How AI Revolutionized IC Validation and Characterization – Transcript
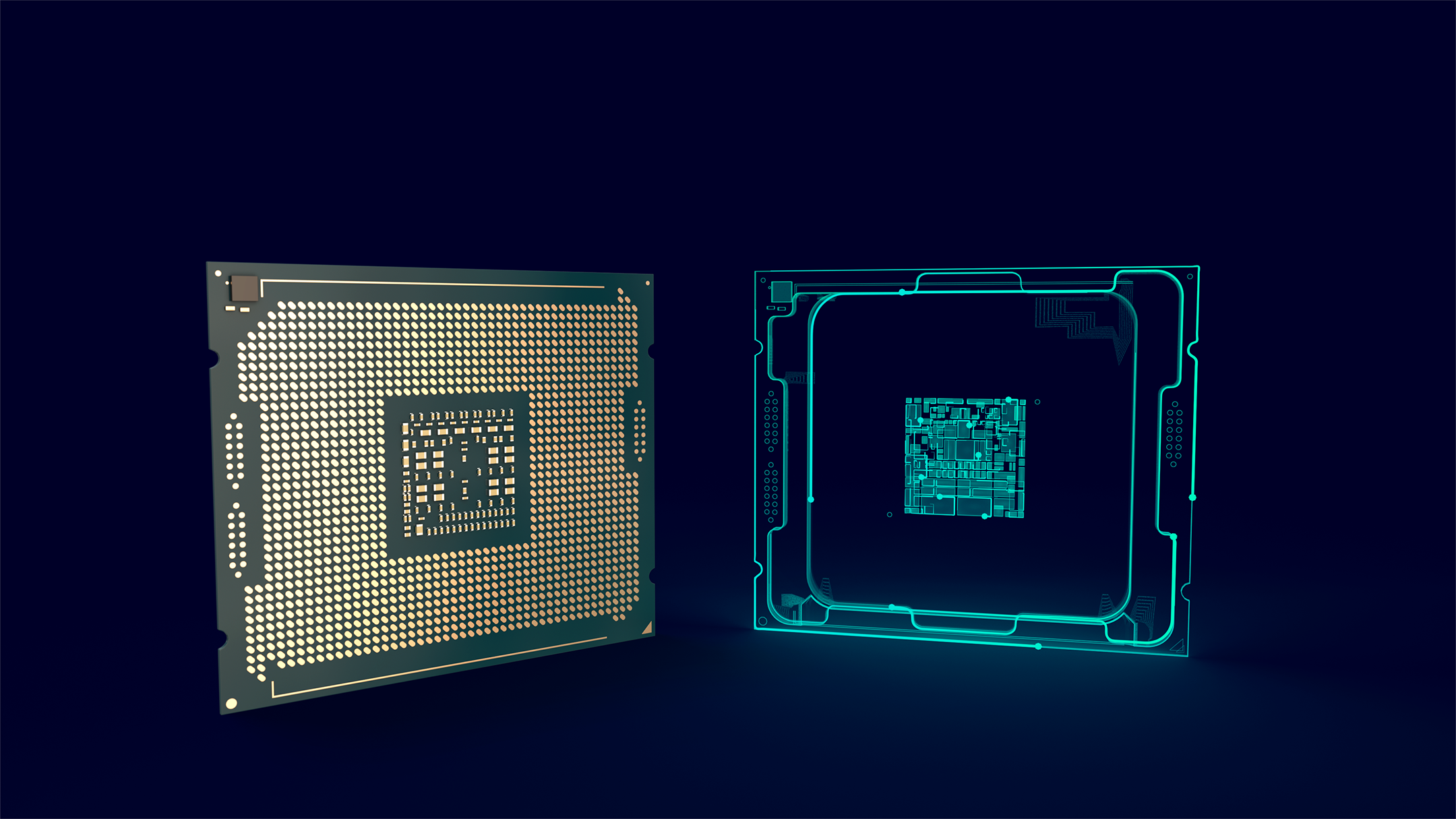
Integrated circuits and chips are among the most complex and expensive to manufacture devices in the world and, despite their small size, are comprised of billions of components. Validating these devices before they are deployed to larger projects is vital but exceedingly challenging. In a recent podcast, I spoke with Amit Gupta of Siemens EDA about how he and his team used AI to revolutionize that exact process and how he was able to drive industry adoption of this ground breaking technology nearly 20 years ago. Check out the podcast here or keep reading for the full transcript.
Spencer Acain: Hello and welcome to the AI Spectrum podcast. I’m your host, Spencer Acain. In this series, we talked to experts all across Siemens about a wide range of AI topics and how they apply to different technologies. Today I am joined by Amit Gupta, founder and president of Solido Design Automation before its acquisition by Siemens EDA in 2017. Amit continues to work for Siemens EDA, leading the growth of Solido’s machine learning enabled capabilities. Welcome, Amit.
