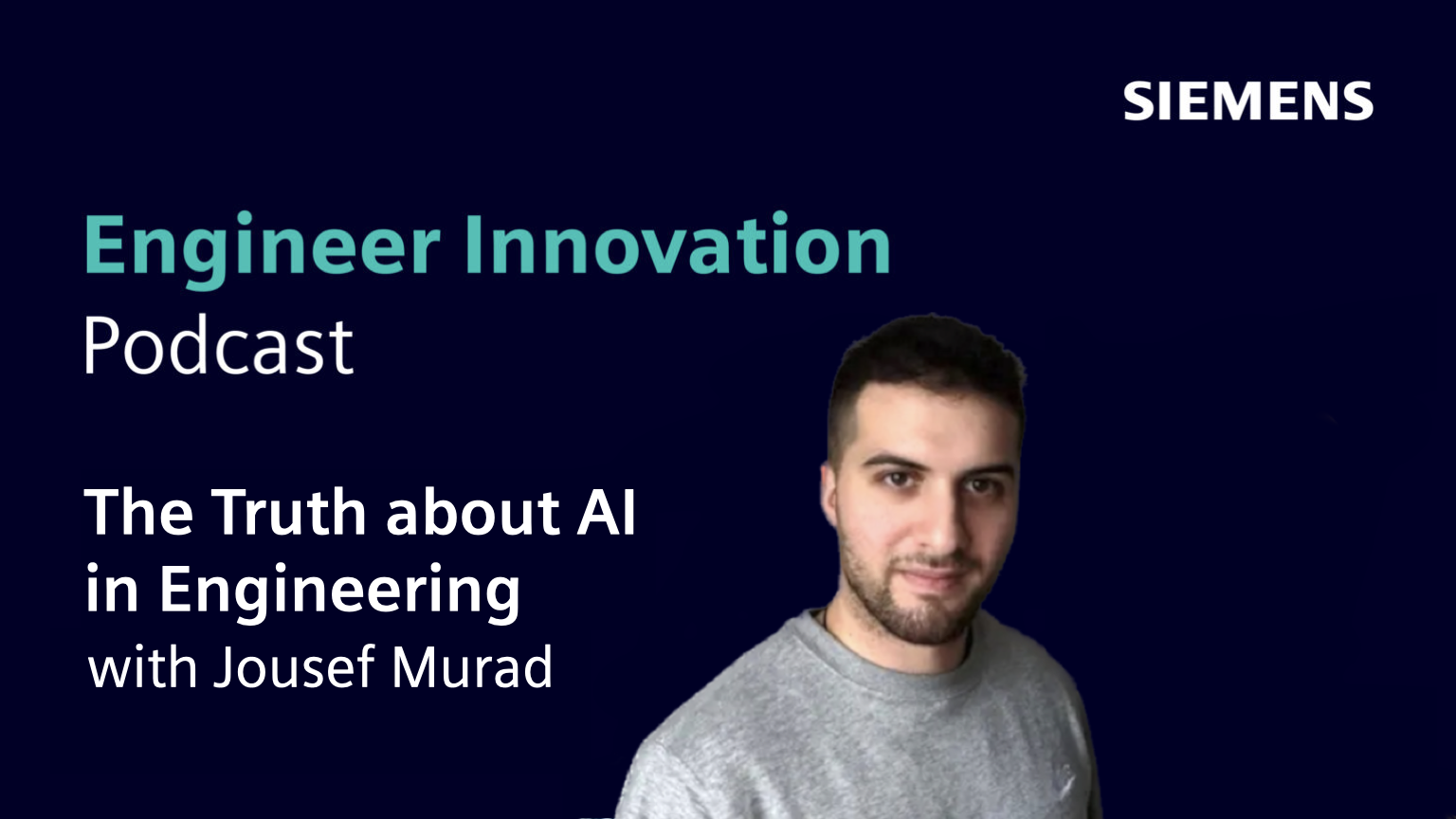
In a special Season 2 Bonus Episode we take a brutally honest look into Artificial Intelligence and Machine Learning to try and dispel some of the hype that has built up around topic, and to see if we can distinguish what is possible now, and what might be possible in the future of AI. My guest is social media influencer, engineer, and engineering AI expert Jousef Murad from the Engineered Mind Podcast and YouTube channel.
-
How AI will change the profile of engineers in the future
-
The role that AI will play in the future of engineering
-
Extracting more value from simulation and test
-
Using AI to reduce mundane and repetitive tasks in engineering
-
Formalising the transfer of accumulated experience between projects using ML=
-
The potential for AI to generate full 3D datasets from existing simulations
-
ML as a tool for eliminating bias in engineering
-
How AI is not a “magic pill”
-
How to get started in AI and ML today
Stephen (00:11):
With me today is Jousef Murad, who describes himself as an engineer, a YouTuber, and a podcaster. And he’s also the author of the Sunday Science Newsletter, which arrives in your inbox every Sunday morning, with all the exciting news about science and engineering. I’d describe Jousef as one of the biggest influencers in the simulation game. Jousef is the host of The Engineered Mind podcast and YouTube channel, which has over 20,000 subscribers now, I think, and is fast approaching the magical hundred podcast episodes. And in this podcast, I wanted to talk to Jousef about how artificial intelligence and machine learning will affect the future of engineering.
Good morning, Jousef. Could you start off by telling us a bit about yourself and your background as an engineer and as a social media influencer?
Jousef Murad (00:58):
Definitely. Thank you so much for the kind introduction, Stephen. Happy to be on the show, by the way. It always feels a bit different to be on the other side of the seat, so to speak, being the interviewed one. So my name is Jousef, as you already mentioned in the introduction. I’m a mechanical engineer by training. So in my bachelor’s, I focused on structural mechanics, so FEA, so Finite Element Analysis, switched over to CFD, Computational Fluid Dynamics and turbulent modeling in my master’s, and then finished off my master’s thesis using AI on 3D data, which we might even talk about later in this podcast. So yeah, I do a bunch of stuff. I work for a company in London called Monolith AI, so they do AI for engineers. I also host a podcast called The Engineered Mind, where I also was very lucky to interview a lot of AI and simulation enthusiasts and experts. So yeah, I’m really looking forward to this episode today, Stephen.
