Simplifying Simulation with AI Technology Part 4 – Transcript
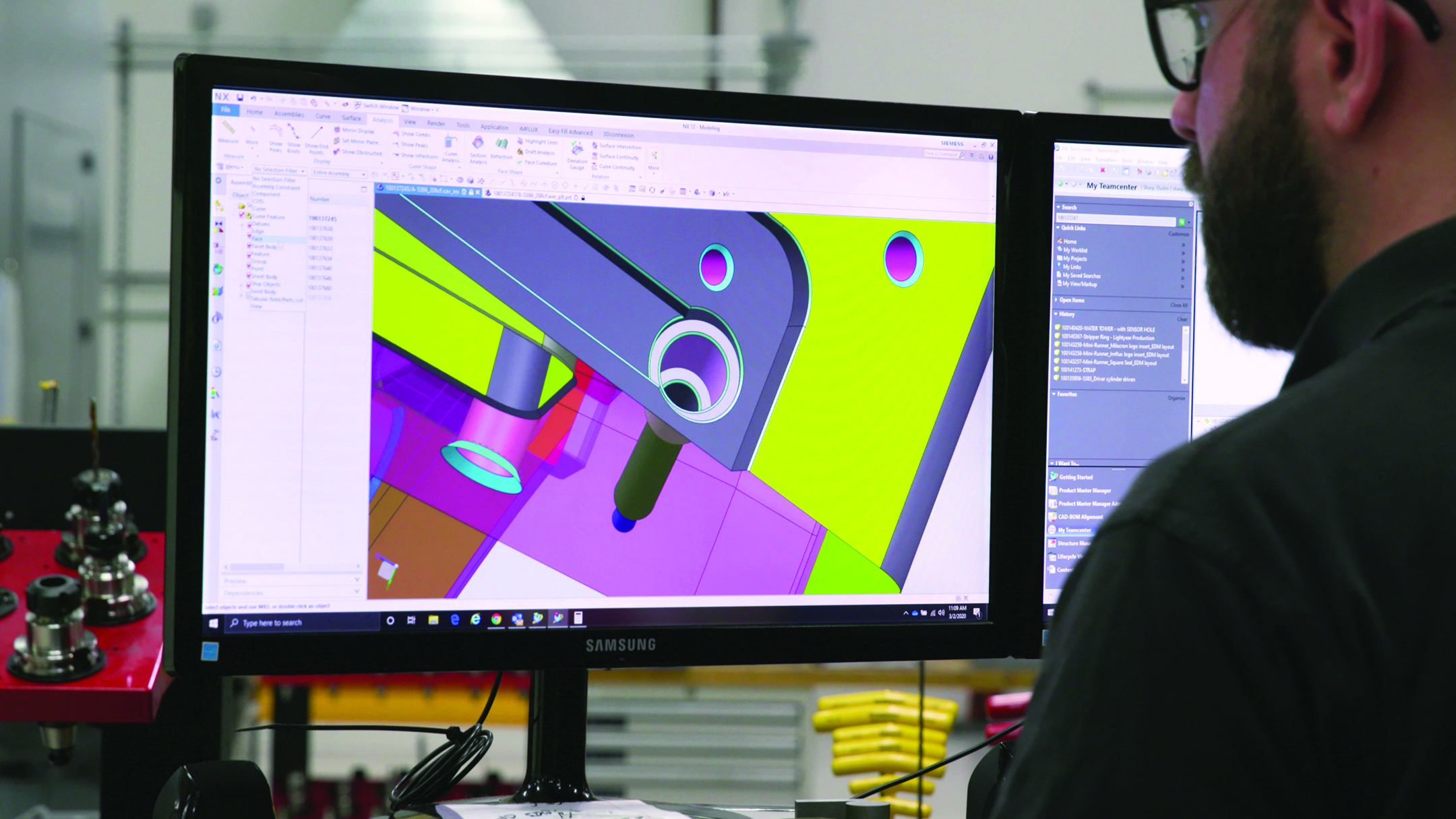
I recently had the opportunity to continue my conversation with Dr. Justin Hodges, an AI/ML Technical Specialist about the ways AI is reshaping the space of physics-based simulation and where he sees the AI space going in the future. Check out the podcast here, or keep reading for the full transcript.
