AI Spectrum – Simplifying Simulation with AI Technology Part 2 – Transcript
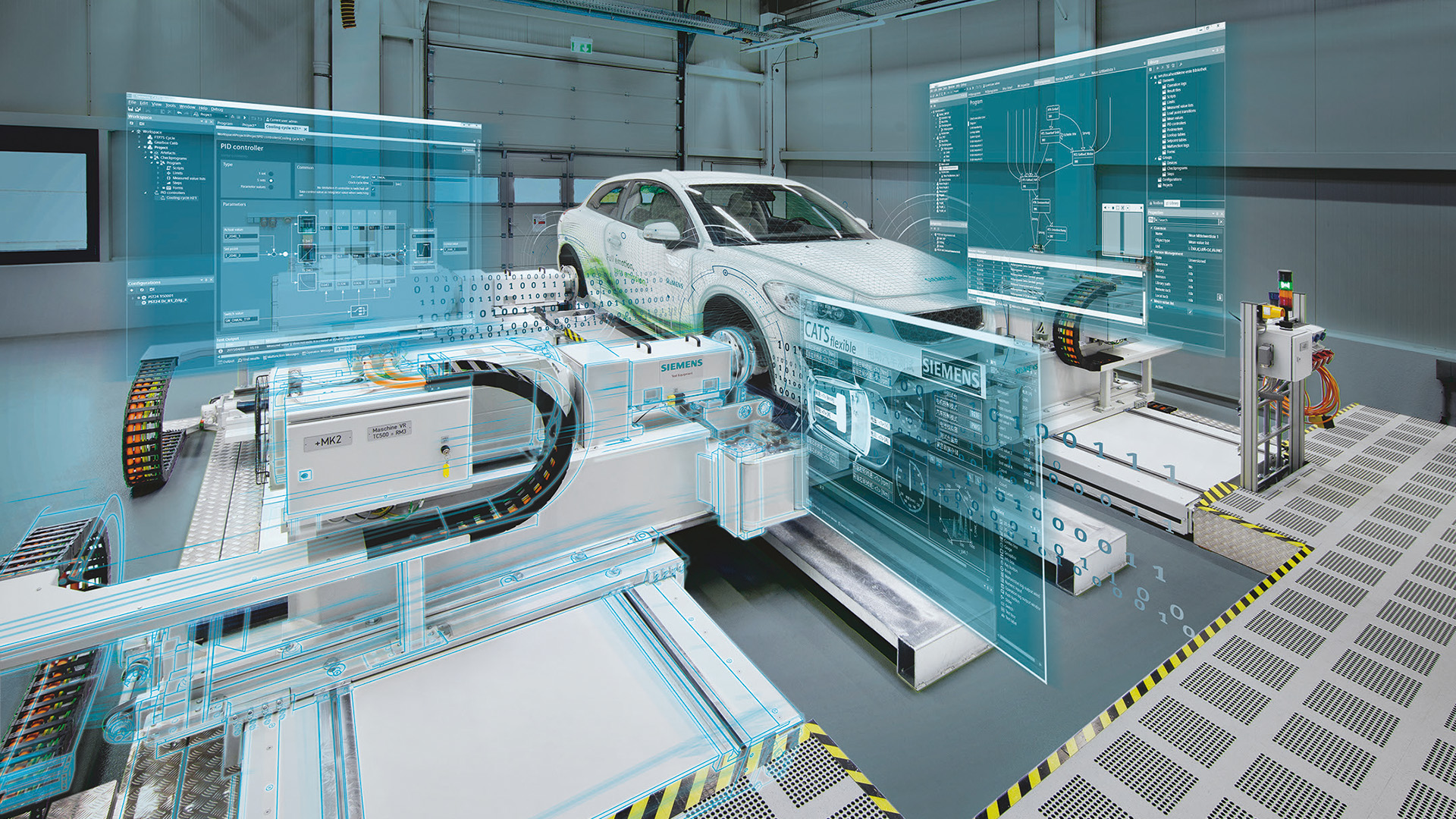
AI is changing the face of simulation, and I recently had the opportunity to continue my conversation with Dr. Justin Hodges on exactly that topic. In the short time we had, Justin highlighted for me the ways AI is capturing design knowledge, and some of the many benefits of AI-driven simulation. Check out the podcast here or keep reading for the full transcript.