Amit Gupta: Thank you.
Spencer Acain: So, before we jump into this, can you tell us a little bit about your background and your entrepreneurial work as well as your current role at Siemens EDA?
Amit Gupta: Sure. Yeah. So, I’ve been in the EDA industry, so developing software for the automation of semiconductor design for the last 20 years or so, I had a startup called Analog Design Automation that got acquired in 2004. And then Solido Design Automation I started in 2005 really focused on using machine learning and artificial intelligence algorithms to improve the power, performance and area of electronic chips, so started the company in 2005. Really did a lot of R&D focused on developing patents and then rolling it out to industry and we had a lot of success and Siemens acquired the company in 2017. So now I’m general manager of the Solido products inside of Siemens and we’re with the Siemens reach, we’re now developing and releasing it out to, you know, a lot more customers in the last four or five years. So really enjoying that role.
Spencer Acain: Yeah, I mean, it sounds like you’ve had a pretty exciting career up till now and could you tell me about, you know you mentioned Solido which is what you currently working on. Could you tell me more about that, especially how you guys are using AI or machine learning in that?
Amit Gupta: The focus that we have is for a specific types of circuits that are done in semiconductor design. So, things like the memory blocks within a semiconductor chip or the analog blocks or the standard cell library blocks. What we do with our machine learning tools is enable our customers who are the designers at the semiconductor companies enable them to get much, much more coverage of their design, so being able to measure the performance of the design, measure the power that their design is consuming, be able to get a lot more coverage of that design in much fewer simulations and what would be required otherwise. So, we’re able to, for example, if they want to verify their design to Six Sigma to make sure that there is a only a one in a billion failure rate, what would traditionally take 6 or 7 billion simulations, which is would take weeks or months to do. That’s not even a realistic thing to do when using our software with our machine learning technology, they’re able to get that same level of coverage in only a few thousand simulations. So reducing from billions to thousands of simulations and what that means for designers as a result is that they are able to get the optimal power performance area and yield for their designs. They’re not over designing, so leaving a bunch of power performance area on the table and they’re not under designing, so leaving a bunch of yield on the table, but they’re getting the full coverage of their design. So huge value from the machine learning algorithms that we’ve developed in our software.
Spencer Acain: Okay, I mean that sounds absolutely kind of incredible really you’re taking literally hundreds of like hundreds of orders of magnitude, but 100 times faster or more like hundreds or thousands of times to do these simulations, like how are you able to achieve that kind of like reduction going, you know from billions of simulations down to what these had like thousands?
Amit Gupta: Yeah, billions thousands. So it’s like a, you know, million X reduction in in. Yeah, number of simulations. And the way we do that really is utilizing the power of machine learning, where the software automatically runs a certain number of simulations to get its training data and then the training data is used to build machine learning models and then these machine learning models are able to predict how the design will do at the extreme conditions, so how will it do at you know that Six Sigma condition that one in a billion level condition using the machine learning models that were built on this training data. So that’s really the magic of using machine learning as opposed to not machine learning you’re doing it in a very brute force way using Monte Carlo analysis and brute force running those billion simulations to see how things are performing and as a result of that, there’s a lot of wasted simulation you’re not taking advantage of learning from the simulations that you’ve done to in order to inform and guide what the next simulations should be so that you can minimize the number of total simulations that you’re doing. So that’s really the value and the power of machine learning.
Spencer Acain: I see, so it sounds like you’re actually, you’re really bringing like the learning into this the like the machine learning aspect so that you’re not wasting a lot of the effort you would be putting into this, just doing it manually.
Amit Gupta: Exactly. So manually, you’re just you’re there’s no learning involved, you’re just brute force doing everything as opposed to our software, which is learning from each simulation so it can intelligently do the next simulation build up that machine learning model and do it and as a result, be able to complete the entire run in that few thousand simulations as opposed to the few billion simulations.
Spencer Acain: Wow that sounds like it would be just a hugely beneficial and especially now with how big modern chips are getting like with that like, how does this technology extend to that? I mean, I know you’ve been working on it for a long time, so how is it handling modern chips, billions or 10s of billions of transistors even?
Amit Gupta: So, So what, happening in the chip design world is there’s mores law where every 18 months or so, the size of the transistor shrink by ½, by 50%. So as a result, what happens is because of that shrinking of the transistors, the amount of variability and how those transistors actually perform when they are manufactured, it goes up and up. So, for example, when we first started the Solido as a standalone company in 2005 designers were designing at 130 nanometer transistor sizes or 90 nanometer transistor sizes. Today the transistor sizes are down to 7 nanometers, 5 nanometers and 3 nanometers. So, all these most advanced chips, the transistor sizes have gone down dramatically. So as a result having those chips work is becoming more and more of a challenge, so there needs to be more and more of the simulation coverage at the transistor level to make sure that the designs are working. And that’s so the value of our software is increasing. I saw publicly in an article yesterday that a 3 nanometer wafer from a foundry, now it’s costing about $20,000 so getting the most power performance area and yield from that $20,000 wafer at 5 or 3 nanometers is really, really, really important.
