AI-driven factories of the future: the transcript
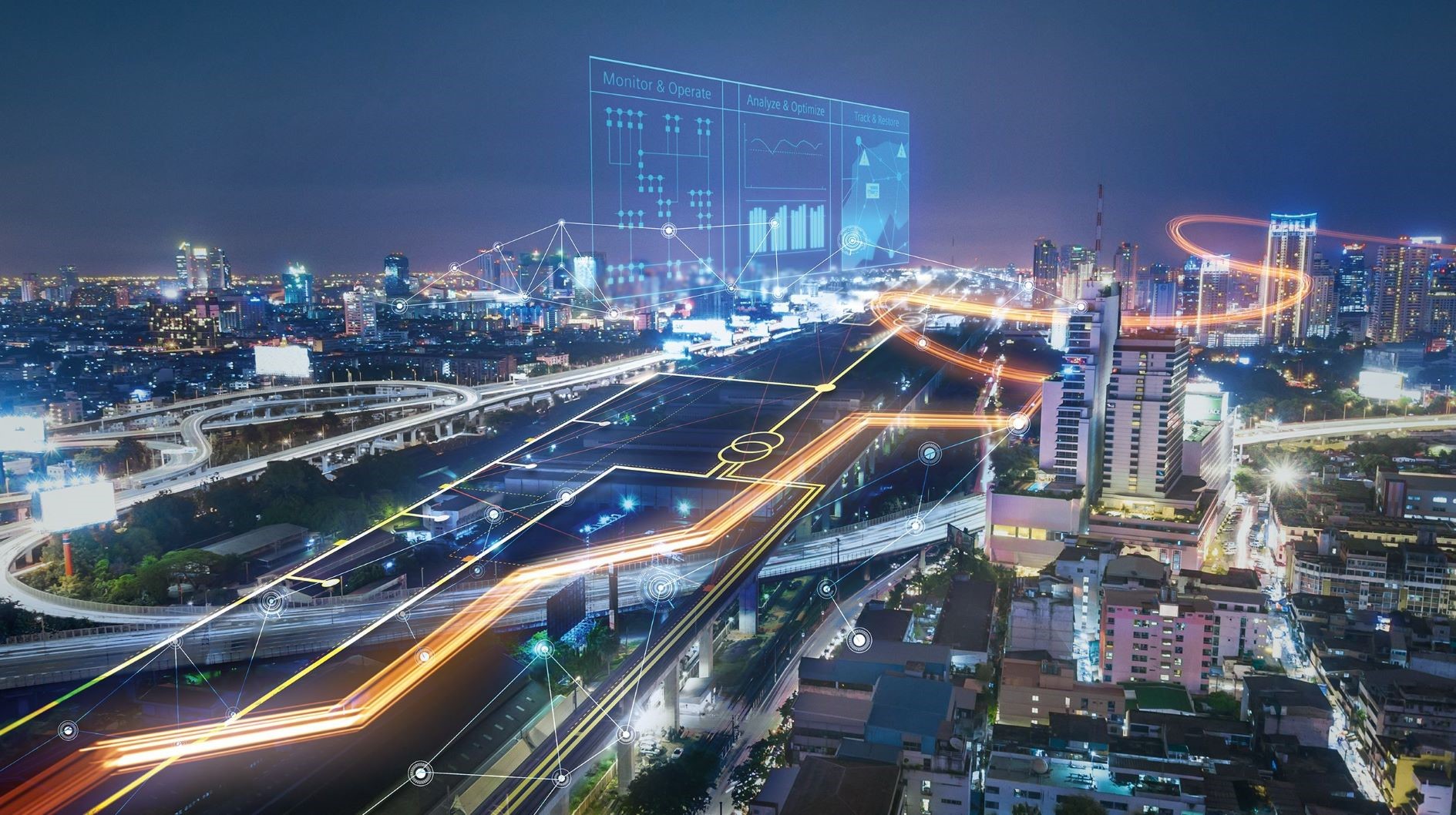
Previously, I summarized a podcast about AI-driven factories of the future, where our expert Dr. Stefan Jockusch discussed his future-forward ideas with the MIT Technology Review. For those that prefer reading instead of listening to the podcast, here is the transcript of that interview:
Laurel Ruma: From MIT Technology Review, I’m Laurel Ruma, and this is Business Lab, the show that helps business leaders make sense of new technologies coming out of the lab and into the marketplace. Our topic today is artificial intelligence and physical applications. AI can run on a chip, on an edge device, in a car, in a factory, and ultimately, AI will run a city with real-time decision-making, thanks to fast processing, small devices, and continuous learning. Two words for you: smart factory.