Stephen (01:48):
So when did you graduate from University, Jousef?
Jousef Murad (01:51):
It was around three-ish years ago.
Stephen (01:55):
So one of my observations is, I graduated from university almost 30 years ago. Yeah, I’m nearly 50. I am 50 now. It’s almost 30 years in engineering. And listening to that description of you, you sound like a generalist. You’ve done some FEA, you’ve done some CFD, and I think that’s kind of the profile of modern engineers, isn’t it? People like me who are just single, niched CFD guys or FEI guys are perhaps dinosaurs who are going to die out in the future. Do you think that’s the future of engineering, is generalists like yourself?
Jousef Murad (02:25):
Oh, that’s a very good question. Usually what we refer this to is the Aisha personality type or a Tisha personality type. So either you go very deep into one topic, which you have done, which I think is good because we need people like you who are experts in that field, but the Tisha personality, I think, will be something which will definitely be more common, so to speak, in the future. So engineers should be able to know a little bit about FEA, CFD, CAD modeling, AI for sure, because I think AI will play a huge role in the product development process of engineers in the future.
Stephen (02:58):
Yeah, so I agree completely. My view is that most of the simple problems in engineering have already been solved, problems that just involve fluid mechanics or just involve structural mechanics. And actually, more and more, it’s becoming a multi-domain problem where engineers have to cross all those domains. So I’m reassured that people like you are coming out of university to replace people like me. So today we’re going to talk about artificial intelligence and machine learning, and we’re going to talk about how it’s going to affect the future of engineering simulation. So I’m going to start with a big question. If we step forward in time, say a decade into the future, what sort of role do you see AI and ML playing in the world of engineering?
Jousef Murad (03:38):
Oh, I love that question, Stephen. Maybe let’s think about the product development process in general because usually when we talk about the simulation space, we talk about things such as front loading and computational fluid dynamics, HPC and enhancing engineering abilities to actually solve problems faster using simulation. Where I see AI in this whole ecosystem, if we want to call it an ecosystem, is that AI is not here to replace engineers. So we’d rather think about actually enhancing or augmenting engineering abilities because AI, first of all, is very dumb. So if you don’t give it enough data, AI will be super stupid. So we don’t have artificial intelligence, we have artificial stupidity, if you want to call it that. So first of all, we need high quality data. Who’s gathering that data? Most of the time, engineers. So the engineers will be the responsible ones for artificial intelligence to be able to properly train and then make accurate predictions.
So where I see AI sitting in this ecosystem is kind of as a third hand, if you want, or an extension of human capabilities to achieve more. Because a lot of people think when AI comes, it’s kind of this Terminator scenario and whatnot if you watched that movie.
Stephen (04:44):
Yes.
Jousef Murad (04:44):
It’s like, they’re going to substitute us, they’re going to destroy us and whatnot. I think we are far away from that. What I love about AI is, it’s going to augment or enhance engineering workflows or engineers in general, in their product development processes.
Stephen (04:57):
So we talked about data and I think as engineers in the simulation game, we leave a huge trail of data behind us, don’t we? I mean, going back to the 1990s, I’ve got tapes which have got engineering simulations and most of that data is kind of waste, isn’t it? We draw a few conclusions with it and then we pack it away on a tape or more likely archive it on the cloud or sometimes delete the result set so you can simulate it again later. Do you think that AI and ML are going to give us more use for that old data and maybe give us an opportunity to re-extract some more engineering value from that data?
Jousef Murad (05:31):
Absolutely. Yeah, what we’d like to talk about in Monolith is basically four engineering challenges, main challenges. One, we have the upscaling problem, which is that engineers generally, they’re not data scientists, but they’re subject matter experts. For example, you Stephen, you’re an expert in CFD.
Stephen (05:45):
Yeah.
Jousef Murad (05:45):
So you kind of know where your data is laying around, how the data is produced, which format and so on and so forth. And then you want to use your knowledge, in that case, the knowledge of the engineer, to actually gather the data, put it into a data lake optimally or somewhere where it’s being stored and actually make sense of that data, because the data scientist is not an engineer and an engineer is not a data scientist. So this is the thing which we have to figure out. So how do we make engineers more capable using artificial intelligence?
Then, what we have is also traditional R and D methods, which we talked about this classical physics based approach, which is CFD, FEA and so on, maybe SPA and so on and so forth, versus the data-driven approach, namely artificial intelligence. So how do you switch from using a physics based approach, which is sometimes very time and cost intensive, to a more data-driven approach? Which brings us to the next point, which is underutilized engineering data. So maybe an anecdote. I was doing my internship building a V8 engine in Korea, and I was building all these parts and at the end, I had this beautiful V8 engine and animating and whatnot, but then I found out there was some data already laying around from some other guy that was doing this internship. And I was, oh, really though I had to do everything from scratch.
And I think this is actually one of the biggest problems. Data governance. Where is the data, is the data in a good format? Is it high quality data? And actually as you said, we have centers everywhere. We are collecting data already. So why not tap into that data and make sense out of that data and maybe extracting the physics that we have collected from the real world rather than going into a physics based approach where we make highly complex assumptions how it goes. We have models and the engine is sitting in front of the computer, which is producing most of the errors. So how do we take sensor data from the real world and actually extract knowledge from that data? And then last but not least, the fourth engineering challenge, which is intractable physics, what we call that Monolith, which is nothing else than complicated physics.
