Go beyond printing smart, to smart printers
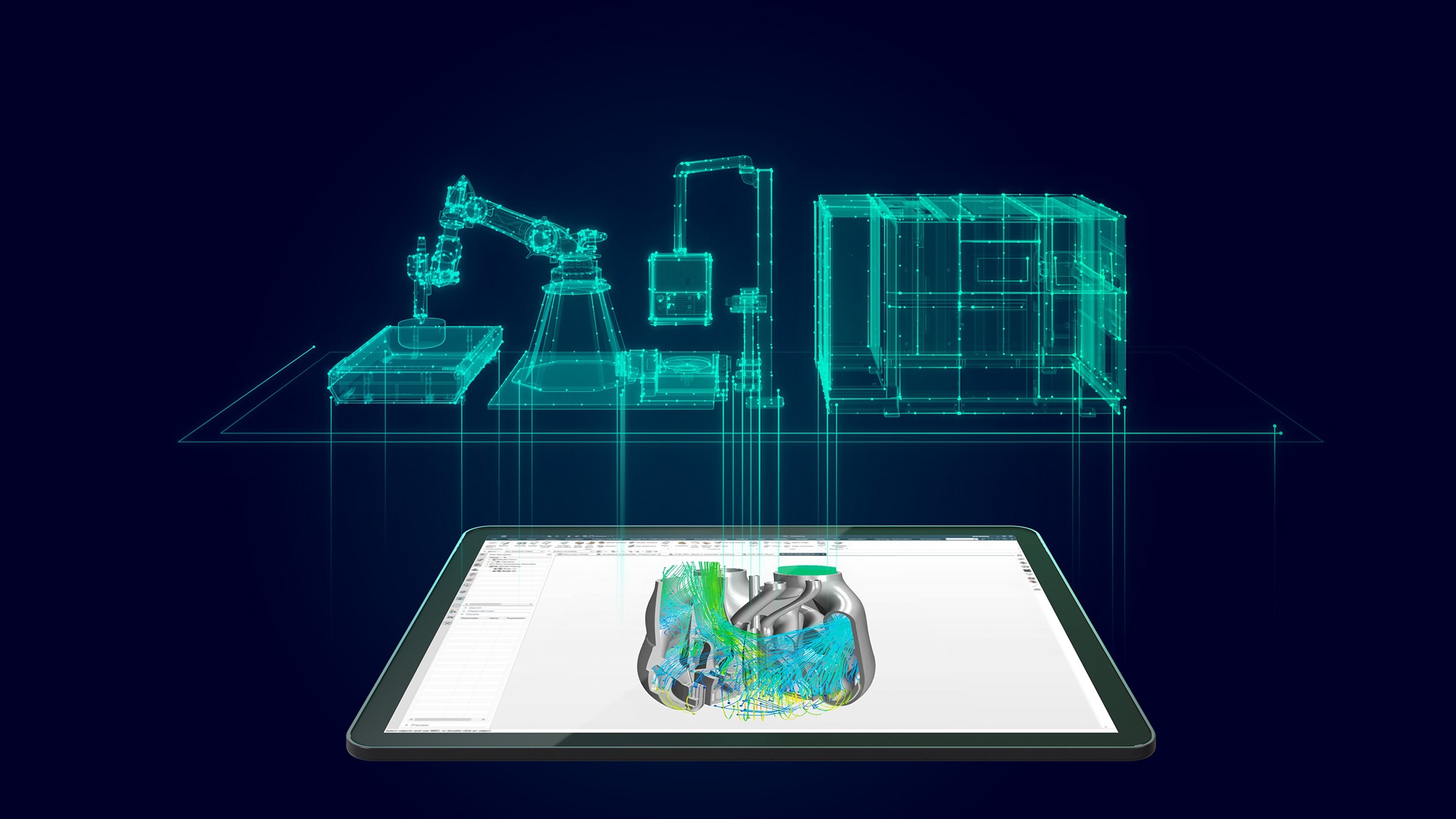
Additive manufacturing (AM) has so many amazing technologies and processes that have expanded the usability, reliability, and viability of printing as a major manufacturing option. Software has brought design for additive ever closer to what is possible with other manufacturing methods. There are geometric opportunities enabled by better machine accuracy and multi-axis capabilities. And over experience acquired across the industry have provided simplifications and optimizations to make additive a choice for more industries than ever before. But even with all of the progress there are still improvements to be made, especially in our understanding of the materials during the printing process. There might just be a possible solution to greater success if the findings out of the University of Cambridge – machine learning used to detect and correct print errors – can be adopted widely.