My guest is Dr. Stefan Jockusch, who is vice president for strategy for Siemens Digital Industries Software. He is responsible for strategic business planning and market intelligence, and Stefan also coordinates projects across business segments and with Siemens Digital Leadership. This episode of Business Lab is produced in association with Siemens Digital Industries. Welcome, Stefan.
Stefan Jockusch: Hi. Thanks for having me.
Laurel: So, if we could start off a bit, could you tell us about Siemens Digital Industries? What exactly do you do?
Stefan: Yeah, in the Siemens Digital Industries, we are the technical software business. So we develop software that supports the whole process from the initial idea of a product like a new cell phone or smartwatch, to the design, and then the manufactured product. So that includes the mechanical design, the software that runs on it, and even the chips that power that device. So with our software, you can put all this into the digital world. And we like to talk about what you get out of that, as the digital twin. So you have a digital twin of everything, the behavior, the physics, the simulation, the software, and the chip. And you can of course use that digital twin to basically do any decision or try out how the product works, how it behaves, before you even have to build it. That’s in a nutshell what we do.
Laurel: So, staying on that idea of the digital twin, how do we explain the idea of chip to city? How can manufacturers actually simulate a chip, its functions, and then the product, say, as a car, as well as the environment surrounding that car?
Stefan: Yeah. Behind that idea is really the thought that in the future, and today already we have to build products, enabling the people who work on that to see the whole, rather than just a little piece. So this is why we make it as big as to say from chip to city, which really means, when you design a chip that runs in a vehicle of today and more so in the future, you have to take a lot of things into account while you are designing that chip. You have to have an idea if the chip, for example, controls the interpretation of things that the cameras see for autonomous driving, you have to have an idea how many images that chip has to process or how many things are moving on those images and obvious pedestrians, what recognition do you have to do? You have to understand a lot about what will happen in the end. So the idea is to enable a designer at the chip level to understand the actual behavior of a product.
And what’s happening today, especially is that we don’t develop cars anymore just with a car in mind, we more and more are connecting vehicles to the environment, to each other. And one of the big purposes, as we all know, that is of course, to improve the contamination in cities and also the traffic in cities, so really to make these metropolitan areas more livable. So that’s also something that we have to take into account in this whole process chain, if we want to see the whole as a designer. So this is the background of this whole idea, chip to city. And again, the way it should look like for a designer, if you think about, I’m designing this vision module in a car, and I want to understand how powerful it has to be. I have a way to immerse myself into a simulation, a very accurate one, and I can see what data my vehicle will see, what’s in them, how many sensor inputs I get from other sources, and what I have to do. I can really play through all of that.
Laurel: I really like that framing of being able to see the whole, not just the piece of this incredibly complex way of thinking, building, delivering. So to get back down to that piece level, how does AI play a role at the chip level?
Stefan: AI is a lot about supporting or even making the right decision in real time. And that’s I think where AI and the chip level become so important together, because we all know that a lot of smart things can be done if you have a big computer sitting somewhere in a data center. But AI and the chip level is really very targeted at these applications that need real-time performance and a performance that doesn’t have time to communicate a lot. And today it’s really evolving to that the chips that do AI applications are now designed already in a very specialized way, whether they have to do a lot of compute power or whether they have to conserve energy as best as they can, so be very low power consumption or whether they need more memory. So yeah, it’s becoming more and more commonplace thing that we see AI embedded in tiny little chips, and then probably in future cars, we will have a dozen or so semiconductor-level AI applications for different things.