Spencer Acain: Yeah, I’ll bet. I mean, getting that like, how do you? I can’t even begin to conceive of how you’d handle, like, looking at analyzing chips, something that’s small like nanometers like that, with billions of them. It’s just incredible.
Amit Gupta: What actually kind of lends itself to machine learning is because when you have, you know, such large circuit sizes with a lot of transistors and each transistor could have seven or eight or nine different variables. So, you know, say 8 variables per transistor times even millions of millions of transistors you’re talking about, you know, 8 million 10s of millions of combinations. So as a result of that, there’s a lot of data. So, it really lends itself to artificial intelligence, where we can produce a lot of data to train these models to be able to do prediction on how the circuit will be performing at these extreme conditions. So, the fact that you know the problem size is so large creates the fact that there’s these large data sets that allows us to mine these data sets and produce this kind of this useful information and this useful prediction to designers.
Spencer Acain: So, it almost sounds like AI and ML was just kind of a natural step forward for the for you guys because you have such a naturally data rich problems that are environment you’re working in.
Amit Gupta: Exactly. And that’s kind of what steered that steered us towards that area back in 2005 when you know there wasn’t a lot of public buzz or talk about machine learning at the time it was, there was a lot of work in research still, but not a lot of discussion and industry about it. So, I think we were really one of the first EDA companies that looked at how can we apply machine learning to the semiconductor design space and we saw, you know, it’s a really, you know, the problem domain is really conducive to machine learning. There’s a lot of acceleration that can be done beyond some of the traditional brute force methods that were being done previous to us coming into the industry. So that’s why, you know, we thought machine learning plus semiconductor design, you know it’s a great fit and we’ve proven that out.
Spencer Acain: It sounds like you’re wildly successful with these sort of tools and a for many companies and a lot of industries and even though you were definitely one of the earliest adopters, like all the way back in 2005, I think you said you were already using this sort of AI and ML technology. When I don’t even think a lot of other industries were considering it at that point, but like you also said that like these wafers are extremely expensive and these processes are very expensive. So, was there like, was there some difficulty in getting adoption of this sort of new unproven technology in such an expensive and like potentially risky area where you could really be stand to lose a lot of money if you get it wrong?
Amit Gupta: You’re exactly right. What I think is unique about applying machine learning for engineering applications in general, not just for semiconductor design but for engineering applications is you know you don’t want the chip to fail. You don’t want the bridge to fall down, so you have to do much, much better than guessing or, you know, having ± 10% error. You need to do much, much better than that. So what we’ve really done is develop our own algorithms that are brute force accurate. So having that brute force level of accuracy is extremely important for engineering applications given the nature of the domain and given the fact that designers and companies are signing off on these on the results of these tools. So, we needed to make sure that the algorithms are robust to you know variety of corner conditions. So, it’s it wasn’t just a, you know, a few months or one year project. This was a multiyear investment that we put in to make sure that the algorithms are accurate across all the different process conditions and transistor sizes and so on, and the other part of it too is the algorithms needed to give visibility to the designers as to the fact that it was converging, that it is converging on a solution and the results are correct so that designers are seeing it’s not a black box where they put in some data and something gets spit out, but they can see the algorithms working and they can see it converging on solutions and why it’s coming up with the results that it is. So it really builds trust among the designers as to why the tool is producing the results it does and then designers can stand up and design reviews and defend the results that are that are provided. The other thing that we really focused on is the user experience and the user interface in the tools. We didn’t want designers to have to become machine learning experts. They know design well and we had to make sure that the user interfaces such that it’s all within the design domain but the algorithmic details are all taken care of automatically under the hood and not exposed to the to the designers, unless they’re power users. So, there is a lot of those kind of considerations in terms of accuracy of the results, verifiability of the results, usability of the results so beyond you know, speed up where we need to provide a at least a 10X improvement in speed or accuracy of what they were doing without our software we needed to have that that accuracy, verifiability and usability type attributes in the software, so that took a you know that’s a quite a while to build out, several years, but now we’re at the point where we have thousands of users using our software around the world. Most of the top semiconductor companies and foundries and system companies all use our software in their sign off flow and it’s all very well trusted. But it was definitely a journey to get it to this level of maturity such that it’s trusted by the mainstream, by the mainstream market.
