The value of AI decision support
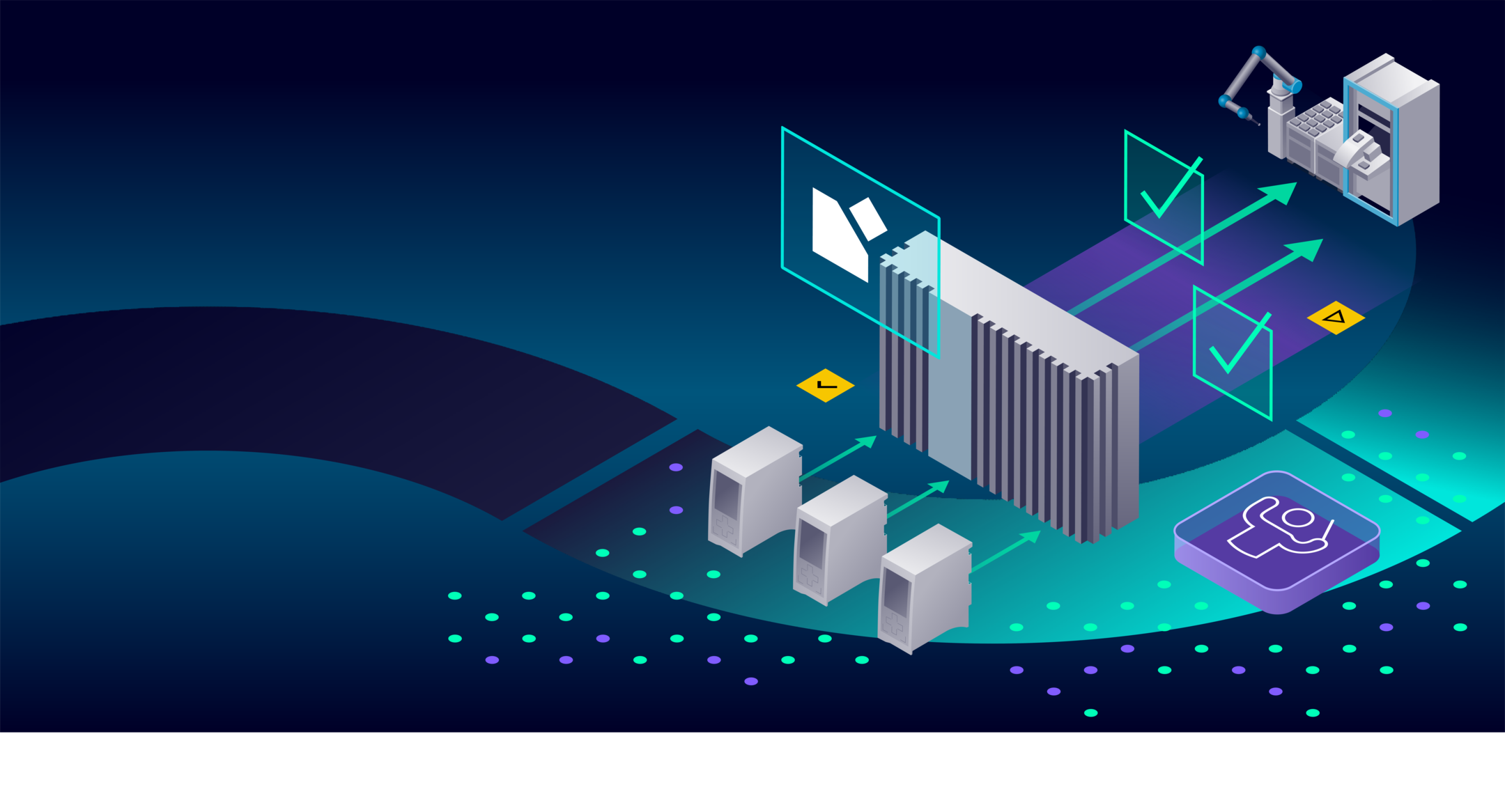
Making decisions is a key part of any business. From deciding top-level strategy to when a machine needs to be taken down for maintenance, interpreting data and reaching a logical conclusion is of critical importance. A big part of making the right decision is sifting through all the available supporting data to look for what’s most important, an often time-consuming process. While AI lacks the critical thinking and reasoning abilities of a human, it far surpasses the human brains ability to collate data and extract useful patterns from it. In a recent podcast with Dr. James Loach of Senseye Predictive Maintenance we how combining AI and human’s abilities together can do a better, smarter and faster job of reaching conclusions and making critical decisions. Keep reading for a summary of the highlights or listen to the full podcast here.
As the manufacturing industry continues its digital transformation journey, an increasing amount of data will be generated by smart machines and systems. While all this data is valuable, allowing for experts to better make informed decisions, managing and understanding it in its raw and unfiltered form would be a nearly impossible task for humans alone. AI, with its fantastic abilities to process data and find patterns is in a prime position to serve as a bridge, filtering the data and highlighting only the most critical information for expert consideration.
James discusses how Senseye takes this approach to predictive maintenance. In the case of maintenance what might traditionally be considered good data, that is to say feedback from a machine that it is working normally with no problems, is actually more akin to noise. Rather, the information of highest value in this scenario is data denoting a problem, which could easily be lost among the terabytes of data constantly streaming off connected machines. Since machines rarely break down, the vast majority of the data they generate will be of limited value when it comes to making decisions. By using AI to filter and present only the most relevant data, not only is it easier for experts to make informed decisions, it also increases the number of machines a single person can monitor without increasing their workload.
In order for an AI decision support system like this to function, trust is critical. James notes that there are many ways to build trust in an AI system but, at its core, Senseye takes the approach of transparency and understandability. Depending on the types of data being analyzed, this can take different forms. In some cases that means making sure algorithms are simple and intuitive while in others it means keeping the complex elements in the places where the user can understand them the easiest. James likens AI recommender systems to a colleague who’s very good at a specific task (in this case, sifting through data) but not very good at many others, such as making decisions. By combining the best of human and AI abilities, it is possible to bridge the gap and attain results superior then either could achieve on their own.
Another way Senseye builds trust is by allowing their system to learn from the user. By accepting expert feedback on whether something that was highlighted as important was or not and, conversely, if something that was missed should have been flagged, users can codify their own expert knowledge into the system, increasing its trustworthiness and making its predictions more accurate. Information on products, production schedules, and system idiosyncrasies can also be manually entered as well, helping teach the AI how to best approach a specific factory or production line.
As the physical and digital worlds become more intertwined, AI will become an even more important tool for connecting people, information and technology. AI decision support systems like those being pioneered by Senseye will be vitally important in the future to help narrow down the vast quantities of available data to only the most critical and impactful for a given application. The melding of human and AI abilities will be one of the driving factors in future innovation with the first steps already being taken toward seamless interaction between the real and digital worlds.
Check out the full podcast here or read along with the transcript here.
Siemens Digital Industries Software helps organizations of all sizes digitally transform using software, hardware and services from the Siemens Xcelerator business platform. Siemens’ software and the comprehensive digital twin enable companies to optimize their design, engineering and manufacturing processes to turn today’s ideas into the sustainable products of the future. From chips to entire systems, from product to process, across all industries. Siemens Digital Industries Software – Accelerating transformation.