Spencer Acain: Hello and welcome to the AI Spectrum podcast. I’m your host Spencer Acain. In this series, we talk to experts all across Siemens about a wide range of AI topics and how they’re applied to different technologies. Today, I am once again joined by Dr. Justin Hodges — an AI/ML Technical Specialist and Product Manager for Simcenter to continue or discussion on how he and his team are using AI to advance simulation and design software.
Spencer Acain: Picking up from where we left off last time Justin, you talked a little about the predictive capabilities of AI in simulation and design, can you tell us more about that?
Justin Hodges: So, the multidisciplinary physics is really the key. Let’s take an internal combustion engine as an example. At some stages, you will do cold flow type of analysis to understand how major design decisions influence the flow field, and you want to look at your secondary flow structures and optimize them in a certain way. Then you have this massive design space that’s lingering in your mind about “Well, what about my drive map, where I have to consider a broad range of RPMs that are possible and fuel economy and all of these things?” It really becomes like a layered problem of different groups that have to work together as you do start to increase the fidelity. Then I also include combustion, for example, that will surely change the answer. And then I also have to consider other connected components in the drive train other than just the actual combustion engine — the cylinder itself. So, what ends up happening is there’ll be low fidelity tools that are used at the beginning of the process to just explore some of these major decisions as far as what the design will be. And then, gradually, you layer on more fidelity with more expensive simulation, and then this will extend to other teams and things like that, which may do the same. So, again, a really novel sort of approach that AI and ML really offers as a lifeline is if I do that on say, one size engine, I could make models that would allow you to skip steps, so to speak; if I want to run three consecutive design studies on the same thing, but I want to increase the simulation fidelity each time and use a different product each time. What if I had a machine learning model that could relate as in this transfer function or correlation type way, what the output is from the first step to the output of the third step? That would really allow me to be more agile in this process and go faster. And then, ultimately, encode this knowledge into machine learning models from the different steps and the process so that when I do it again, in the next season of my job, where now I design a similar size engine — maybe bigger or smaller, some difference, but it still looks the same in some ways — I can take advantage of these surrogates, if you will, that I’ve created to populate the space initially and then start refining it. It’s kind of like a smart, intelligent DOE via transfer learning. You get a big head start is how I like to think about it.
Spencer Acain: I see that does sound very helpful, and it really ties back with some of the other things we’ve been talking about; how you can really use AI to not quite skip steps but to smooth things over and to speed things up that would normally take a long time.
Justin Hodges: Data is an asset. So, this is one way to leverage that data and make use of it beyond the time where you just generate it when you’re designing that particular component. If you have all of these designs and your history in databases in the company and this data lying around, that’s for some scenarios, a lot of really nice things to offer, not only in terms of Teamcenter and design space exploration and smart ways to sift through the results you have and suggest good ones for future features AI/ML will provide. But just generally, having these surrogates that can make use of this information.
Spencer Acain: I think you gave us a couple of examples of how AI is helping with predictive capabilities. Do you have any examples or synergies even that might benefit from AI/ML in this area?
Justin Hodges: Yeah, absolutely. We talked about some, but we’ve neglected the test space. And I think that’s a very fruitful space that can benefit from AI and ML. For example, anomaly detection is a popular one that’s out there — maybe I’m acquiring test data for a facility or maybe the turbine on an airplane. It is a very, very complicated system with thousands of parts. It can be really hard to understand why some global metric — like the temperature or the pressure — is changing in a system. It can be influenced by so many constituent components that it’s very hard to identify where it’s coming from. So, yeah, machine learning models can play a nice role there for the operators, where they can alert if anomalies are about to happen, and then you have that warning ahead of time and you can prepare accordingly; maybe ramp down or ramp up faster through resonance region or whatever the specific application is that you’re in, it can alert you with these anomalies, which would otherwise be too complex for a human eye or ear to ascertain. Another one is this interpretability and things like that. If I’m looking at this analog-looking type signal from a variety of test sensors, sometimes it’s hard to know. Again, within an anomaly scenario, if my hardware is breaking or if I need to fix something, actually, before I deploy or before I go test. These are very time-consuming and expensive exercises with very limited times. For example, wind tunnels and automotive testing are really valuable time slots there — people pay a lot of money to have them, and it’d be a shame to show up and have sensors continue to break or take faulty data and drift and maybe you don’t realize it till you get back. So there are a lot of ways that it can work on historical signal-type information and really be valuable there as well.
