Talking Aerospace Today – AI in Aerospace Part Three – Transcript
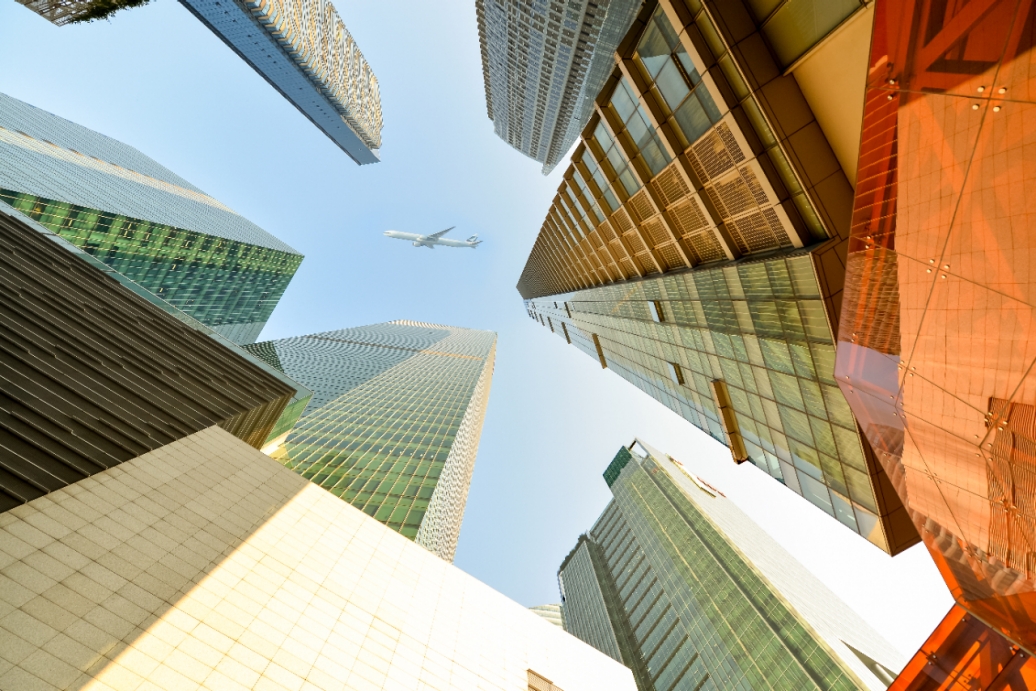
In the latest episode of Talking Aerospace Today, Todd Tuthill and Justin Hodges wrap up their conversation on AI in digital transformation maturity and the A&D industry, discussing how confidence in AI can be increased and future applications in the industry.
Patty Russo: Greetings, and welcome to another episode of Talking Aerospace Today from Siemens Digital Industries Software. I’m Patty Russo, and today once again I am joined by our VP of Aerospace and Defense, Todd Tuthill. We are also joined again by Justin Hodges, AI/ML technical specialist and project manager for Siemens Simcenter. Today we’ll be wrapping up our conversation from the past two episodes on AI in the context of digital transformation maturity. We’ve covered a lot in the previous episodes, from what AI/ML is and why the A&D industry needs to begin to recognize it and find ways to adopt it, to how it could improve engineering processes and the perception of the industry as a whole. There is still plenty to cover, so let’s get this conversation going again.
Patty Russo: So you both touched on a couple of things in your answers there that I want to drill down on just a little bit. Justin, you mentioned the idea that there’s open source, manuscripts in open source, and then, Todd, you talked about IP in the tools. And as we know, many of our different industries are doing cloud-based applications for a lot of their companies’ tools. And one of the things that comes up anytime, especially in aerospace and defense, anytime we talk about cloud, anytime we talk about IP, anytime we talk about open source is the idea of trust and of security, safety constraints around intellectual property and around their data. How can people in the industry learn to trust AI? What are some of your thoughts in regards to where that may impact the adoption of this type of a solution?
Justin Hodges: Yeah, it’s an important question. I think it’s also a bit philosophical, but true to zoom out and say AI simulation, adjoint optimization, I mean it could be any new method that emerges, there is this process for adoption betting validation and then people basically, you know, incorporating it into the standard tool set to be used, and I think that to a large extent it’s just another example of that in history is now AI. I think that when you commented on open source and contrast to the data privacy concern, to think it’s great in terms of setup because some of these state of the art language models for example, are completely offered open source for people to take and then use and fine tune to their application in, in a private way.