So how do we actually extract physics from highly nonlinear phenomena such as crash testing for example, or if you have a sloshing simulation. You know that for example, CFD does not do a very good job of actually predicting sloshing and actually sloshing noise because of its fidelity. So this is a project we’re working with Caltech at the moment where it’s like, how can you predict sloshing noise in a tank using artificial intelligence? So this is I think very, very exciting. And from my personal experience, because I mentioned earlier on, I was doing my master thesis in AI for 3D data. If a CAD designer for example, uses AI, how cool would it be if we create a part, maybe we do a revolution or like you create a cylinder and then the AI would tell you some kind of a recommender system, hey, by the way, I think you want to build or create a shaft.
And then the AI recommender would tell you, do you want to have this part, this part or this part? And then the CAD design would basically just click on it and then it would grab that file from the database and actually make it usable for the engineer or the design.
Stephen (08:37):
So that’s an example of how AI is going to improve the engineering process as well. So for example, if somebody’s working on an underhood simulation of an automobile, you know, get thousands of CAD parts in those assemblies, and the first big job for an engineer is sorting out all those CAD parts into different groups. So you can apply boundary conditions, you can delete the nuts and bolts that you don’t need. And I think AI is a good method already, or it’s starting to become a good method of identifying different parts and taking all that kind of heavy lifting work out of engineering simulation.
Jousef Murad (09:09):
Absolutely. But at the end of the day, I think it still needs the engineer to actually do this kind of sanity checking. Sometimes AI can be used for anomaly detection, sanity checking and whatnot. But I think still at the end of the day, the engineer has this intuition, this gut feeling, that you cannot take away from us. So we still need our expertise and our intelligence, but I think in combination in synergy with AI, I think we will become a very strong force in the future.
Stephen (09:34):
And that’s just a truism about engineering simulation in general, isn’t it? I mean, because I think we all run models. Predicting the future is a difficult thing and you have to do it with a great degree of skepticism. Anybody who doesn’t look at every single result set and look for potential errors and challenge their assumptions. I always say when you’re performing a simulation, before you do a simulation, you should do the simulation in your mind first and kind of predict the outcome so that when you see the fancy colorful graphics, you aren’t confused by things which are just artifacts of the simulation or just plain mistakes. I’ve seen lots of people confused by that over the years, including myself.
I’ve made a few mistakes myself. So I think that kind of skepticism and engineers working together with AI is really important. You just talked about when you were doing your MSC thesis and not realizing that somebody else had done some of the work before. Isn’t that another factor in engineering is that most of us spend our whole careers accumulating knowledge and expertise and then we move to different projects, different jobs, and that expertise moves with us. Do you think AI has the potential to formalize that data collection, so it becomes independent of people and the models have some of that learning and you can pass that kind of learned experience from project to project? Is that one of the applications?
Jousef Murad (10:57):
Love it. Yeah, absolutely. So what we usually distinguish is implicit data and explicit data. So explicit data would be something like CAD files, spreadsheet, whatever. It lays out somewhere in a specific format. And then we have implicit data, which is the knowledge in the engineer that they take with them. So for example, if an engineer retires, the knowledge goes away from that company if it’s not being stored somewhere. And I think AI is the perfect opportunity to actually take that knowledge from the engineers that they have produced in the past or maybe right now. Extract that knowledge and actually keep it for generations of engineers to come. So if a new engineer comes with that AI model, the AI model would immediately tell us engineers, this is the data that we’ve used. This is something we extracted from that data, and here you go. Instead of asking a retired engineer, by the way, what have you done 10, five years ago? That would be a bit of a struggle.
Stephen (11:47):
Yeah, because most of us can’t remember what we did yesterday, let alone 10 or five years ago. Again, I’m biased because I’m a CFD engineer and I kind of think of the world in a CFD context. Let me give you a quote from one of my customers who I talked to recently who said, “We spend most of our time performing complicated engineering simulations that generate terabytes of data when all we are really interested in is one or two numbers that pop out at the end.” Now I think that’s a bit of an extreme example, but it’s basically true. It is that we perform all these simulations and we take a few small pieces of information from them.
I’d say as an engineer, I’d always look through the flow field and look through the pressure field and you’d be looking for certain flow features, but there’s also a huge amount of bias in what I recognize as an important flow feature. It’s just limited by my vision and by my experience in the past. Do you think that by scanning these data sets, AI and ML algorithms, we’ll be able to spot things that old engineers like me don’t see with our eyes that influence the performance of a product that they’re simulating?
Jousef Murad (12:58):
Probably, I’m not a fortune-teller Steven, unfortunately, so I can’t actually tell what’s coming in five to 10 years, but I think we are moving in that direction. So basically getting instantaneous insights of whatever, like specific full three-dimensional CFD fields for example. And I think we are moving towards that direction. So actually making use of CFD data and getting instantaneous insights and improved design exploration and what you already mentioned, the transfer of knowledge of that data that we are creating, and for CFD simulations. Not only CFD simulations, but simulations in general. So my wish is actually for the future that we get there, but I think the next five to 10 years will show how AI will actually transform engineering workflows.
Stephen (13:39):
And that quote that actually we generate lots of data, but in the end we only really consume small parts of it. We generate a few plots and a few numbers or some performance curves. That’s also good news, isn’t it? Because actually what we need from artificial intelligence and machine learning is not to generate the full dataset. We need it to generate those pictures, those numbers we don’t have to have… We’re not expecting it to generate a full 3D distribution of all the data are we?
Jousef Murad (14:09):
It really depends on the use case I think. I think it really depends on the use case, but I believe that I will definitely help us to make that step, but actually make faster predictions in the future. I’m not sure for a CFD engineer what it will bring in the future. I really have no idea. But my wish is actually that the simulations will actually be performed faster.