Spencer Acain: It sounds like, in terms of predictive capabilities and simulation design, AI has a lot to offer, along with its benefits in other areas as well. But it sounds to me as well, AI is really a constantly changing and evolving field. It’s kind of a moving target to keep up with all of the requirements that you would find in a given project or given process. Can you tell us about how Siemens is positioning itself to address this constant need for innovation change and stay on top of emergent technology, so to speak?
Justin Hodges: It’s a really good question. I saw a funny thing yesterday, actually, that I read that said something to the effect of: when you talk about moonshots in a corporate environment, big companies say they need a small company-like culture to tackle it, and small companies will say they need a big resource in order to go after it. So, it’s always challenging despite the company that you’re in. But I think that our company structure is really well supported for it; we have this long-term work and bank of patents and competence that we have in AI and ML outside of just our niche engineering simulation world. But it really enables cross-department and cross-business unit collaborations. We have technology groups that truly understand in-depth the technology, and have been creating it and using it, and so when it makes sense, we can partner with those groups. We have a very long-reaching arm to partner with academia groups as well when they try to make substantial changes and innovation in terms of how things can be done fundamentally. Same for partners; we have a ton of partners that can tell us from industry, “Hey, this is the problem today I want to solve, and this is the problem that people will value so much that they will pay for it.” So, we have both sides of the spectrum in terms of influence. And then internally in terms of our core Simcenter team, we have the right agile and collaborative mindset to work together from product management to product developments, to the forward-looking emerging technology groups for strategy and innovation. And then, as well, we have just incredible engineers all over the place in terms of customer facing and business strategy and that sort of thing. So, it allows us to take advantage of some of the things that are easier for small companies, while also the advantages and things that are well-posed for large companies. But you’re right, that it’s continuously evolving and needs continuous adaptation. So, we certainly do our best, and it’s really great to work in this environment because it’s a really nice concert that we work in between all the different groups.
Spencer Acain: That’s wonderful to hear. It sounds then that Siemens has positioned themselves rather uniquely in the field of AI with this. Can you tell us a little bit more about how Siemens is unique compared to our competitors or distinct, I might say?
Justin Hodges: That’s a fun one to answer for sure. We’ve mentioned a lot of stuff about data and making use of it and keeping track of it and allowing companies to really benefit from an efficient way, despite teams working in different disciplines, probably different countries, which is really popular today. I would say, it’s like a scope thing. We have the products at the very end point; “My CFD software needs to do blank.” We have that ability to offer that product, not just in simulation, but also in test. But then even from that total opposite side of the granular to the large scale. If I want to do optimization, if I want to do design space exploration, if I want to use Teamcenter to tie all the data together, and TC Sim to make suggestions and sort of what parts of the space are fruitful to look at. So, I would say a big piece of it, in terms of how we establish ourselves, is the comprehensive offering. And that would be also a big piece implied from what I’ve already said, which is the digital twin is a combination of physical data and simulation data as well. So, it’s a vast space, and I would say that’s one of the benefits for sure.
Spencer Acain: One of the big topics I think we’ve talked about a lot so far is that in a lot of these cases, you’re relying on AI to make the right decision; to come up with the right answer to categorize a part correctly. How are you addressing the need to build trust in this effectively black box, that is AI simulation, in your testing software?