Spencer Acain: Hello and welcome back to the AI Spectrum podcast. I’m your host, Spencer Acain. In this series we explore a wide range of artificial intelligence topics from all across Siemens and how they are applied to different technologies. Today I am once again joined by Dr. Justin Hodges, an AI/ML technical specialist and product manager for Simcenter.
Spencer Acain: Picking up from where we left off last time talking about the way AI is creating new opportunities for interconnectedness and cross-pollination between different tools, which sounds like a major goal you’re working towards, do you have any goals for integration beyond a single suite of tools? Such as other Siemens tools like NX or PlantSIM or even a broader range of tools beyond what we have at Siemens such as open-source tools or even tools from other vendors?
Justin Hodges: Yeah. So, this is where our customers really get a lot of benefit. You know, you can use a lot of open-source tools and a lot of things in the machine learning community and publications and codes have been made open source, which is a tremendously valuable thing. But if you work at a plant, an airplane company and a part has a malfunction, you really want to be less distracted by code manually running things, things like that. You want to focus on, you know the principal area of what you’re trying to do in the tools can enable that, but not distract you too much and as a part of that, you know you can also have variation, different teams work on something in a very hands on the keyboard, like raw coding open-source way in terms of different ways of simulating, setting up solving, etcetera. And you wanna make standards so that everyone can kind of do it the same way, even if they have different levels of experience in the company or in the field. And so that’s where providing these tools makes our customers really better off as we’ve kind of tried to make that less of a factor so that they can be more standardized and things like that. Now to your earlier point regarding different things that this can be done outside of Simcenter tools, the PlantSIM one is a great example.
Justin Hodges: I think it goes back to like classical optimization machine learning problem. Umm, you know, because there’s a lot of decisions in terms of logistics for factory automation and assembly chains and things like that. There’s so many variables and possibilities that exceeds even in simulation and design, like how many variables we usually have and options of permutations of different designs we can have, you know, goes back to like classical problem for machine learning or simple example of like, you know, I wanna go on vacation and hit like 5 countries this really mega vacation, right? And as far as every decision goes, I wanna make it optimal. You know the best food in every airport I stop, you know, I wanna sit in this range of the seats which obviously implicates the cost and maybe I have fixed budget. I don’t want any delays. I wanna stay in certain places longer. I wanna even be as granular as like what type of excursions do I like and stuff like that and so you have like basically unlimited number of options right? And as you quickly find out it’s a great optimization and machine learning problem because it’s open-ended and there’s so many possibilities. And so, I think that this has broad implications across Simcenter and well beyond Simcenter and yeah, you’re right, Siemens has a lot of tools outside of Simcenter and basically for everything you could want to do. So, I would say just at large has a very large impact for a lot of industries and one of the things that we bring as a provider of the software and services is, you know the standardization lowering the bar of entry so people want to be experts to use these sort of methods. Or maybe it doesn’t eat up a bunch of schedule time to set it up. So that’s something I think that we really are powerful at Siemens.
Spencer Acain: That’s great. I mean, it sounds really helpful to be able to just kind of have everything work together, all the standards are standardized and so that you don’t have to be an AI expert to use an AI tool.
Justin Hodges: Yeah, that’s the major persona. You know, people are very smart and but you know, there’s only so many so much time in the day to focus on at work as far as your expertise goes. And it’d be great to incorporate and leverage a lot of methods without having to, like, have done your PhD on it. So the more we can infuse in their tool belt, the better.
Spencer Acain: Kind of like to change tacks here a little bit and move back to the realm of simulation for a little while here, kind of. I’d like to build on the idea of putting AI in simulation, but how do you see AI changing the landscape of existing physics-based simulations, such as through the use of physics informed neural networks and other similar technologies?
Justin Hodges: Yeah. Well, I think everyone’s favorite theorem to quote, that’s from engineering school is no free lunch theorem, right? So I think there’s no absolute win without any cost and physics informed neural networks have immense potential. They’ve generated an extreme, you know, amount of innovations in the field but it’s still evolving and growing to be more and more usable by industry, and it’s something we’re heavily looking at and testing and working with. So, it’s identified as something important for sure without a doubt. But you know is it industry ready? Depends on the problem and that sort of things. But there’s really a good place for it because in one sense you have simulations that provide data and machine learning models that take that as the absolute truth and make conjecture and predictions from that and that’s not the whole story, right? You definitely would want other ways of making a pipeline of machine learning, where perhaps it doesn’t rely on imperfect simulation data to treat it as 100% true all the time. And you do have these physics based and physics informed neural networks, types, approaches. And it is very revolutionary to the field, that’s for sure. It helps with possibly reducing the data requirements for not needing as many simulations to train. Yeah. So I think it’s, I think it’s really powerful field, you know and we’re working on it cause it’s something that could definitely be a game changer in the technology.