Stephen (14:30):
So one example that we’ve seen here at Siemens with Simcenter is our engineering services team looked at some vehicle cabin simulations, HVAC simulations, so basically modeling the thermal comfort of the inside of a car. And actually the single piece of information which was most useful from that simulation was a three-dimensional plot or 2D plot view of the temperatures projected onto the interior of the cabin and all the passengers. And what they managed to do is by running… I think they did run 1500 simulations and just from those simulations exported a single plot, which just showed temperature contours on the passengers in the vehicle, and then they trained a machine learning algorithm. And from that machine learner… From those 1500 scenarios, then now they can almost in real time simulate any other number of scenarios. So that’s one of the potential applications too, isn’t it? Because we are used to sitting in a design meeting on Monday morning looking at our results thinking, oh, we need to change that, and then spending the next week generating data before the next design meeting.
So if we could sit in those meetings having trained our algorithms with lots of the data we’ve done in the past, we could start to get some indications about the performance of future designs without having to run the whole simulation.
Jousef Murad (15:48):
Yeah, I think that’s super fascinating. But then on the other hand, that would be the more of a skeptical person and ask, have you thought about edge cases for example? Or how do engineers perceive AI being used in their workflow at the moment? Right now, it feels like I remember back in the days when you went from a drawing, a mechanical design on paper, we just had pen and paper and then we went from CAD, which also took some time to actually be adopted in the engineering workflow. And now I feel AI is not the same, but something similar. So how do you see personally, maybe in the engineering workflow, do engineers welcome AI? Are they afraid of that or do they just blindly trust AI, some of them at least and say, okay, well we just do a click and then we have the results?
Stephen (16:28):
So I’d hope that most people who are involved in engine simulation don’t blankly trust anything because that’s one of the, like I said before, it’s one of the worst mistakes you can make as a CFD engineer or FE engineer is trusting your data too much. So I hope they’re skeptical about everything. I think it could be used to guide the next decision. So I wouldn’t want anybody using the AI prediction of the simulation results as the final answer because it should be just a way of maybe down selecting the next simulations you’re going to do. But I mean, I think at the moment it’s still early days. I think people like Monolith are helping to package up AI into a usable kind of work stream. The big question is people like me, and I guess you who are engineers, do we have to become data scientists to really make AI at ML useful.
Jousef Murad (17:20):
I don’t think so. So again, I speak from a Monolith experience. It’s actually the movement towards No-Code tools. So for example, or a low-code environment. So if you want, you could inject code if you’re like a geeky engineer who loves to program, maybe in Python or any other language. So we’re making that step towards making AI more accessible for engineers. So what we are doing is not disrupting the workflow by saying, by the way, here are 15 packages, please use them. But rather saying, you have a no-code environment, you import your data, you can build a whole pipeline with it, build models and directly get insights to that data. And then you have all the other USPs such as you can share the results collaboratively in an environment that’s everything sourced on the cloud. You make use of the HPC capabilities and so on and so forth.
So I think not only monolith, but a lot of companies in the simulation space actually take that step and move more towards a no-code environment. But then on the other hand, a skeptic might say, well, if everything is no code, it’s kind of a black box. So it’s a bit of a balance to actually make sure that an engineer still has the ability to tap into that data and actually actually gets insight into the models and what’s happening behind the scenes maybe in the numerics, but also not waste too much time on actually numerically tweaking settings and model parameters and all these things.
Stephen (18:37):
But for an old guy like me that argument is as old as CFD. And so I remember when I first started doing CFD, it was a very manual process. There weren’t any GUIs and we used text interfaces and they used to have to type everything into them online, which was surprisingly efficient. So don’t knock it because we used macros more than button clicks. And I think in some ways we were further forward than some people today, but in those days there was, as CFD started to advance and become more and more usable, when we started to have GUIs and window clicks, the criticism was always that CFDs becoming too easy and the wrong people will start to use it. Because in my generation of CFD engineers, I don’t have a PhD, but almost everybody who started with me to… In order to even start a career in CFD in 1993, the expectation was that you had a PhD.
And so most of my contemporaries had PhDs and even engineers like me were kind of looked down on because we didn’t have enough expertise to run CFD. And of course all that was completely wrong because CFD has become much easier to use. Yeah, it’s become much more accessible. And I just think the quality of engineers is enough that they understand it’s only a tool and they learn how to use it and learn how to use it much better and in much more different ways than I did as well. So I think that fear about are the wrong people are going to use it? I don’t think that’s really played out in my career. I’ve seen a fair amount of bad cfd, but I’ve seen much more good CFD and good simulation, so I’m not too concerned about that. What is the current…
After you?
Jousef Murad (20:11):
I would do it, definitely agree, Stephen. So I don’t think we need a PhD nowadays to actually tap into AI, CFD and whatnot. I think it’s actually a beautiful thing that it’s being democratized, whether it’s AI or simulation and it enables or opens new areas for other engineers to actually use these tools and not do a PhD first and then being able to use these tools. So I think it’s a good thing, not a bad thing.
Stephen (20:32):
An issue with real life engineers is that sometimes we are biased with the sum of all our experiences, and so we apply our bias to everything we do. Is there any way that AI and machine learning can kind of remove that bias to help us make more objective decisions, do you think?
Jousef Murad (20:50):
Definitely. I’m an expert in bias and whatnot, but definitely there are some ways of actually removing bias. Which I think from my intuition, I would say it’s a bit tricky because there will always be bias in data. So how can you actually tackle that? Maybe you have some kind of committees, maybe regulations that you follow when starting an AI project. So I think the most critical step for an AI project would probably be to train the model and then after deploying it, maintain it and actually see what the results will be, whether that’s in edge cases or in special cases to make sure that these biases are removed as good as possible. But from my perception, I might also be wrong here, and there will always be bias in the data, but we have to make sure as humans to actually see how the model performs in the real world.