Justin Hodges: Yep, that’s a critical one, not just in terms of the technical solution, but it’s also very important that it’s made clear to the non-AI expert. So, it’s definitely a multifaceted problem. But there are certainly solutions there that are popularly employed, industry agnostic, whether it be blind validation exercises where you segregate the data ahead of time before you do anything, and some of the data is used for training, some is used for testing, and then some of it is held completely aside and then just looked at after the full pipeline is done for validation. That’s one important way to do things. But even decisions like that, it comes down to data analysis; I need a tool that can recommend to me the best way to separate my data, the best way to know where I can apply my model and where I can’t apply that model. We have a lot of that excellent state-of-the-art post processing and commercial-leading in terms of providing that insight on the data so we understand it. This is really from a variety of tools, not just not just one specific tool. But again, back to that comprehensive nature of our portfolio that allows you to do this despite whatever product you’re looking for. And then furthermore, offering some level of transparency — so, whatever models are there, which ones to choose — letting the user see that without an opaque wall, they can pick which ones they think are right for their problem. I guess, lastly, one thing that’s fun about engineering, simulation and physics and applying AI to that specifically, is you can make physics-aware or physics-informed type features or datasets or machine learning models. And that’s where it gets really fun, because you can interpret what models will do in certain domains of the physics. And it’s fun. There’s nothing wrong with the black-box type neural networks and things like that as solutions, but it’s also fun to look at the gray boxes that can have some tie back to all the stuff we spent so many years in school learning regarding physics and engineering.
Spencer Acain: Yeah, absolutely. I have to say, I feel the same way. As someone who’s been trained and math and engineering myself, it’s nice to crack open the box a little, you can see in there all those familiar equations, principles, and whatnot.
Justin Hodges: Yeah, absolutely. Because the reality is, sometimes there are limitations — plenty often, there are limitations — so, part of it is just being pretty clear to the user and communication, expert or not, on what they are and that they exist. And AI is not the answer to everything in what we do. So, plenty of times, the traditional way of solving our physics, it really is way better than applying machine-learning approach. So, there’s also some bit there in terms of communicating that to the user for the right problem. But therein lies the fun.
Spencer Acain: Absolutely. Well, is there anything else interesting that you’d like to share with us, either from Siemens or outside the company even?
Justin Hodges: Well, there’s so much going on in the landscape of AI in our world. But I would say one thing that’s really exciting is our partnership with Nvidia, who’s producing game-changing technology in a lot of different industries. You can see the ripple effect. I think now a lot of other names people will recognize with Microsoft, Meta as of recent, and Google, they are focusing on this branch of partial differential equations. And that’s really what relates with the physics that we’re trying to solve iteratively by solving these PDEs. So, you just look at some of these headlines and tabloids and things like that, as far as what’s happening, non-technically and just see that it does have a relationship to what we’re doing, and it will propagate to us. I think in Nvidia’s recent GTC, they showcased some stuff regarding their digital twin of the earth. I mean, that’s just the coolest thing ever, even if you’re not an engineer, to fight climate change. What a time to be alive and see that sort of stuff. So, we can see that this is not all the different than LAS; LAS came into play with forecasting and weather in large scales and stuff like that. So, you’re seeing really the marrying and overlap of these different technologies. And it’s personally very exciting.
Spencer Acain: Well, that sounds really interesting. And I think that would be a good place to end our talk here today. Justin, I’d like to thank you for joining me here.
Justin Hodges: Yeah, it’s been a pleasure. We could talk more and more on this because there’s so much going on, but hopefully this very small select snippet of stuff that we ended up covering is useful to a bunch of listeners.
Spencer Acain: Absolutely. Once again, I’ve been your host, Spencer Acain, and thank you for listening to the AI Spectrum podcast.
Siemens Digital Industries Software helps organizations of all sizes digitally transform using software, hardware and services from the Siemens Xcelerator business platform. Siemens’ software and the comprehensive digital twin enable companies to optimize their design, engineering and manufacturing processes to turn today’s ideas into the sustainable products of the future. From chips to entire systems, from product to process, across all industries. Siemens Digital Industries Software – Accelerating transformation.