Laurel: Well, that brings up a good point because it’s the humans who are needing to make these decisions in real time with these tiny chips on devices. So how does the complexity of something like continuous learning with AI, not just help the AI become smarter but also affect the output of data, which then eventually, even though very quickly, allows the human to make better decisions in real time?
Stefan: I would say most applications of AI today are rather designed to help a human make a good decision rather than making the decision. I don’t think we trust it quite that much yet. So as an example, in our own software, like so many makers of software, we are starting to use AI to make it easier and faster to use. So for example, you have these very complex design applications that can do a lot of things, and of course they have hundreds of menus. So we have one application where the program watches the user and tries to predict the command the user is going to use next. So just to offer it and just say, “Aren’t you about to do this?” And of course, you talked about the continuous improvement, continuous learning—the longer the application can watch the user, the more accurate it will be.
It’s currently already at a level of over 95%, but of course continuous learning improves it. And by the way, this is also a way to use AI not just to help a single user but to start encoding a knowledge, an experience, a varied experience of good users and make it available to other users. If a very experienced engineer does that and uses AI and you basically take those learned lessons from that engineer and give it to someone less experienced who has to do a similar thing, that experience will help the new user as well, the novice user.
Laurel: That’s really compelling because you’re right—you’re building a knowledge database, an actual database of data. And then also this all helps the AI eventually, but then also really does help the human because you are trying to extend this knowledge to as many people as possible. Now, when we think about that and AI at the edge, how does this change opportunities for the business, whether you’re a manufacturer or the person using the device?
Stefan: Yeah. And in general, of course, it’s a way for everyone who makes a smart product to differentiate, to create differentiation because all these, the functions enabled by AI of course are smart, and they give some differentiation. But the example I just mentioned where you can predict what a user will do, that of course is something that many pieces of software don’t have yet. So it’s a way to differentiate. And it certainly opens lots of opportunities to create these very highly differentiated pieces of functionality, whether it’s in software or in vehicles, in any other area.
Laurel: So if we were actually to apply this perhaps to a smart factory and how people think of a manufacturing chain, first this happens, and then that happens and a car door is put on and then an engine is put in or whatever. What can we apply to that kind of traditional way of thinking of a factory and then apply this AI thinking to it?
Stefan: Well, we can start with the oldest problem a factory has had. I mean, factories have always been about producing something very efficiently and continuously and leveraging the resources. So any factory tries to be up and running whenever it’s supposed to be up and running, have no unpredicted or unplanned downtime. So AI is starting to become a great tool to do this. And I can give you a very hands-on example from a Siemens factory that does printed circuit boards. And one of the steps they have to do is milling of these circuit boards. They have a milling machine and any milling machine, especially one like that that’s highly automated and robotic, it has a tendency to goop up over time, to get dirty. And so one challenge is to have the right maintenance because you don’t want the machine to fail right in the middle of a shift and create this unplanned downtime.
So one big challenge is to figure out when this machine has to be maintained, without of course, maintaining it every day, which would be very expensive. So we are using actually an AI application on an edge device that’s sitting right in the factory, to monitor that machine and make a fairly accurate prediction when it’s time to do the maintenance and clean the machine so it doesn’t fail in the next shift. So this is just one example, and I believe there is hundreds of potential applications that may not be totally worked out yet in this area of really making sure that factories produce consistent high quality, that there’s no unplanned downtime of the machines. There’s of course, a lot of use already of AI in visual quality inspections. So there’s tons and tons of applications on the factory floor.
Laurel: And this has massive implications for manufacturers, because as you mentioned, it saves money, right? So is this a tough shift, do you think, for executives to think about investing in technology in a bit of a different way to then get all of those benefits?