A different material problem
Many of the lingering problems to solve around additive manufacturing arise from the material properties of the printed substances – metals, plastics, and composites. Our understanding of these materials comes from their application in other manufacturing process and need to be corrected for an extremely different method. Metal casting and injection molding led to an understanding of how materials change from semi-liquid to solid structures under constant pressure, but extrusions from a printer instead rely on gravity-induced pressure between layers without uniform pressure.
There is also the variable of temperature. Forged, cast, injected, and many other traditionally manufactured parts have relatively consistent temperatures across a mass of material. This, in general, leads to more uniform material properties and fewer material phases. Granted, there are edge cases – a CNC operation on a thin part can create enough friction to warp a material and certain mold geometries impact the uniformity of cooling, impacting the part’s final composition. But in AM, this can happen at any point in the print because in many processes it is continually melting and solidifying material to cooling regions. This can alter the adhesion of layers, deform the part from unexpected forces, and even change the material properties of regions.
A software solution
There are some software solutions to these challenges in printed materials. An engineer might run simulations geometries to understand how a printer might handle the novel situation. This involves simulating the length of a print path to understand how the heat transfer from the print head impacts the surrounding material. The problem though is that this approach is very computationally intensive and the path lengths can stretch for miles, even in small prints. Another tactic is to simulate orientation adjustments for the printed part in the print volume. This relies on experimental understandings, an example might be to avoid printing above thin structures or to back-track within a print layer to allow modest cooling before return to an area that could experience overheating.
The problem with solutions available today is that they are expensive, some in time, others in institutional experience, and more in cash investment because of the computational requirements. Engineers have to continuously improve their prints based on the behaviors of specific printers and geometries. But they cannot account for every situation, and definitely not fast enough to correct a print in real-time, which means a part may fail before in can be made successfully. And none of this can readily account for the truly unexpected, even with print monitoring.
Applying machine learning
What tool is great at analyzing lots of information rapidly and automating operations? Machine learning, which is the reason for the researchers at the University of Cambridge applying it to print monitoring. Their system was trained on nearly a million images captured during prints of 192 objects, with information on printer settings, to start categorizing the sea of information. The model also received secondary adjustment values to make the prints good, to allow the system to learn how to make adjustments and how errors might arise. But while this monitoring model can make assumptions between different materials because they are all essentially semi-liquid coming out of the nozzles, there are still missing variables at the microscopic level that could be integrated for metals and plastics.
An exciting future
While the solution from the University of Cambridge might not be valuable for manufacturers just yet, there is massive potential in this line of thinking for in-situ monitoring of prints. It might even be applied to hybrid printers so that an uncaught mistake could cause the machine to switch to CNC milling operations almost as an eraser for the error. But no matter what innovative solutions come from this research, all of them will rely on a broad understanding of the component and system as you’d find in a comprehensive digital twin – with simulation and geometric understanding.
Siemens Digital Industries Software helps organizations of all sizes digitally transform using software, hardware and services from the Siemens Xcelerator business platform. Siemens’ software and the comprehensive digital twin enable companies to optimize their design, engineering and manufacturing processes to turn today’s ideas into the sustainable products of the future. From chips to entire systems, from product to process, across all industries, Siemens Digital Industries Software is where today meets tomorrow.
For more information on Siemens Digital Industries Software products and services, visit siemens.com/software or follow us on LinkedIn, Twitter, Facebook and Instagram.
Siemens Digital Industries Software – Where today meets tomorrow