Justin Hodges: So it’s a great start because we can take advantage of these advancements that seem to be happening in AI on a weekly or monthly basis, right? To keep up with the cadence of how much these things are, how often these things are created. But then to get to the point of answering your question, I think there’s a lot of things to say. Well, how can we trust machine learning in our adoption? But probably the big three would be explainability and bias and then user education. So you know, one want to start with on in terms of explainability would be that, of course, reach researchers are working hard to, you know provide less of a black box when they use machine learning models. More of a semi opaque or semitransparent box.
Justin Hodges: And the hope is that it can shed more light on uncertainty. It can shed more light on why decisions were made or why predictions were made versus others. Potential areas for sensitivities, things like that. So that provides you some context in the prediction on how much to trust it. In terms of bias, I mean the name of the game is bias and generality for machine learning. Bias could be the models tendency to give certain predictions or do well in certain cases, but bad in others. And I think that of course practitioners are working to mitigate bias and identify bias, so that no discrimination, discriminatory patterns and things like that are included in the models that would tend to sway people into the wrong direction.
Justin Hodges: So of course reducing bias is important, and then you know, ever you listen around this podcast is probably able to relate to the last one on user education. You know, I think in the last however many months since ChatGPT came out based on when you listening to the podcast, I think people in society have quickly got up to knowing, you know, kind of how to talk to the model. What’s a good response? What’s a bad response? What type of question you can trust versus not? Kind of how to sequence your questions to get to an answer. It’s kind of a silly example, but it’s also very true at the heart of the of the example, which is, you know, the education on the user side is important.
Justin Hodges: Going in to have some minimum level of understanding of how to use it and things like that in order to set yourself up for safe and good predictions, or I would say inferences when you use the model and get a response. So those are the big three out point out, but obviously there’s more.
Todd Tuthill: Justin, some great points, and I want to pick up where exactly where you talked about because I think that’s true. Any kind of new technology is at first not trusted. One of my favorite movies because I love space and biographies and documentary kind of movies is Hidden Figures. You probably a lot of you have seen it. It’s about this group of women back in the very early Mercury and Apollo programs that did all the calculations by hand. And there’s this great scene before the very first launch, before John Glenn is going to launch, and they’re talking about the coordinates and the computer calculates it, and John Glenn says, well, you know, “I’m not going to trust this. Tell that lady I met-” I think her name was Johnson? I forget her name but, “-until that lady recomputes it for me manually.” She does it and he trusts it.
Todd Tuthill: And that’s a great example because today we just take it for granted that when computers do calculations, we can trust them, but we got to remember that wasn’t always true. That’s a very similar thing I think for this new technology in terms of AI. It’s going to go through the same kind of trust issues, but it’s a tool that we will eventually adopt just like computers. Now if we look specifically in the aerospace and defense sector, there’s a couple of unique things we need to talk about. As we talked about in the commercial sector, there’s just general intellectual property and companies are concerned. You know, Boeing doesn’t want Airbus to steal their intellectual property to know how they design an aircraft and vice versa.
Todd Tuthill: But if you look at the defense side, there’s even a higher level of scrutiny when it comes into designing things that are a matter of national defense, and the area of classified information. And the way I think we’re going to see this go in aerospace and defense is that, and that’s why machine learning is so critical is that this intellectual property, this machine learning. It’s going to happen in aerospace companies, inside their companies, inside proprietary systems and proprietary data lake. And sometimes we call those air gap systems that aren’t even connected to the Internet. And I think that’s what we’re going to see it start in aerospace. That’s where the companies are going to learn from that.
Todd Tuthill: And Justin talked about user education. We’re going to look at those things and we’re going to be certain that this algorithm is not sharing my intellectual property with somebody else. Now I’m moving it to the Internet. It’s going to start there. That’s how I think these companies will learn to trust. It will have to design systems that do that and as they use them more. As it becomes more mainstream within society, I think aerospace companies will begin to trust it too. Trust is a key issue that I hear when I talk to aerospace customers all the time and it will be a slow gradual process just like it was in Hidden Figures to move from a human being that does calculations to a computer that does.
Patty Russo: Yeah, lots of great analogies and example in this whole conversation. We could talk for quite some time more I’m sure, but we are getting close to our time on this podcast. I’d like to ask one final question and that’s kind of an open-ended question. Is there anything that either of you wanted to comment on that maybe we haven’t covered related to AI/ML and, looking back at the beginning of the podcast, when we talked in terms of digital transformation maturity and those five stages. Is there anything you want to comment on that we haven’t touched on before we wrap up for this episode?