Spencer Acain: Yeah, I mean, I could see that. I mean, if you can reduce the amount of data you need for training and stuff, but it does sound I’m kind of reading here between the lines, maybe a little, but maybe there are some challenges with this approach like you having some that it’s not ready maybe ready for the mainstream quite ready to be adopted everywhere yet. Can you maybe talk a little about these challenges for us like what maybe we’re is holding back this PINNs from just being used everywhere, for example.
Justin Hodges: Right, right. So I mean, it depends on the problem to address the specific challenges, the challenges associated with it. But I think that one of the end goals is how general your machine learning model can be. I think that’s like even in a technical sense like a tradeoff, when you train a model like how specific it is to learning your individual problem or your space and thus low amounts of error, it would be present because you’re making your model highly tuned to that problem versus a very, very general model that can make predictions under a vast amount of scenarios and designs, in which case maybe you have, you know, higher losses when you train and stuff like that because you’re really trying to make a general so that in the cases that you use it going forward, you know it’s accurate. So I don’t know, I mean, there’s definitely work to be done in making it industry ready and scalable for big problems and industrial problems with complex geometries and complex physics and stuff like that. But there are places where it’s successful and essentially our goal is to keep it in motion and working on it and evolving with things in academia on this topic so it can be used more in industry when it’s ready. But I guess a key factor would be speed and complexity, as you increase the complexity you know, the speed drops off dramatically when using these sort of models but then visa versa on more simple problems it’s better. But as you know, industry tends to view very complex things to have as realistic of a simulation as possible. But it’s a key theme. you know, we wanna have faster solving and faster insights for the customer and that sort of thing. So, you know, it’s definitely a big pillar in what we’re looking at.
Spencer Acain: Well thank you for giving me that answer. It’s very informative. So, I think we’ve talked a little bit now about physics informed neural networks and of course a few of the challenges. But what are some of the major benefits, some of the areas that would benefit most from Like AI and ML entering into that space with them like, you know, maybe beyond physics. Just in general or?
Justin Hodges: Yeah, there’s a few. There’s a few big things that you could consider using machine learning for in your simulation product, or your physical measurement based product or Simcenter. All variety of tools. I think it has a pretty universal impact and probably to an extent on several of these like outside of just engineering. But the other, the only time I talked about so far is like faster predictions, faster insights and things like that, you know, accelerating traditional methods so that our customers can get answers faster. That’s certainly one is speed. The other one I would say is convenience for the user. You know, if I can get from my simulation and extremely accurate answer and I don’t need more accuracy out of my method, but it takes days and days and days to set up. Well, that’s, you know, not a perfect story. As we mentioned before, it is time to market and time to generate simulations is a key consideration. So, you know our customers certainly require innovation on how fast we can do things and how much work they have to do in setting up their simulations. And you see this all the time for like workflow systems and recommender systems, I mean you can see this well outside of the software for engineering. You know very, very intuitive layers laid over, you know, legacy software, machine learning features so that it’s more intuitive, easier to use, less click miles, stuff like that. And there’s really a lot of other themes probably, but I could just say a big other one is complexity, you know, and this is kind of an intuitive thing about machine learning is it can provide insights that you otherwise wouldn’t have available to you. So, we have this software, we have this ability to model physics but having these machine learning methods in our toolbelt definitely increases the complexity that we’re able to ascertain and to capture and harness with our design insights and usage and stuff like that. You know, for example, I think a really popular field is anomaly detection for machine learning, like physical sensor data and stuff like that. And so, you know, if I put like 30 analog signals in front of you in real time, recording data for whatever device you want, it doesn’t matter some instrumentation. I mean, it’s me very hard for a human to understand literally anything while looking at the time series evolve.