Stephen (21:34):
That’s a good segue into the world of physical test data as well. So we’ve talked a lot about how engineering simulation can be used to feed AI and ML algorithms, but yeah… Engineering simulation data is full of errors, isn’t it? All of it is. And all models are wrong. Some models are useful, and so we get used to correct mentally tuning those things out. You’ve mentioned before the possibility of using AI and ML using real life test data or sensor data. Can you tell us a bit more about those possibilities and how you think that plays into the picture?
Jousef Murad (22:06):
Definitely. I can probably talk the whole day about this because we have some good experience with customers at Monolith. So what the basic principle looks like is as follows, you basically tap into your test data and what you then do is actually apply AI models on that test data and then extract knowledge from it. You can now say, well, it kind of gets the physics quote unquote, but it’s a whole nother topic on physics informing your networks and whatnot. But actually what you do is you take that sensor data from the real world, the measurements that you have taken from the real world or your test then and actually try to make sense out of that data using all the things that you have collected, whether that’s sensor data, maybe crash test data, maybe specific other quantity that you’re interested in. For example, in a crash test could be something like a tibia index.
So what is the force on your tibia? So with all that data that you’ve collected over the years and BMW for example, one of our customers is like they have a huge data lake. So we thought about, okay, let’s tap into that, let’s work on a project. And actually what we did is, and we were able to predict the outcome of a tibia index. So basically see how big the force on the tibia is by actually changing input parameters and saying, for example, if the car has this velocity crushes at this angle, how big would the tibia force be? And surprisingly… And I was very surprised as well as it’s a non-simple phenomenon, and the AI was able to actually predict the force very accurately, which is I think really, really cool. But it doesn’t stop at crash testing. For example, if we think about wind tunnel testing, so if you’re an engineer, test engineer, aerodynamics engineer, you go into the wind tunnel, as we all know, wind tunnels are extremely expensive.
So that cost tens of thousands, maybe hundreds of thousands of Euros. So how cool would it be if an AI gives you a prediction of, for example, you have the wind speed and other input parameters, and then you would be able to predict, for example, the drag value on a rim design. So you don’t only work with test data in a tabular format, but you would also be able to use 3D information, which is like the rim design. And then the AI model would actually give you a new design, but also be able to predict the drag value on that rim design.
So actually the engineer would see, okay, this would be the drag value. So I’m very lucky that I didn’t have to go to the wind tunnel, remove the old rim, put back the new rim, and actually see what the drag value would be. But I had actually saved myself time, money, and hassled to actually go to the wind tunnel and actually predicted what the outcome would be. So I think even when I saw that the first time, I was like, nah, that can’t be very skeptical. But actually it worked out in the end, which is really crazy.
Stephen (24:31):
Yeah, and it’s incredible. And the cost savings from that would pay for any R and D cost into AI very, very quickly. I think that the return on investment would be huge. How good are AI algorithms for dealing with that kind of 3D data? We see a lot of 2D and tabulated data examples like image recognition.
Jousef Murad (24:49):
Yeah.
Stephen (24:50):
How robust are they dealing with three-dimensional data at the moment?
Jousef Murad (24:53):
Yeah, I can’t tell so much about it because it’s a patent pending algorithm. So it’s a proprietary algorithm of Monolith. So first of all, they are very data hungry. So what I can tell is that you need a lot of data for that, whatever a lot means here in that case, but they’re very performance. So training takes a lot of time. But fortunately, as I mentioned earlier on, we have the HPC capabilities, so everything’s running on AWS. You would be able to actually train the machine learning model rather quickly, quote unquote, to make, to be able to make those predictions based on 3D data. But as you said, 3D is very complicated.
2D was already back in the days five, 10 years ago, was very complicated with all the convolutions and whatnot doing convolutional neural networks. But we’ve made a huge step actually overcoming that what they call AI winter, and actually the next step is now going to 3D and then actually maybe the next step would even be 3D, but a whole car and actually predicting, for example, CFD parameters or CFD values, quantities of a whole car by changing input parameters such as the form of the car, make it more SUV, maybe more like vocabulary.
So that would be the next step. And actually explaining the predictions, which is then even worse or even harder because you have 3D, which is hard. And then you have the explainability part, which is hard because no doctor, for example, would trust the CNN prediction of like, yeah, this is maybe a cancer cell or whatnot. So why would an engineer trust the prediction? So we have to also make sure that these algorithms are explainable.
Stephen (26:20):
Yeah, I mean think that was a problem with the early days of optimization as well, if you’d go through a complex optimization problem to improve your design and it would come up with something silly and unfeasible as well. So the explainability I think is a really key part of that. So we’ve talked again about a lot about how data hungry these algorithms are, and we’ve talked about engineering simulation and test, but of course things like digital twin now give us the opportunity to collect real life data in real time and almost not only validate our simulations and our test data, but improve it as well. So do you think that kind of building machine learning into digital twins and digital twin data is going to be another application?
Jousef Murad (27:04):
No, I think so too. So whatever you ask me, Steven, I will always be the optimist.
Stephen (27:08):
Yes.
Jousef Murad (27:09):
And say, yeah, yes, we’ll do it in the future if we actually do it in the future. I have no idea. But my wish actually for engineering applications would definitely be that you kind of have a mixed reality or mixed role of actually a digital twin and machine learning applications. How that might look, I have no idea, but I think the future definitely looks bright for engineering applications using AI, virtual reality, mixed reality, and all these kinds of things. So I think the future engineers will not be bought for sure.
