How generative AI is augmenting the engineering process
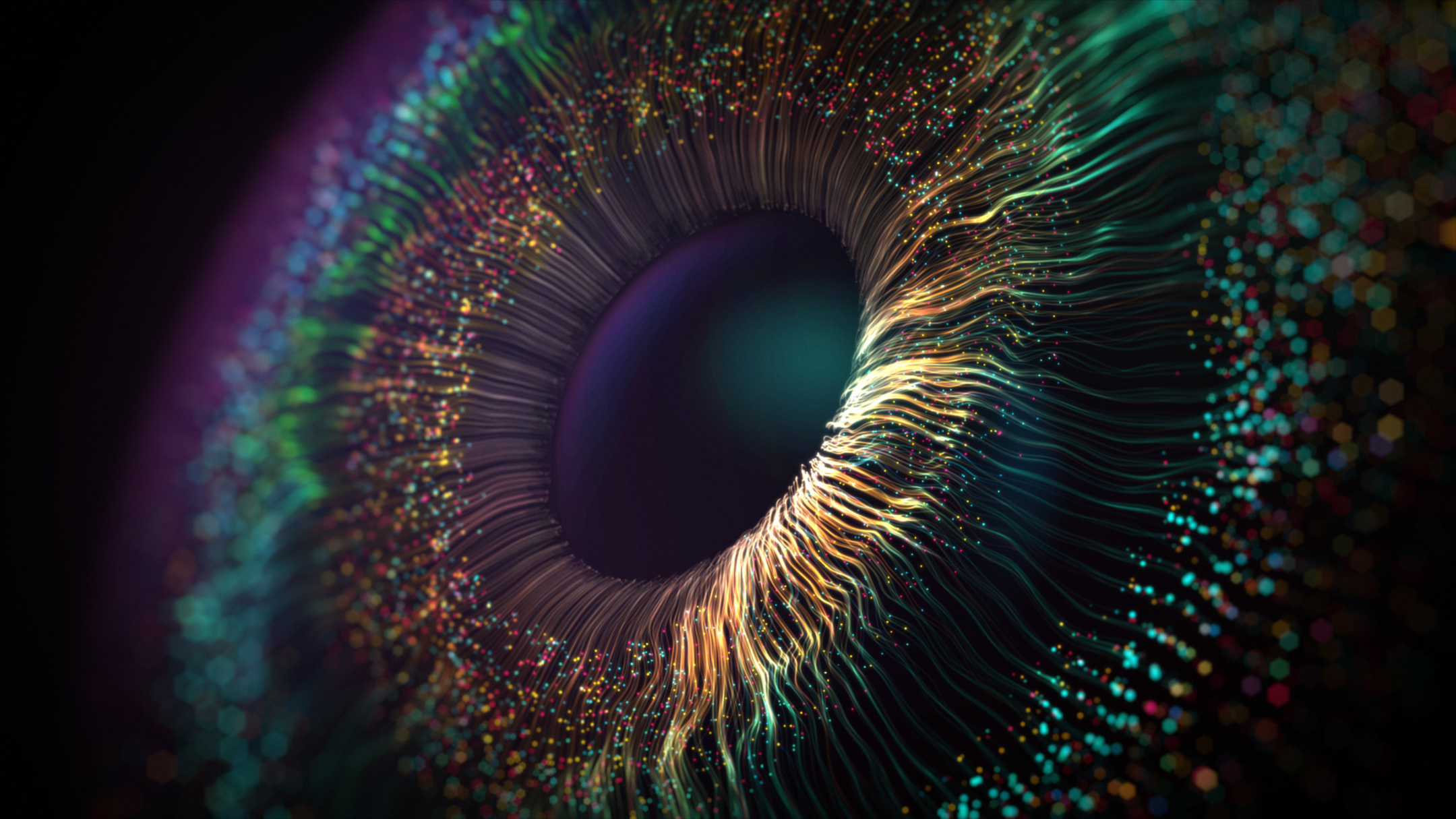
In a recent podcast I discussed some of the ways generative AI is poised to impact the engineering, design and simulation space with its ability automate mundane tasks while also improving the accessibility of information. The benefits of generative AI, however, do not stop there. In this second episode, Dr. Justin Hodges continues to expand on potential applications of generative AI, specifically how it enables wider design space exploration and supports a strong requirement driven engineering process.
These new generative AI-driven elements could also serve to enhance and enable generative engineering processes. To learn more about generative AI, generative engineering, and the difference between them check out this blog. The full episode of our talk can be found here or keep reading for some of the highlights of that conversation.
To start things off Justin proposes the idea of reverse or inverse engineering; that is the rather than creating models, adjusting parameters and then running tests to find out how a given design performs instead starting with a set of requirements and working “in reverse” to arrive at an optimized design. While the idea is a simple one, actually executing on it is anything but. For a human, it’s simply impossible to work backward using vast quantities of historical data as a guide to arrive at an optimal solution given a set of requirements.
The forward moving iterative design process is thus developed out of necessity since it is compatible with the way humans create and develop ideas. However, for a generative AI model, it is comparatively easy to comprehend the entire breadth of available design data and, using that as a guide, develop an optimized solution to perfectly match a given set of criteria.
Building an AI model capable of performing requirement-driven, inverse engineering requires vast quantities of data which is often gathered from running simulations on existing designs during the design space exploration step. This is where tools like HEEDS, part of Siemens Xcelerator, are vitally important. A tool like HEEDS allows for semi-autonomous design space exploration and uses integrated non-generative AI and machine learning capabilities to help traverse the design space while also, crucially, helping highlight the areas of data shortfall where further simulations are required.
Generative AI-based data augmentation can serve as a powerful tool to address these potential short falls in existing data. While this synthetic simulation data cannot full take the place of running full simulations, it can bridge the gap between known data points while exploring a design space, offering a high-fidelity approximation where previously time wouldn’t allow for a simulation to be run at all. Since time is one of the major limiting factors when it comes to design space exploration, having the ability to reduce the amount of time spent running slow, computationally expensive simulations without sacrificing quality is a key element in searching for the best possible designs.
When it comes to generative AI, Justin doesn’t see it as something that will replace humans or existing processes, rather he sees it as an aid or a supplement, working alongside designers or sitting atop existing tools to enhance their functionality. In order to get the most out of generative AI in simulation and design, it will be important to develop a connected tool chain, where different tools can connect and work together to feed data into each other and into generative models. This cross flow of information between tools and disciplines is not only vital for the development of generative AI models in design but serves to benefit the design process as well.
Generative AI is a powerful tool which has the potential to bring about a paradigm shift in the way data is used, stored, and thought about, enabling designers to not just work more quickly and easily, but across a broader scope as well. Vast quantities of historical design and simulation data are available to help guide the path of future designs and now, with generative AI, it’s easier than ever to turn that data in to a tangible benefit. In turn, this enables the exploration of a wider design space, offering new and revolutionary ideas to support the demand for smarter more sustainable products delivered in record time.
Siemens Digital Industries Software helps organizations of all sizes digitally transform using software, hardware and services from the Siemens Xcelerator business platform. Siemens’ software and the comprehensive digital twin enable companies to optimize their design, engineering and manufacturing processes to turn today’s ideas into the sustainable products of the future. From chips to entire systems, from product to process, across all industries. Siemens Digital Industries Software – Accelerating transformation.