Justin Hodges: So you know it does add, you know, understanding and comprehension when you have these machine learning models that can quickly, you know, deduce red flags that show up and operator data and stuff like that. And then like, alert the user to do something to prevent equipment damage or bad performance and stuff like that. So, there’s definitely a big element of new insights that you can have with machine learning.
Spencer Acain: That sounds like a fantastic addition to any tool belt, really, in simulation, and even just beyond simulation. I guess as a final question for you, before we wrap this up, I’d like to ask where do you see this technology going in the future? You know, as AI is starting to become more interconnected integrated at every level of product design and testing and even usage, what are some of the coolest innovations in the technology that you see coming out in the near future or even a little beyond that?
Justin Hodges: Yeah, that’s a fun question. There’s a few things, but even on those I think you know I’m thinking pretty small as we tend to do as humans on you know, thinking outside of what we’re used to. But one thing that was pretty cool was long time ago, maybe, I don’t know, whenever the iPhone came out long time ago, I was at a Turbo machinery conference and had nothing to do with iPhones or that sort of thing. But the President of this huge power plant energy supplier company, Duke Energy, was speaking as the keynote and he basically said that in the way they conduct their business. One of the biggest things that changed them over that timeframe of the iPhone came out was the iPhone, because it changed, you know, the level of expectation and convenience and, you know, Elon Musk likes to say basically turns you into a Cyborg in terms of being able to access vast amounts of information very quickly and do things with it, you know, beyond your human input output. So a few years before the iPhone, it’s like predicting how that would have an impact on everything. So it’s a bit like that, you know, it’s super hard, but we try to think big, right? So I would say one thing as far as the future is definitely knowledge, knowledge base and system information, you know, so you have so many products, so many different amounts of data that customers produce for different tests, you know, basically every large company has, you know, multi country locations. So, there’s so many factors that go into all the data that’s generated all the data that’s stored all the data that’s made use I think that number gets like super small each time when you go from all the data produced to all the data that’s used. And so I think that there’s a lot of ways machine learning can be implemented to try to fix that. And thanks to our big, you know, tech company overlords like Amazon and Google and Netflix and Facebook. You know, you’ve seen these huge innovations on cloud-based things and you know, database management and systems engineering to provide an infrastructure so this data can be communicated back and forth. And I hope that engineering communities keep building that proficiency up and matching that and then implementing machine learning so that it’s very, very easy for people to do their daily work and then just have stuff appears sort of as knowledge to them. Like what you’re about to do is 92% waste of time because and then points to other work that was done in the past, I say. Oh. OK, great. You know, let me not spend $10,000 worth of resource on that, you know, sort of study you know. So, I think that’s a big thing. You know we’ve been generating a lot of data for a long time. I hope we can better use it going forward and then in a silly way you know I love NLP to be incorporated in what we do. So, I could you know write emails and then just tell my tell my CAE software to do things and it would do things on voice command so I don’t have to do it and stop what I’m doing otherwise.
Spencer Acain: That would be pretty awesome. I mean, to me that’s just sounds like something out of Star Trek to say computer make me make me something. Make me like, a computer. Make me like a tricorder or something. And then it just does. It don’t have to think about it. It just knows what you want. It takes all that gather data from generations and just makes it for you.
Justin Hodges: Yeah, there’s definitely been some exciting relations. So we’ll see what comes next. As I mentioned in the beginning, you know there’s no shortage of money moving in this direction, people working on it from research point of view or, you know, people incorporating it into what they do in industry. So I think it’s just the perfect recipe for an exciting future. No doubt about it.
Spencer Acain: I couldn’t agree more, but and it’s been a pleasure talking to you and I think we’re pretty much out of time here. So, Justin, thank you for joining me here and giving your wonderful insights on AI and where it’s going in the future.
Justin Hodges: My pleasure. Nice discussion. We’ll do it again.
Spencer Acain: Absolutely. Once again, my I’ve been your host, Spencer Acain, and this has been the AI Spectrum podcast. Thank you for tuning in and be sure to catch our next episode.
Siemens Digital Industries Software helps organizations of all sizes digitally transform using software, hardware and services from the Siemens Xcelerator business platform. Siemens’ software and the comprehensive digital twin enable companies to optimize their design, engineering and manufacturing processes to turn today’s ideas into the sustainable products of the future. From chips to entire systems, from product to process, across all industries. Siemens Digital Industries Software – Accelerating transformation.