Enhancing supply chain prediction with AI
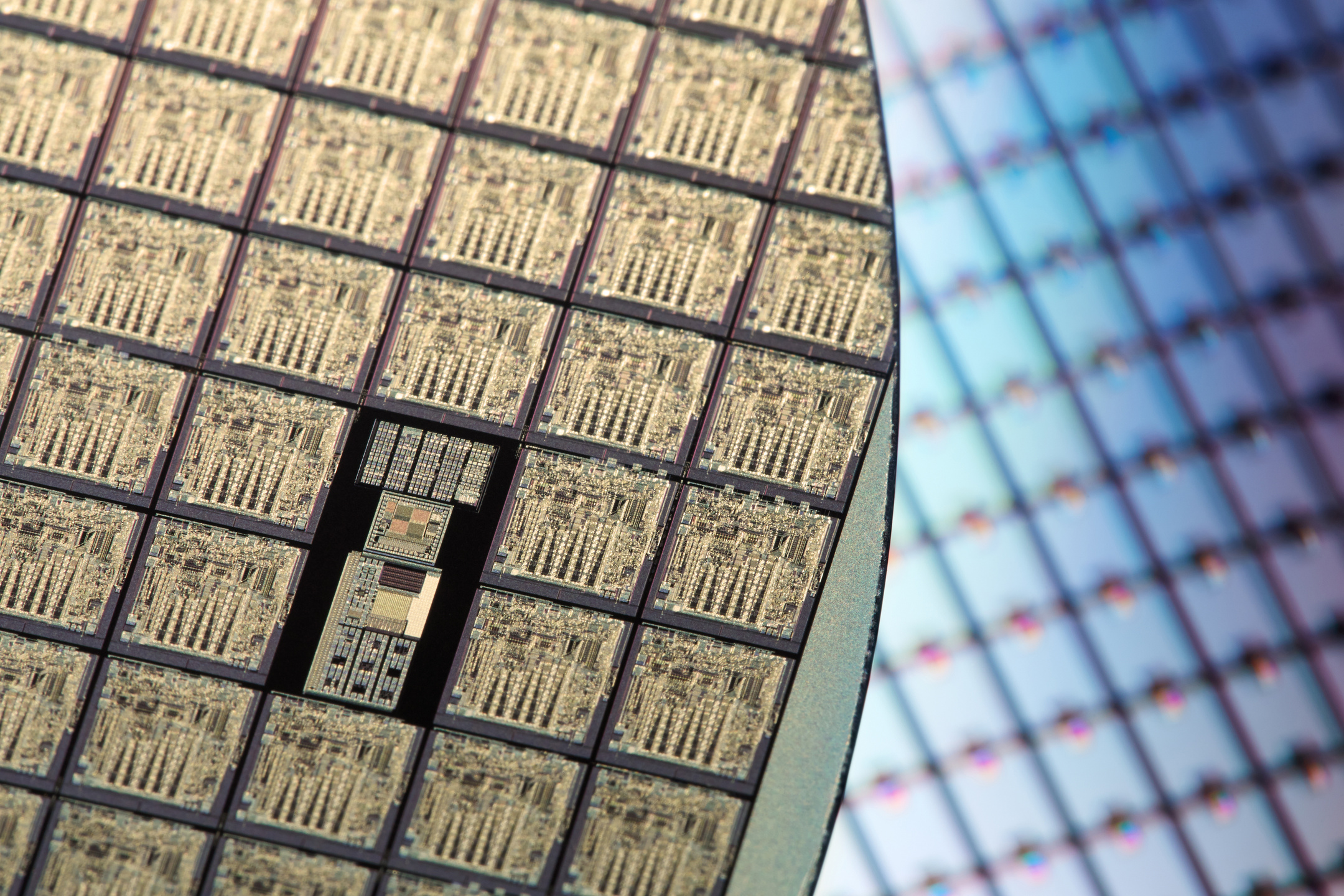
In a recent podcast, I had the opportunity to continue my conversation with Richard Barnett, Chief Marketing Officer for Supplyframe. I wanted to understand how AI plays a vital role in understanding the challenges, risks, and patterns of the global electronics value chain. In this episode, Richard discusses why, compared to traditional models and methods, AI is better suited to understanding the full breadth of modern, globally connected, supply chains. The greatest advantage is that traditional methods can only look at thin slices of the data, rather than a more comprehensive whole which AI is capable of. For the unabridged discussion you can listen to the full podcast here, but keep reading for the highlights.
Supplyframe offers a unique industrial ecosystem and pioneering Design-to-Source Intelligence (DSI) solutions which transform how people and businesses design, source, market, and sell products across the global electronics value chain. Leveraging billions of continuous signals of design intent, demand, supply, and risk factors, Supplyframe’s DSI Platform is the world’s richest intelligence resource for the electronics industry with over 10 million engineers engaging with the platform worldwide.
Richard notes that at Supplyframe, they’ve found is that the electronics value chain often only looks at thin slices of data such as past sales or financial measure for insight into trends which isn’t enough to get a complete picture of where the market is going. By limiting forecasting metrics to simple historical sales data or demand projections based on current order volume with an eye to only understand short-term profitability – what will happen next week or next quarter – companies can easily be caught flat-footed when external factors strongly impact the market.
To highlight this point, Richard uses the case of the automotive industry during the early days of the COVID pandemic. During this time, anticipating lockdowns and lowering car sales, major automotive OEMs slashed their projections and, in turn, the number of ICs and other components they had on order near the beginning of 2020. However, by the end of that year, in December, many were forced to completely shutdown factories as a combination of suppliers diverting components to different buyers and lead times increasing from a few weeks up to 30, 40, or 50 weeks and beyond meant that components which had been available just-in-time were now virtually nonexistent. Using traditional forecasting methods, these OEMs only became aware of the problem a few weeks before its effects were felt, far too late to do anything about it.
Taking the above case as an opportunity to test their AI algorithms, Supplyframe combed through their historical data and found that their model was showing early warning signs as far back as April – a full 8 months before the problem arose. Strong indicators in May and June could have helped alleviate the challenges faced by automotive OEMs later in the year by showing reliable predictions much further into the future, even as much as 18 months ahead Richard noted in our discussion.
While this is an extreme example, it illustrates the benefits of using AI to examine data networks holistically. Because their model was able to more accurately predict the impact of the pandemic across all levels of the supply chain, from raw material all the way to finished assembly, Supplyframe could see how changes would propagate through the complex network of supply and logistics in a way that simply isn’t possible without AI-enabled methods.
When it comes to something as complex a global supply chain, looking for complex patterns and extracting insights from it is simply impossible using traditional methods, the volume of data is too high and the interplay between different elements too complex for humans alone to manage. Artificial intelligence disrupts that notion and even thrives in complex data sets, finding patterns and drawing conclusions impossible with traditional methods and models. AI is leading the way for companies to capitalize on the vast quantities of existing data in new and novel ways while at the same time granting increased flexibility to handle even the most unexpected situations.
Siemens Digital Industries Software helps organizations of all sizes digitally transform using software, hardware and services from the Siemens Xcelerator business platform. Siemens’ software and the comprehensive digital twin enable companies to optimize their design, engineering and manufacturing processes to turn today’s ideas into the sustainable products of the future. From chips to entire systems, from product to process, across all industries. Siemens Digital Industries Software – Accelerating transformation.