Stefan: Yeah. It’s like with every technology, I wouldn’t think it’s a big block, there’s a lot of interest at this point and there’s many manufacturers with initiatives in that space. So I would say it’s probably going to create a significant progress in productivity, but of course, it also means investment. And I can say since it’s fairly predictable to see what the payback of this investment will be. As far as we can see, there’s a lot of positive energy there, to make this investment and to modernize factories.
Laurel: What kind of modernizations you need for the workforce in the factories when you are installing and applying, kind of retooling to have AI applications in mind?
Stefan: That’s a great question because sometimes I would say many users of artificial intelligence applications probably don’t even know they’re using one. So you basically get a box and it will tell you, is recommended to maintain this machine now. The operator probably will know what to do, but not necessarily know what technology they’re working with. But that said of course there will probably will be some, I would say, almost emerging specialties or emerging skills for engineers to really, how to use and how to optimize these AI applications that they use on the factory floor. Because as I said, we have these applications that are up and running and working today, but to get to those applications to be really useful, to be accurate enough, that of course, to this point needs a lot of expertise, at least some iteration as well. And there’s probably not too many people today who really are experienced enough with the technologies and also understand the factory environment well enough to do this.
I think this is a fairly, pretty rare skill these days and to make this a more commonplace application of course we will have to create more of these experts who are really good at making AI factory-floor-ready and getting it to the right maturity.
Laurel: That seems to be an excellent opportunity, right? For people to learn new skills. This is not an example of AI taking away jobs and that more negative connotations that you get when you talk about AI and business. In practice, if we combine all of this and talk about VinFast, the Vietnamese car manufacturer that wanted to do things quite a bit differently than traditional car manufacturing. First, they built a factory, but then they applied that kind of overarching thinking of chip to factory and then eventually to city. So coming back full circle, why is this thinking unique, especially for a car manufacturer and what kind of opportunities and challenges do they have?
Stefan: Yeah. VinFast is an interesting example because when they got into making vehicles, they basically started on a green field. And that is probably the biggest difference between VinFast and the vast majority of the major automakers. That all of them are a hundred or more years old and have of course a lot of history, which then translates into having existing factories or having a lot of things that were really built before the age of digitalization. So VinFast started from a Greenfield, and that of course is a big challenge, it makes it very difficult. But the advantage was that they really have the opportunity to start off with a full digitalized approach, that they were able to use software. Because they were basically constructing everything, and they could really start off with this fairly complete digital twin of not only their product but also they designed the whole factory on a computer before even starting to build it. And then they build it in record time.
So that’s probably the big, unique aspect that they have this opportunity to be completely digital. And once you are at that state, once you can already say my whole design, of course, my software running on the vehicle, but also my whole factory, my whole factory automation. I already have this in a fully digital way and I can run through simulations and scenarios. That also means you have a great starting point to use these AI technologies to optimize your factory or to help the workers with the additional optimizations and so on.
Laurel: Do you think it’s impossible to be one of those hundred-year-old manufacturers and slowly adopt these kinds of technologies? You probably don’t have to have a Greenfield environment, it just makes everything easy or I should say easier, right?
Stefan: Yeah. All of them, I mean the auto industry has traditionally been one of the one that invested most in productivity and in digitalization. So all of them are on that path. Again, they don’t have this very unique situation that you, or rarely have this unique situation that you can really start from a blank slate. But a lot of the software technology of course, also is adapted to that scenario. Where for example, you have an existing factory, so it doesn’t help you a lot to design a factory on the computer if you already have one. So you use these technologies that allow you to go through the factory and do a 3D scan. So you know exactly how the factory looks like from the inside without having it designed in a computer, because you essentially produce that information after the fact. So that’s definitely what the established or the traditional automakers do a lot and where they’re also basically bringing the digitalization even into the existing environment.
Laurel: We’re really discussing the implications when companies can use simulations and scenarios to apply AI. So when you can, whether or not it’s Greenfield or you’re adopting it for your own factory, what happens to the business? What are the outcomes? Where are some of the opportunities that are possible when AI can be applied to the actual chip, to the car, and then eventually to the city, to a larger ecosystem?