Todd Tuthill: Patty, I think I would say the advice I give to customers because I talk about digital transformation maturity and specifically around AI/ML, and while there’s a lot of great things coming, I don’t want customers to think they have to wait five years to begin investing to, to begin using this technology. There are a lot of things available, even to aerospace customers now that they can apply today in our products. So I say don’t wait. Start investing now. Start using it now. There are plenty of ways that they can benefit from this technology today, and if they begin investing and using it now, they’re going to grow as the tools in the industry grow. Because if they don’t invest, their competitors are going to.
Justin Hodges: Yeah, I think I’ll just piggyback off that. So, I had a slide I showed I think in 2020. So it’s outdated now at this point, but basically it was called the Stanford AI Outlook Survey, and they did the they did this on a yearly basis. It’s a great resource to see kind of the what’s happened this year sort of thing, but the metrics are really are really mind boggling. I mean we talk about you know people are investing and getting on board with it, you know, I totally agree, because even in that year for 2019, the survey, you know, explicitly stated that there was 100,000 publications and some odd change on the topic of machine learning and AI, et cetera, globally for that year in 2019.
Justin Hodges: So that sounds like a lot, but let me put, I mean and it is a lot, but let me just you know give you another figure to show you how much that is. So at that time I thought, “Okay, what’s that scale like?” So I looked up the total number of manuscripts from the American Society of Mechanical Engineers ever since their inception, and, I don’t remember the date 18 something before 1900. Maybe I’ll say 1880, and that number was 250K, so maybe it’s not a one to one comparison, but you can go look at the report from this Stanford AI Outlook and look for yourself.
Justin Hodges: I mean the impact that this is having and the amount of people pushing this, this wheel uphill, you know, to accomplish this, I mean it’s like a revolution for sure. It’s almost like another industrial revolution, and I totally agree that now is the time where yesterday is the time to start doing things. And to just supplement a bit you know don’t be, I’ll say don’t be intimidated. Start with areas you’re interested in. There’s so many applications of AI can be overwhelming, but just, you know, focus on what you’re interested in. There’s a ton of resources to get your hands dirty and start playing with things and doing tutorials without any cost to you on hardware. You don’t have to install with anything resources such as Google Collaboratory or Cagle.
Justin Hodges: There’s so many great courses. My favorite is on Coursera. Just jump in. Don’t feel like you have to systematically provide your full learning path for the next year or that sort of thing. Just, you know, begin and gravitate towards what you’re interested in, and it’ll probably work out fine.
Patty Russo: It’s exciting to think about what’s next and such a great conversation. The application of AI and machine learning is going to happen, I think, faster than we than we might think. Even Justin, you and Todd, who are obviously very passionate and knowledgeable about it, the key messages that I just heard were “don’t wait” and “it’s a revolution.” Just like ChatGPT kind of hit us very quickly and suddenly and everybody’s using it like it’s been around forever. So I would trust that if well one of our listeners, if you’re not using it or looking at opportunities to apply it, chances are somebody in the industry, perhaps your competitor might be. So it’s probably a good time to begin exploring and considering where in your digital transformation journey you can apply some of this technology.
Patty Russo: So Todd and Justin, our time is up. Thank you so much for such great insights in this discussion.
Todd Tuthill: Hey, Patty. Thank you. It’s been a great discussion and I want to say a big thank you to Justin. It’s a lot more interesting, I think, to have three people on the podcast than just the two of us. So you really contributed a lot, Justin. Thank you for coming on today and thank you to our listeners for hanging out to talk about artificial intelligence and machine learning.
Justin Hodges: Thanks a lot for that. The pleasure is all mine. My first professional experience in AI was in 2017 and it was pretty lonely then, so the fact that in the last few years now it’s this tidal wave and everyone’s talking about it and working on it, people like yourself can testify from the industry side how useful it is. It’s just, it’s such a fruitful conversation. It’s such a good time, you know? I enjoyed it. Thanks for inviting me.
Patty Russo: Awesome. Yeah, we hope this conversation helped our audience learn more about AI’s role and digital transformation maturity and how it can potentially be an enabler for our industry as road maps or developed for digital transformation. We’ll definitely have some lively discussions about this and other topics in future episodes. I’m Patty Russo and we look forward to having you join us on our next episode of Talking Aerospace Today.
Siemens Digital Industries Software helps organizations of all sizes digitally transform using software, hardware and services from the Siemens Xcelerator business platform. Siemens’ software and the comprehensive digital twin enable companies to optimize their design, engineering and manufacturing processes to turn today’s ideas into the sustainable products of the future. From chips to entire systems, from product to process, across all industries. Siemens Digital Industries Software – Accelerating transformation.