AI and the executable digital twin
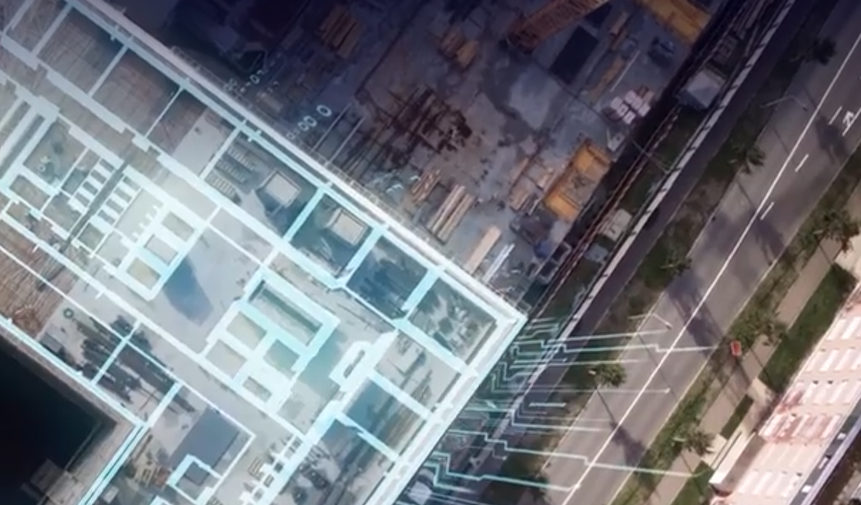
In the world of engineering, design, and manufacturing the idea of testing and validation using simulation is nothing new. Now in the age of AI we’re starting to see the rise of more advanced simulation based on the comprehensive digital twin, which allows for continuous improvement by integrating real world data back into the simulated model. This enables a positive feedback loop of innovation driven by AI and rapidly advancing compute technology. But as amazing as a comprehensive digital twin is, by its comprehensive nature it can also be “too much” for some applications which is why the executable digital twin, or xDT for short, has been developed.
An executable digital twin is essentially a compact version of the comprehensive digital twin designed to run lighter and be executed in operational conditions. The xDT relies on several techniques based on the principle of model-order reduction to simplify areas of the simulation that aren’t being actively tested and modified, substantially reducing the complexity of the model being worked with. For example, if you have the digital twin of a car it would include things like drag, friction, stresses on the internal structure, sensor data, and engine characteristics. Some of these, especially drag on the body, are very computationally intensive to calculate. If you don’t intend to change body shape, it then it can be reduced to a more simplified model while you are exploring different engine configurations. This is where the xDT starts to shine. With an xDT, all the aspects that didn’t relate to engine could be simplified into a highly accurate approximation allowing the model to run even on an edge device that normally wouldn’t be able to handle the rigors of full 3D simulation.
Of course, it is easier said than done to accurately simplify something like a digital twin. Under the hood many of the governing mechanics are defined by complex systems of non-linear equations, difficult to accurately solve at the best of times, and asking for further simplification might be beyond the ability of data-driven modeling and traditional numerical solvers. But those aren’t the only tools in our simulation toolbox anymore. A deep neural network can be trained with all the data from the full digital twin and simulation environment and the resulting trained network packaged up with the xDT, providing a result similar to a numerical approximation based on all available data, both simulated and real.
This is one area of AI that isn’t talked about as much, its ability to infer. For a human, the ability to estimate and extrapolate is second nature but it traditionally hasn’t been something computers are good at, or even capable of. Computers can use numerical and statistical models to extrapolate results based on any amount of data, but the models need to be manually created and tuned for every set of data which is impractical when working with smaller datasets and specialized systems which require one-off solutions. But with AI, computers can work with data much more naturally, almost like a human would, while still benefiting from a machines ability to handle large and complex datasets, making it perfect for estimations in areas where extrapolating from data is easier than trying to find a mathematical equation. Compared to other solutions, a trained AI model is also something that can be deployed to an edge device, allowing the benefits of a massive training data set to be condensed onto something that will fit in your hand.
In the realm of data, its hard to think of a more impactful tool then AI right now. The ability to process vast quantities of information into an accurate predictive model is something that can benefit almost any field. But just as exciting is the prospect of using AI to replace, at least to some extent, highly complex mathematical equations used in physics modeling and other areas of simulation that traditionally rely on computationally heavy numerical models. Instead, you can train a neural network with the expected solutions based on real world data. As a mathematician, I find the prospect of being able to “solve” nonlinear partial differential equations via extrapolation from real-world data efficiently a fascinating one, especially since many of these equations can already only be solved approximately using real-world data to begin with. AI presents a great opportunity to make physics simulations more accessible and more accurate, bringing the power of a datacenter to every workstation and tablet.
Siemens Digital Industries Software is driving transformation to enable a digital enterprise where engineering, manufacturing and electronics design meet tomorrow. Xcelerator, the comprehensive and integrated portfolio of software and services from Siemens Digital Industries Software, helps companies of all sizes create and leverage a comprehensive digital twin that provides organizations with new insights, opportunities and levels of automation to drive innovation.
For more information on Siemens Digital Industries Software products and services, visit siemens.com/software or follow us on LinkedIn, Twitter, Facebook and Instagram.
Siemens Digital Industries Software – Where today meets tomorrow.