Stefan: Yeah. When we really think about the impact to the business, I frankly think we are at the beginning of understanding and calculating what the value of faster and more accurate decisions really is, which are enabled by AI. I don’t think we have a very complete understanding at this point, and it’s fairly obvious to everybody that digitalizing like the design process and the manufacturing process. It not only saves R&D effort and R&D money, but it also helps optimize the supply chain inventories, the manufacturing costs, and the total cost of the new product. And that is really where different aspects of the business come together. And I would frankly say, we start to understand the immediate effects, we start to understand if I have an AI-driven quality check that will reduce my waste, so I can understand that kind of business value.
But there is a whole dimension of business value of using this optimization that really translates to the whole enterprise. And I would say there’s a lot of work happening to understand these implications better. But I would say at this point, we are just at the starting point of doing this, of really understanding what can optimization of a process do for the enterprise as a whole.
Laurel: So optimization, continuous learning, continuous improvement, this makes me think of, and cars, of course, The Toyota Way, which is that seminal book that was written in 2003, which is amazing, because it’s still current today. But with lean manufacturing, is it possible for AI to continuously improve that at the chip level, at the factory level, at the city to help these businesses make better decisions?
Stefan: Yeah. In my view, The Toyota Way, again, the book published in the early 2000s, with continuous improvement, in my view, continuous improvement of course always can do a lot, but there’s a little bit of recognition in the last, I would say five to 10 years, somewhere like that, that continuous improvement might have hit the wall of what’s possible. So there is a lot of thought since then of what is really the next paradigm for manufacturing. When you stop thinking about evolution and optimization and you think about more revolution. And one of the concepts that have been developed here is called industry 4.0, which is really the thought about turning upside down the idea of how manufacturing or how the value chain can work. And really think about what if I get two factories that are completely self-organizing, which is kind of a revolutionary step. Because today, mostly a factory is set up around a certain idea of what products it makes and when you have lines and conveyors and stuff like that, and they’re all bolted to the floor. So it’s fairly static, the original idea of a factory. And you can optimize it in an evolutionary way for a long time, but you’d never break through that threshold.
So the newest thought or the other concepts that are being thought about are, what if my factory consists of independent, moving robots, and the robots can do different tasks. They can transport material, or they can then switch over to holding a robot arm or a gripper. And depending on what product I throw at this factory, it will completely reshuffle itself and work differently when I come in with a very different product and it will self-organize itself to do something different. So those are some of the paradigms that are being thought of today, which of course, can only become a reality with heavy use of AI technologies in them. And we think they are really going to revolutionize at least what some kinds of manufacturing will do. Today we talk a lot about lot size one, and that customers want more options and variations in a product. So the factories that are able to do this, to really produce very customized products, very efficiently, they have to look much different.
So in many ways, I think there’s a lot of validity to the approach of continuous improvement. But I think we right now live in a time where we think more about a revolution of the manufacturing paradigm.
Laurel: That’s amazing. The next paradigm is revolution. Stefan, thank you so much for joining us today in what has been an absolutely fantastic conversation on the Business Lab.
Stefan: Absolutely. My pleasure. Thank you.
Laurel: That was Stefan Jockusch, vice president of strategy for Siemens Digital Industry Software, who I spoke with from Cambridge, Massachusetts, the home of MIT and MIT Technology Review, overlooking the Charles River. That’s it for this episode of Business Lab. I’m your host, Laurel Ruma. I’m the director of Insights, the custom publishing division of MIT Technology Review. We were founded in 1899 at the Massachusetts Institute of Technology. And you can find us in prints, on the web, and at events online and around the world. For more information about us and the show, please check out our website at technologyreview.com. The show is available wherever you get your podcasts. If you enjoyed this episode, we hope you’ll take a moment to rate and review us. Business Lab is a production of MIT Technology Review. This episode was produced by Collective Next. Thanks for listening.
This transcript was provided courtesy of the MIT Technology Review.