Stephen (27:35):
And I think what you’re describing is that we’ve always used test data to validate simulations, but actually AI might be a way of combining learning from simulation, learning from test, learning from real life experience. And it’s almost like the holy grail, isn’t it? It’s like real time validation. We constantly improving all of our predictions based on real life experiences. There’s a book called The Signal and the Noise, which is my favorite book about predictions. And the people who make the best predictions are actually weather forecasters because not economists, not people who or volcanologist who are trying to predict when earthquakes and volcanoes are going to happen, but weather forecasters because every single day you can look out the window and see if the prediction you made the day before was correct with economists, things that get predicted in 10 years time. So you always have to be checking your simulation predictions against reality. And I think that AI and ML are an opportunity to do that in real time and always be kind of improving all of our predictions by verifying them against real world and test data.
Jousef Murad (28:35):
Absolutely, Stephen. Now I might add actually, if people are listening to this podcast, of course I’m biased here. I work for Monolith. I love what AI can do for engineers, but you have to also say that AI is on the magic pill. AI can do a lot of great stuff, but it’s not the solution to everything. For some people or some companies, CFD or traditional R and D approaches such as CFD and whatnot, they might be useful enough to do the job. Sometimes you don’t even need AI. So you really have to actually think. First of all, think about the data. So do you have data available? If so, how much data is available? Think about the company pain points, what do you actually want to solve with AI? But then also think about AI capabilities. So we see companies such as DeepMind and OpenAI.
They do all these kinds of great things, but the thing is they take a brute force approach most of the time. They have huge, huge HPC systems. They take millions of cost to actually train that model. Which brings us to the point of sustainability. Is that sustainable? Probably not. So we have to make sure that the AI models that we train are sustainable and also make sense in a specific use case and solve a specific business problem. So if you think about AI, don’t think it’s a magic pill. It can solve a lot of problems, a lot of complicated problems, but it can definitely not solve everything for an engineer. So that’s something engineers really have to think about.
Stephen (29:53):
And it’s only really valuable if it improves the amount of quality data we’re getting from simulation, isn’t it and, or reduces the cost.
Jousef Murad (30:01):
So correct.
Stephen (30:01):
I think for me, the real benefit will be, it’s going to less let us extract more data or more useful engineering data from the simulations we already produce. I think it’s going to increase the productivity of engineers by eliminating some of the repetitive work. And I think, like we said before, it’s going to help us transfer knowledge we acquire on simulations or projects. From project to project or job to job as well, which I think all those things are valuable, but it has to pay for itself, doesn’t it? If it costs more, then it delivers, then people are not going to adopt it and they’re not going to invest in it. And I think these are early days and hopefully that cost will decrease, but at the moment, yeah, it still has to prove its worth in lots of examples, but I’m sure it will.
Jousef Murad (30:44):
What I would always recommend people to do, maybe start with a POC, a small demo, take some of your data, work with a company with a small list or another company, try your POC and see what it can do. Maybe the results will be awesome, maybe the results will be complete garbage, but I think it’s a first way to actually see what I can do for your company. So instead of being hesitant, give it a spin and see what it can do for you.
Stephen (31:08):
Yeah, so my view on that as well is, so every… Used to be every year I intend the life conference, which is a seamless conference, which is kind of where we talk to all our engineers and they do presentations about the cutting edge stuff they’re doing. And last year in Berlin, in the summer, I sat in a room and I interviewed 26 engineers. Real world engineers, solving real world problems about what they were doing. I’ve done that three years ago before that, before COVID had to take a two or three year break, and three years ago, none of those guys were talking about AI. And I think last year about half of them were either working on AI and implementing their solutions or thinking about doing it as well.
So I think that shows to me that if people aren’t doing it already, they’re already thinking about it. And I think like you say, if their competitors are doing it, then there’s no real choice. If it really is something which is going to improve productivity, which is what we all want. Then if your competitors are improving their productivity, you have to do the same thing. So I’m sure that that will drive future generations of engineers to be using AI regularly, I guess.
Jousef Murad (32:16):
Absolutely. I would 100% agree.
Stephen (32:18):
So talking a bit, we’ve talked a bit about the future of AI, but I wanted to finish off by talking about the future of engineering and engineers. So we talked about at the start about how your experience as kind of a generalist is very different than my experience as a specialist, which is the difference in 20 odd years of evolution in the engineering industry I’ve come across a guy called James Plummer who used to be the Dean of engineering at Stanford, and he has a talk called, The engineers of the future will not resemble the engineers of the past. And he says, “Careers are becoming global and unpredictable, lifelong learning is essential.” And his point is that the half life of engineering knowledge is three to five years. Which is controversial for somebody like you who finished university three to five years ago. Firstly, do you think that’s true? Is the stuff that you learned in university still relevant or are you having to learn to keep up with changes in the industry?
Jousef Murad (33:21):
Oh, I love the question, Stephen. Really good question. I’m not sure if that’s true, to be honest. I would have to check myself. But there’s this thing called the Ebbinghaus Forgetting Curve, which is like an exponential decay of knowledge. So there’s some time where your knowledge actually decreases, and then you basically have to do some kind of space repetition to actually get back to a specific baseline of knowledge. My general recommendation is always to check, maybe go on Twitter and check out the latest publications if you want, and what’s going on in the AI simulation space? What is the latest solver and whatnot? And also keep yourself up to date with maybe learning, a continuous learning. You can go to Coursera, for example, to have excellent machine learning, deep learning courses for engineers. So I think having an excuse like, I was in uni and I forgot most of it.
I think it’s not an excuse anymore. You can literally just go to Google and learn everything you want, whether that’s statistics, machine learning, or you even want to start to learn a new program, whether it’s Cree or any other CFD tool. So I think the engineers of tomorrow, what they have to be able to do is actually learning how to learn. And I think this is one of the most essential skills. So if a new project comes, how do you actually start the project and actually get up to speed very quickly and actually learn that skill? And you can’t then say, well, I didn’t learn that in uni. That’s just a bad excuse, I feel.
