AI Spectrum – Exploring Siemens NX’s Smart Human Interactions Feature Part 2 – Transcript
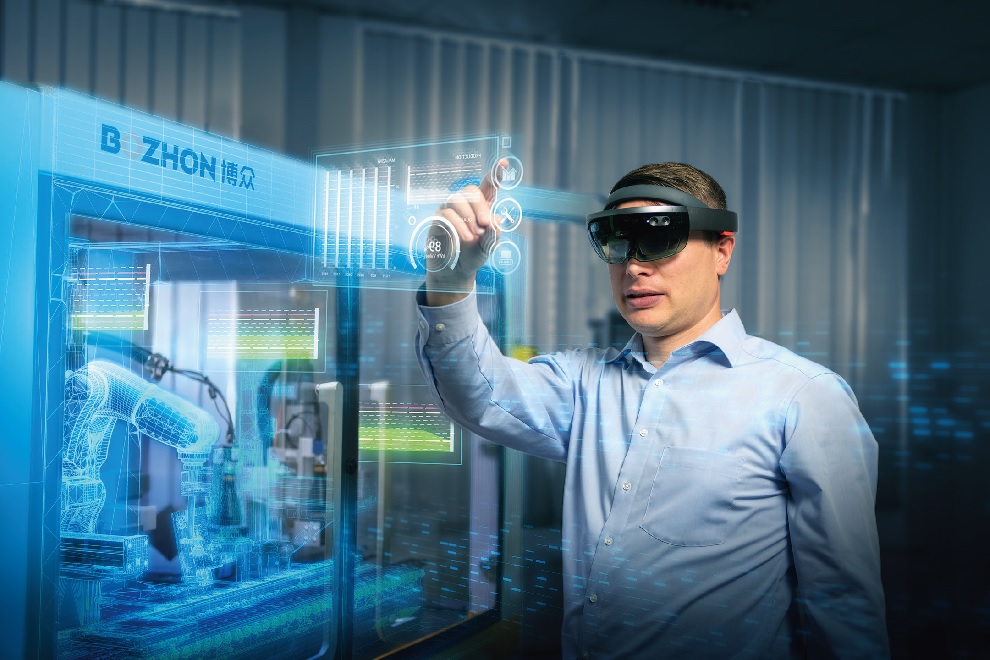
In a recent podcast I got to chat with Shirish More on how NX is using AI to drive smarter human-computer interactions, how they are developing new and innovative ways for designers to interact with design software and even redefining the relationship between designer and software.
To listen to part 1, click here.
For the full podcast of part 2, click here.
For those that prefer to read, the full transcript of part 2 is below and a link to the transcript of part 1 is available here.
Spencer Acain: Hello everyone, and, welcome to part two of the AI Spectrum podcast on AI in NX. As mentioned in our previous podcast, we are talking to experts all across Siemens about a wide range of artificial intelligence topics and how they’re applied to various products. I’m your host Spencer Acain, and today we are again joined by Shirish More to discuss how Siemens’ NX platform is using AI. Welcome, Shirish
Spencer Acain: Last time, we ended our talk by discussing how NX is pushing the boundaries of smart human-computer interaction by closely integrating human and computer work to become a collaborative process rather than merely having the explicit input of a keyboard and mouse.
Shirish More: Yeah, that’s that was a very good point, keyboard and mouse. But keep in mind, we are entering into a world of virtual reality and augmented reality, wherein we don’t easily have access to mouse or keyboard. So, in those cases, this is a perfect example, wherein if we have this smart human-computer interaction going, I can just wear those VR goggles or a Meta setup, and I can say, “Okay NX, take a section in XY-plane.” Rather than going through some complicated user interactions, wearing my goggles, we can just ask NX to perform certain actions without even worrying about how is the user going to take a section, for example, while he’s wearing a VR goggle. So, you see how we are taking the desktop experience, how we are learning from how users are using NX while he is designing a part to a next level, wherein if he has a process setup, if he has a command that he knows that NX has learned from his actions, and if he has a skill, and if he has a voice associated with that skill set; we can easily take it further to virtual reality and other areas wherein he really doesn’t need any keyboard or mouse, he can just say, “Okay NX, take a section. Okay NX, release my design. Okay NX, apply this visualization material, I would like to see this design in a shaded mode.”
Spencer Acain: It sounds like this is also an important first step toward that augmented reality that new generation of how we interact with the digital world and computers in general. So, you’ve mentioned prediction a few times now – so, both in selection command prediction and predicting how user will interact with a given part or model based on what they’ve used in the past. Are there any other ways that you’re bringing prediction into NX to help drive the user design experience?
