Austin’s butterfly and the evolution of adjoint industrial optimization
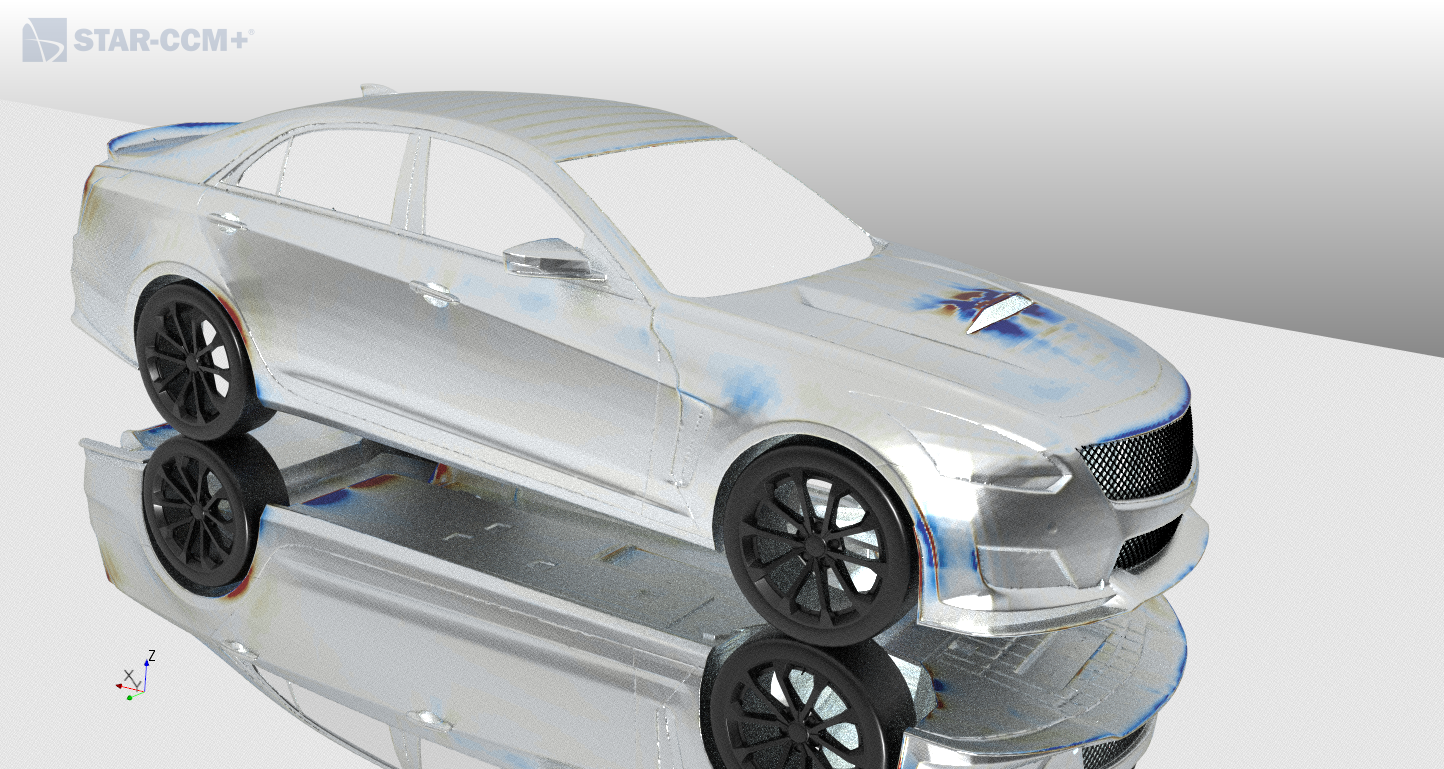
Have you ever heard of Austin’s butterfly and Student-Engaged Assessment? It’s an educational method developed by Ron Berger from EL Education. It is based on the transformational power of models, critique, and descriptive feedback to improve student work. The method is explained in the book Leaders of Their Own Learning: Transforming Schools Through Student-Engaged Assessment.
Austin, a first grader student, used this method for sketching a scientific illustration of a butterfly. Through multiple updates based on feedback from his peers, he managed to transform his sketch into a high-quality final product. You can watch Ron Berger describing this in Austin’s Butterfly: Building Excellence in Student Work. The impressive results can be seen in the picture below.
Austins’ butterfly
The method is based on the following principles:
- Complete product in each cycle
- Evolve product through critique/feedback
- Continuous improvement
It is known to equip and compel students to understand goals for their learning and growth, track their progress toward those goals, and take responsibility for reaching them, whilst developing a growth mindset.
Simcenter STAR-CCM+, like Austin’s butterfly, is built on these same principles. Through Ideastorm (our innovation forum to submit new product development ideas) users feed our development process, resulting in 3 major releases per year. This ensures that we continuously deliver innovative technologies to broaden your application scope and respond to your evolving simulation needs. Adjoint optimization has been part of Simcenter STAR-CCM+ for many years but it is not until recently that it has started attracting attention from our users in industry … and we listened to their feedback.
The adjoint solver is a very powerful tool that can provide sensitivity information on any given objective with the cost of one solution. For example, in the image below you can see the surface sensitivities of a vehicle with respect to drag reduction. Where red means pull, and blue means push the geometry to achieve improvements on the objective. These sensitivities can be used for changing the shape manually or even perform automatic optimization.
Adjoint surface normal sensitivities with respect to drag reduction
More and more customers have been requesting new features or ask for improvements in the usability and convergence of the adjoint solver. In order to meet our customer’s needs and enable continuous improvements going forward, we completely refactored the solver in Simcenter STAR-CCM+ 2019.2. The new solver uses less memory than the previous version. As a result, optimization of heavy industrial cases is easily achievable and, in some cases, 2nd order adjoint can run successfully.
The defaults were revisited, and they are now more suitable for a wider range of applications. They offer improved stability, convergence and speed in most cases. Best practices were built based on the extensive testing that was undertaken for proving the benefits of the new solver.
The cost functions definitions were moved under the adjoint solver. This change improves the workflow and allows more comprehensive setup of multi-objective optimization cases by reducing the travelling up and down the tree.
Once the solver was refactored, additional improvements naturally followed within months. We were able to quickly implement the idea:”D2593: Provide adjoint of turbulence” offering our first differentiated turbulence model. Namely, the adjoint Spalart Allmaras model. This model was chosen naturally, as it is a one equation model, and was suitable for the first release. Further, more commonly used, turbulence models will follow.
The adjoint turbulence model improves the accuracy of the adjoint solution compared to the “frozen turbulence” approach as it is directly considering the effects of turbulence. This model is naturally more beneficial and suitable for external aerodynamics aerospace applications. The Spalart Allmaras model was differentiated using the in-house automatic differentiation tool which provides tested and very accurate differentiation.
Another development that was inspired by Ideastorm (D2035: Add a trigger for starting the Adjoint Solver) is the adjoint workflow with the Simulation Operations tool. Simulation Operations allows you to orchestrate and seamlessly execute your simulation operational sequence, natively, without code. In the case of adjoints in a typical workflow you need to run the flow and the adjoint solution and then perform some kind of deformation or even just calculate the sensitivities. Before version 2019.2 macros were essential in this process. But now with a few clicks that you can see in the video no macros are required anymore.
Three major features, all inspired by customer feedback. They, together, represent a complete product that is continuously improving. Automation and tackling industrial applications all ticked! I am excited about the next stage of this “butterfly”. Aren’t you?