Stephen (34:37):
And increasingly, people are learning skills from YouTube. For me, I’m the textbook generation, but even I’m increasingly turning to YouTube. And so we’re talking about lifelong learning. It would be wrong for me not to try and plug your podcast and your YouTube channel. I noticed today actually that your content, lots of it is just you talking to engineers and learning from other engineers. There’s also lots of technical content as well, like learning how to derive the Navier-Stokes equations, which I thought was interesting. Tell us a bit more about what’s on your channel for engineers who want to learn and improve their skills.
Jousef Murad (35:11):
So the channel is mostly about engineering and science, and I tap into the minds of very great minds of engineering, but also other fields such as, for example, neuroscience, which is also a field that’s very interesting, especially for engineers. And also self-improvement, which I think is also very critical. So work life balance and all these kinds of things. So if you’re interested in becoming a generalist, feel free to check out my channel. And obviously I really much appreciate you having me on your show, Stephen. I’m not an expert by any means. I know a lot of things quite well, but I don’t know a lot of things very well. So if you want to have a talk with me, come to my podcast, feel free to do so, and I’ll reach out to him. I’m more than happy to help you out and have you on my show and let’s discuss some cool stuff about science.
Stephen (35:57):
And so thank you very much, Jousef. I highly recommend the podcast. It’s been great talking today and learning about AI. Thank you very much.
Jousef Murad (36:04):
Thank you, Stephen.
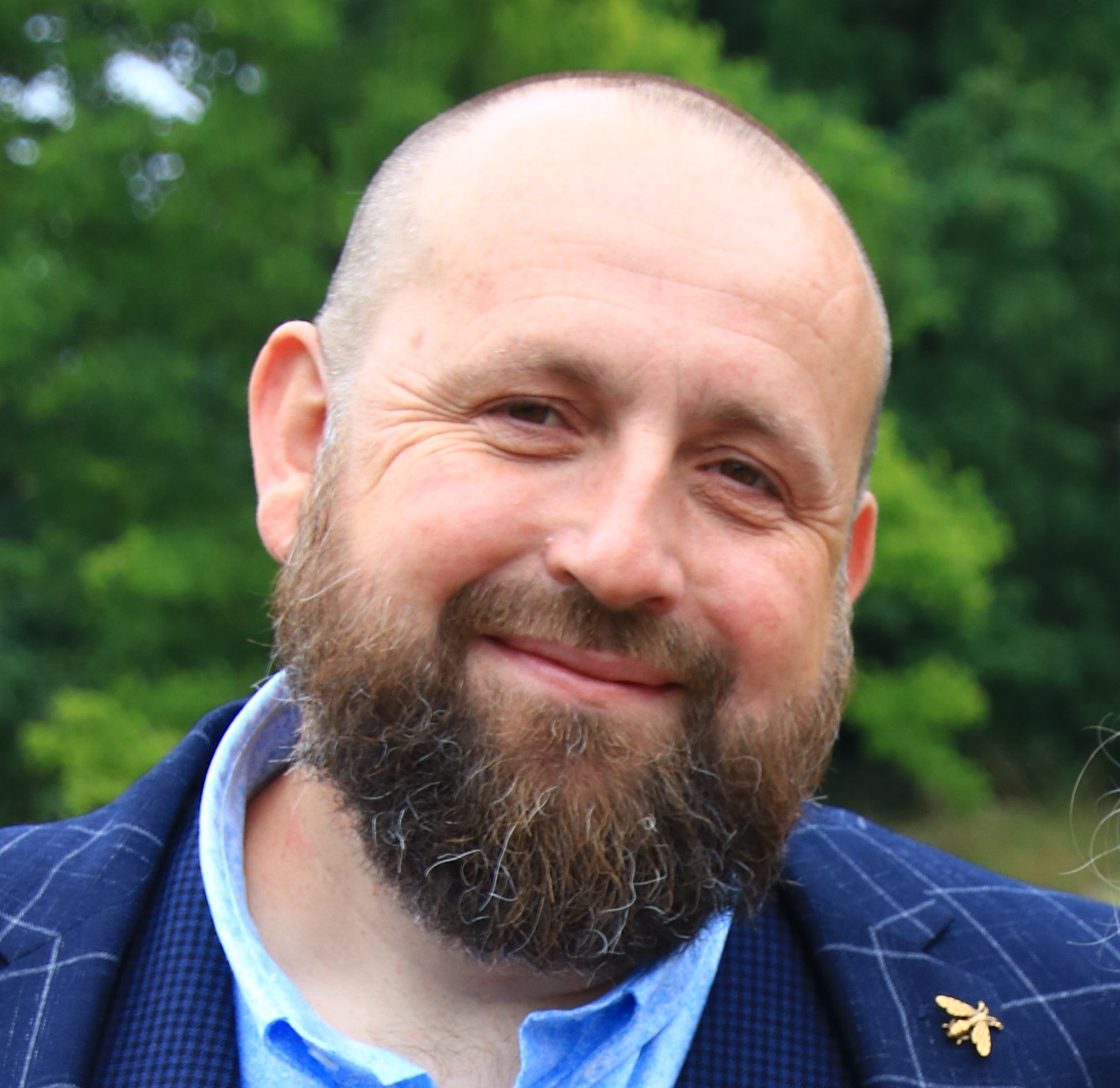
Stephen Ferguson – Host
Stephen Ferguson is a fluid-dynamicist with more than 30 years of experience in applying advanced simulation to the most challenging problems that engineering has to offer for companies such as WS Atkins, BMW and CD-adapco and Siemens. Stephen’s experience of AI and ML is limited to late night experiments trying to convince ChatGPT to do something genuinely useful.
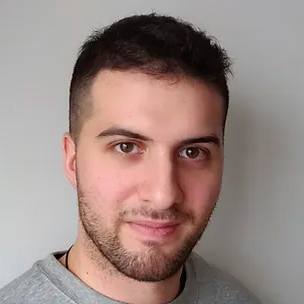
Jousef Murad – Guest
Jousef Murad is an engineer, YouTuber and a podcaster, and one of the biggest social media influencers in the world of engineering, science and simulation. Jousef is the the host of the Engineered Mind Podcast and YouTube Channel, which has over 200k subscribers and the author of the Sunday Science Newsletter.