Shirish More: That’s a very good question. There are two things happening. Prediction is a huge workstream for us. Anything that we can do inside of our design environment to speed up, the overall design process is huge. And there are a number of opportunities for us wherein we can predict things. And we just covered two areas wherein command prediction is a perfect example wherein you know that day in day out, I work on such types of design, can you start predicting a sequence of command? Or can you start recommending or predicting commands that I normally use and bring it in the front while I’m designing? That really speeds up the overall process. But that’s an example of how we learn while user is using our software NX. And then based on his actions, we can start predicting the commands that he is going to use. But then there is another aspect to this whole prediction. As I mentioned earlier, when designers design their design, and once it gets approved, meaning that it’s a proven design, there is a lot of engineering knowledge that goes inside of those designs. What we have started doing is we have enabled customers to extract the engineering parameters from their proven designs, and then generate a machine learning model out of it. And once they deploy this machine learning model, NX can start predicting; “Well you are working on an injection-molded part, we predict that you might need a draft angle for this particular cavity.”
Shirish More: So, you see where we are taking prediction. We are taking prediction to a next level, wherein not only we can predict things based on how users are interacting with our software, but we have a way in which we can leverage their existing design knowledge or existing user knowledge which is stored in their proven designs, we can extract that information, we can learn from it. And then once the user encounters a similar context or a problem within our software, we can start predicting the parameters that he should be aware of, meaning that “Well, looks like this is a plastic part, I know exactly which material. I cannot predict 100% to which material unit to use, but at least I can serve the list of five proven materials that, based on their past experience, the designer needs.” So, we are taking prediction to a next level, wherein not only we can predict the user interactions, and selections, and entities while he’s working. But we can also start predicting parameters that he needs to use, or he should be at least aware of so that we reduce the number of iterations between design and simulation and manufacturing. So, we are trying to get designers to design right the first time so that we reduce the number of iterations and thus speed up the overall product development lifecycle.
Spencer Acain: That’s actually very cool. It sounds like a great way to capture the knowledge of previous designs and to re-integrate that without needing to train every designer and every engineer in the process; they don’t need to look at every design because the computer is doing that for them.
Shirish More: That’s exactly where we are heading.
Spencer Acain: That sounds to me like it would be a boon for sustainability as well because you can see this, you reduce the number of iterations, you’re improving the design right off the bat with good practices that you’ve developed over time.
Shirish More: That’s exactly what we are enabling. And the way we are enabling that is by using inbuilt machine learning or AI framework and infrastructure. What it means is that there are two ways in which we are speeding up the overall design processes here is by deploying the machine learning models with out-of-box NX, meaning that from a customer’s perspective, they get a generic model, and they can just leverage it. There is no pre-processing needed on their front; they can just install NX. And for command prediction, for example, or for selection prediction, they can just leverage the out-of-box machine learning model. But once we get to parameter prediction, or once we get to predicting certain engineering parameters based on the industry in which the user is using our software; we need a way in which a given customer has to have a mechanism in place that allows them to say, “Well, I have these 10 proven designs,” or “I have these hundreds of proven designs that I’ve been reusing for n number of years. Can you extract the knowledge from these 100 proven designs? And from that point onward, next time when my user is going to design something similar, can you guide him in making sure that he uses parameters that fall within a certain range?” For those kinds of use cases, we also have ways in which customers can leverage our framework and infrastructure to train their custom machine learning model, which is very specific to their workflows. And they have the ability now to extract the knowledge, learn from it, and deploy a custom machine learning model within their premises. So, as you can see here, NX as a software supports both out-of-box machine learning model which are pretty generic, like selection prediction, command prediction. But then we also support ways in which customer can leverage their existing data to train a custom machine learning model, which can speed up their overall processes. And this custom machine learning model can remain within their premises.
Spencer Acain: So, we’ve been talking about having, basically, expert users and just users in general training the software as it goes. But you’re saying that there’s also a way to just frontload the training if you have a lot of good existing designs, you can take a lot of that information that you already would have stored and just put it directly into NX before you even put it in the hands of your designers.
Shirish More: That’s correct. So, when it comes to NX, the example that we covered earlier – expert user versus a new user – was more from using NX perspective; “How can I reduce the learning curve that a new user needs to go through when he is using NX for the first time?” So, in those cases, if he has an out-of-box machine learning model or if he has a model that was developed using expert users’ interactions, he can just speed up the overall processes while he’s using the NX. But then the second example that you just mentioned is, yes, not only from NX usage perspective, we can speed up how a given user is using NX, but leveraging their existing data to train a custom machine learning model around a specific process is huge, because now you don’t have to ask a user to look up a best practice or look up as to how to create so-and-so part or how to create so-and-so design. If they have proven designs within their premises, they can leverage our machine learning framework and infrastructure to train custom machine learning models and deploy those in their production environment.
Spencer Acain: Sounds like we’ve covered quite a bit of what NX is doing with AI right now. But is there anything you’re looking forward to or planning to do with AI in the future involving NX?