Spencer Acain: Yeah, I mean, I can imagine I’ve had the opportunity to speak with some experts over from NX and the Simcenter side of things and I know that they’ve been struggling with this as well getting the building that user trust in the results and letting them see that the data that what the machine learning algorithms are spitting out is accurate to what they need and safe enough to use. But it sounds like you’ve been tackling that for a long time and you’re actually had a very mature spot compared to a lot of other of groups or a lot of other software that are at a kind of still in the early adopter stage even.
Amit Gupta: I think the way developers of machine learning solutions for various engineering applications you know need to look at it as is beyond that like I was saying beyond the speed attribute looking at how do we get brute force level accuracy, how do we give visibility to the designers such that they can verify that the results are accurate? How do we make the user experience such that there that the designers don’t need to become machine learning experts. You know those are all things that need to be codesigned with the speed performance boosting in order to get mainstream adoption and get it out of, you know, one or two people using it in an organization to getting it to hundreds of people, thousands of people in a in an organization using it. I think, I mean I think the other, the other big thing is as there are success stories and companies you know, start seeing value, being able to showcase those kinds of success stories so that other companies in the industry can benefit from the from what those companies have achieved. I think that’s really important. So, we have a number of success stories with customers. We published one a few months ago with ARM talking about how ARM was able to use our verification solution for their designs and how it provided 1000x speed up to their to their design. So having those kind of yeah, having those kind of examples, I think really give credibility to the to the machine learning solutions and get it out of being like a science project to being a production solution.
Spencer Acain: So things kind of just snowball once you can start to show some real benefit that works and have a few people who have adopted it and then you can really take it from there and it just starts to grow naturally, as people start to trust by large right group at large.
Amit Gupta: I mean, I think I think our experiences you know there is growth, but it also takes a lot of a lot of effort. So we have a I mean we have an applications engineering team that works really closely with customers and user by user to make sure that they’re using the software really well and they can see they’re able to generate the value of the software on their design. So I think you know, with any kind of engineering type software having a having a really good applications engineering team is important for a successful rollout of product but yeah, I think combination of you know excellent algorithms with excellent user interface with an excellent implementation and support team. I think that that’s kind of the recipe for getting these kinds of tools out of the market and broadly adopted.
Spencer Acain: Yeah. I mean, it sounds like you guys have really invested very heavily in AI and have been for more than a decade now, maybe even close to 20 years. But so I’d really like to wrap things up here by going into a little more detail on just exactly what Solido was doing with AI and ML. Can you just give me the just the hard facts here, so to speak?
Amit Gupta: Yeah. So we have we have two product families, specifically Solido Variation Designer and the Solido Characterization Suite that incorporates machine learning algorithms to provide the improved design performance in fewer simulations then brute force. Solido Variation Designer focuses on variation aware design for analog type cells, memory cells, standard cell cells where we are able to improve the power performance area and yield of the designs by giving more coverage and fewer simulations than brute force. And then the Solido Characterization Suite focuses specifically on library cells. So standard cell library elements, memory cell library elements in order to more quickly generate what’s called liberty files, which are characterization files of how the cells work and also provide better quality of those of those cells. Again, both accelerated using machine learning and we’re doing some exciting things that I can’t announce right now, but in our road map that’s coming out over the next over the next year or so to provide even more benefit to our users. So really excited about not just what we’ve what we’ve released and out in production but some of the new innovation that our R&D team is doing that will provide even more benefits to our users.
Spencer Acain: Well, that sounds pretty incredible overall that you’ve got a very mature suite of software here that really delves into, that really showcases just how powerful AI and ML can be in the engineering space and the testing design verification, all of that.
Amit Gupta: Yeah, absolutely. It’s, it’s exciting. And I think the other part of it is being inside of Siemens now our scope is a lot larger than what we we’re where we were at with as a startup in Solido where now we see other tools and opportunities to apply machine learning in other tools within the semiconductor space and then beyond the semiconductor space, into digital industry software, everything that the other teams within Siemens is doing there’s a lot of opportunity for collaboration that we’re really excited about.
Spencer Acain: I look forward to hearing more about that at some point. But I think we are just about out of time here. So Amit, thank you for joining me here. It’s been a pleasure talking to you.
Amit Gupta: Yes, that it’s been great. Thank you very much.
Spencer Acain: Once again, I have been your host, Spencer Acain, and this is the AI Spectrum podcast.
Siemens Digital Industries Software helps organizations of all sizes digitally transform using software, hardware and services from the Siemens Xcelerator business platform. Siemens’ software and the comprehensive digital twin enable companies to optimize their design, engineering and manufacturing processes to turn today’s ideas into the sustainable products of the future. From chips to entire systems, from product to process, across all industries. Siemens Digital Industries Software – Accelerating transformation.