Take a listen to the previous episode of the Engineer Innovation Podcast. Series 2, Episode 4: Experiencing Digital Twins in the Industrial Metaverse.
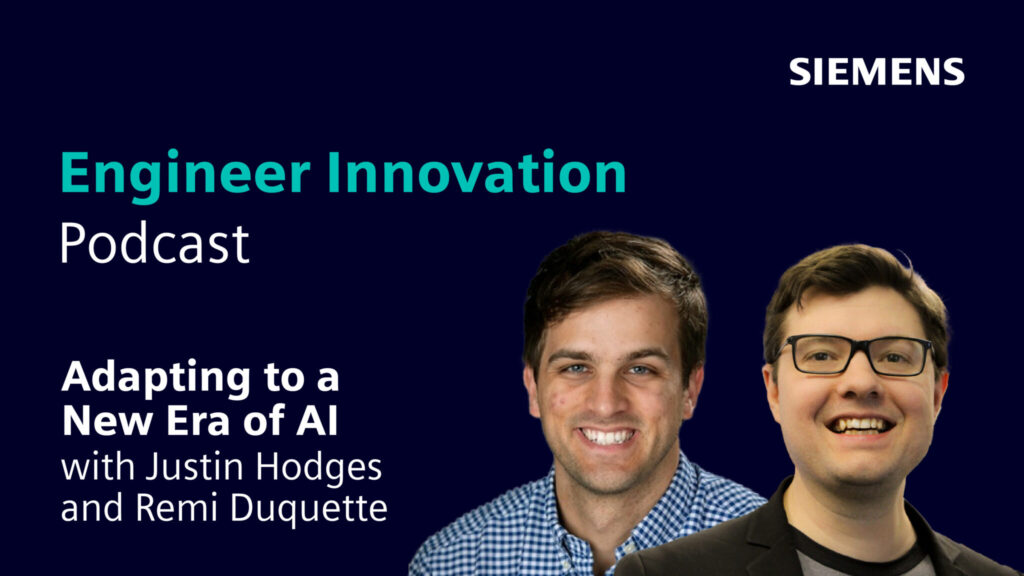
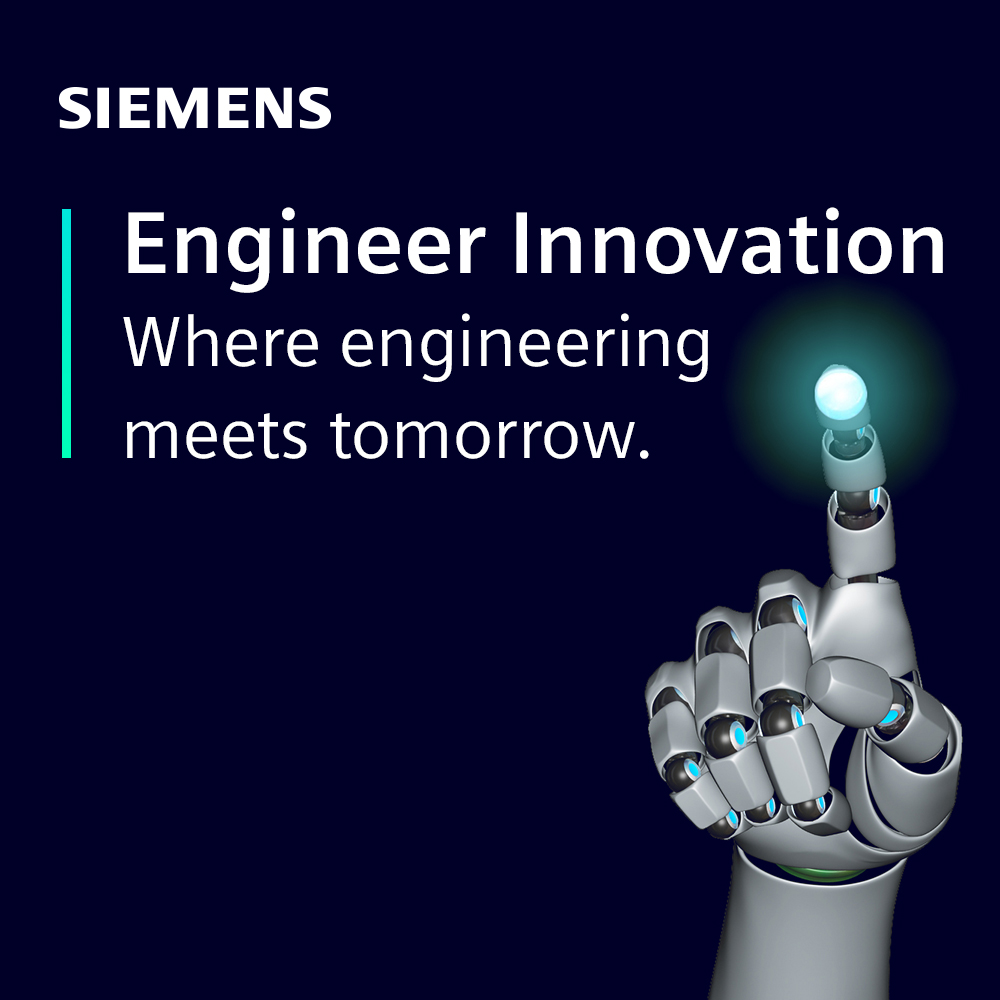
Engineer Innovation Podcast
A podcast series for engineers by engineers, Engineer Innovation focuses on how simulation and testing can help you drive innovation into your products and deliver the products of tomorrow, today.