Shirish More: There are definitely a couple of key areas. One is going back to the smart human-computer interactions. Nowadays, if you look at the hardware that we are getting and with people working from home, almost all of our laptops do come with inbuilt cameras. So, we are looking into some advanced gestures; how users can use the gestures, either the hand gestures or the face gestures to speed up the design process. So, that’s one area where we are looking into. Rather than investing in hardware like Space Ball and other things, can I just use my hand to drive? For example, I want to rotate my design about an axis, can I just perform that gesture in front of the camera and say, “Okay, can you rotate this?” And NX design should react to that gesture. So, that’s one area where we are looking into.
Spencer Acain: It sounds like that would integrate well with what you were talking about with VR and AR since that’s very gesture-based as well.
Shirish More: Exactly. Going into the next area. We talked about workflows, but how can we leverage the machine learning to speed up, not only from a command prediction perspective but for example, if we detect a pattern and we know the context in which the user is using NX, can I help the user drive the entire workflow? For example, if I’m working against a sheet metal part and NX detects that; well, traditionally, this user always creates a mid surface for a sheet metal part. If, from a collaboration perspective, that sheet metal design goes to a user who is not aware of the best practices, we can prompt to the user, saying, “Do you want me to create a mid surface?” And the user says, “Oh, yes, thanks for reminding me. Go ahead and create a mid surface for me.” So, that’s where the robotic process automation or workflow prediction will come in handy. So, not only we are predicting commands and selecting entities and predicting parameters, but we’d like to get to a stage where we can predict the entire task, and we should be able to automate some of those workflows, or at least start guiding designer in triggering the appropriate workflow at the right time. So, the moment we detect a pattern, the moment we detect a state in which this design is, we can say, “Well, I think you have reached to a point where you should assign a material,” or “You should create a mid surface,” or “You should calculate the weight.” We are trying to see how can we detect this pattern and predict things or predict workflows so that the user can say, “Okay, I like what NX you’re predicting, go ahead and execute that workflow for me.”
Spencer Acain: That really sounds like it would be a huge asset for design companies. It almost sounds like you’re having the expert knowledge and the correct workflow moving with the design as it goes from person to person, as opposed to relying on each individual person’s knowledge to correctly design something to do all the right steps at any given time.
Shirish More: Exactly. So, it’s not only going to complement our help documentation, but each customer, each organization that I normally work with, they have their own best-practices document. And traditionally, they have asked the user, “Well, if you’re working against the sheet metal design, make sure you read this document and understand how we ask our users to create the mid surfaces or organize the entities in your design so that downstream processes don’t break.” But rather than asking the users to read a document or follow certain steps, I think we are trying to reach to a point where we detect a state in which his design is and we can prompt him with a question saying, “Do you want me to create a mid surface?” And if he says yes, we can just execute a sequence of commands or guide him in creating a mid surface. So, what we are proposing here or working on is coming up with a framework that will help the user speed up his overall design process with recommended best practices.
Spencer Acain: It sounds like you’re not only speeding up the best practices and the workflow and everything, but it’s a way to cut out the bureaucracy or the busy work or just dealing with making sure that everything follows a strict style guide implementation and letting the designers really get down to the design work. And instead of having to organize lists or to make sure that everything is exactly the way it’s documented it has to be. Is there anything else you’ve been working on recently that we didn’t talk about in relation to AI or even outside the company?
Shirish More: Personally, I keep an eye on how other industries outside of PLM domain are taking advantage of machine learning. We are all getting used to smartphones, streaming devices, and these devices intelligently configure themselves based on the resources that we have. And when I say “resources,” the internet bandwidth, for example, or you might be in a location where you can’t use voice but still it predicts things and it prompts things and other things. So, we are closely keeping an eye on how machine learning, as a subset of artificial intelligence, is getting leveraged in a number of domains outside of PLM. And we are trying to see how we can apply some of those techniques inside of our domain as well to speed up the overall product development process. And not only NX, in the end, we are trying to apply machine learning techniques to a variety of design sources. And NX itself is one design source, but then what gets loaded in NX can come from different systems. So, how can we apply machine learning techniques to a variety of design sources to solve engineering problems under a variety of contexts? Because the models that we come up with need to be smart enough to adapt itself to the industry, adapt itself to the user’s skill set, or adapt itself to the context in which it’s getting called. And for us to do that, we are putting some smarts in NX so that the NX can start leveraging advanced machine learning techniques to make the designer happy or to speed up the overall processes.
Spencer Acain: That’s very interesting to hear how you’re just pulling stuff in from everywhere to make not only NX better but the entire design experience. I think that would be a good place for us to stop. Once again, I have been your host, Spencer Acain, and I would like to thank Shirish More for joining me here.
Shirish More: Thank you, Spencer.
Spencer Acain: This has been the AI Spectrum podcast.
Siemens Digital Industries Software helps organizations of all sizes digitally transform using software, hardware and services from the Siemens Xcelerator business platform. Siemens’ software and the comprehensive digital twin enable companies to optimize their design, engineering and manufacturing processes to turn today’s ideas into the sustainable products of the future. From chips to entire systems, from product to process, across all industries. Siemens Digital Industries Software – Accelerating transformation.