What is the difference between data and analytics?
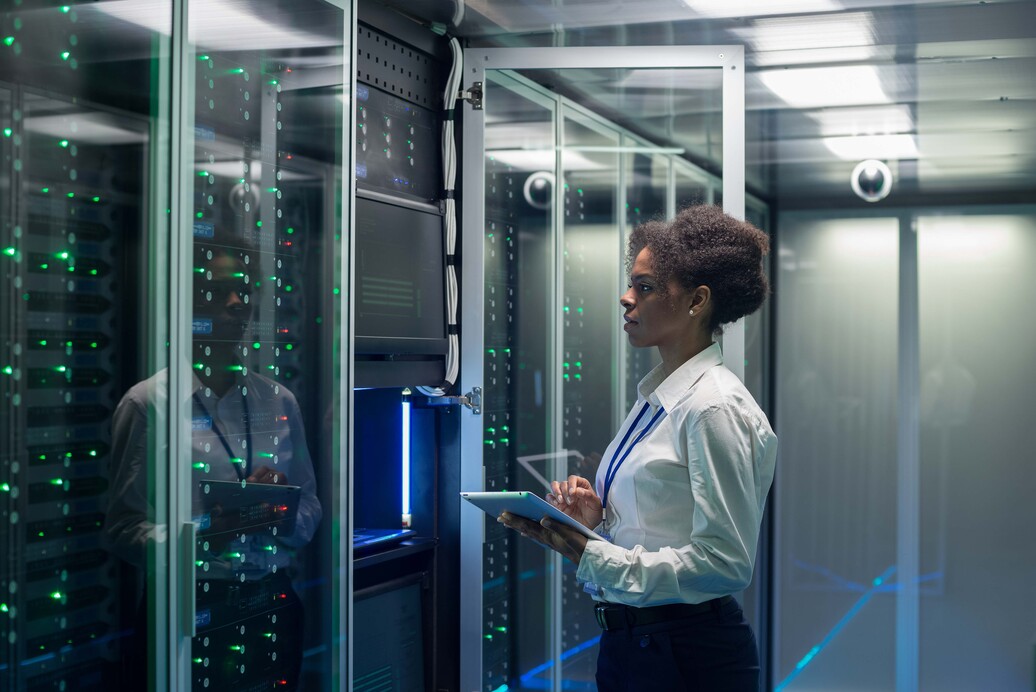
Manufacturing processes today generate enormous amounts of raw data. Collecting and storing big data is costly and doesn’t automatically deliver a return on investment. To deliver real value, raw data must be converted into smart data—validated, standardized and prioritized. Only then can the data be utilized to create analytics—insights and predictions that can be applied back to the manufacturing processes in order to improve processes and overall efficiency.
Not all analytics are the same. There are several types of analytics that can be used in manufacturing, each delivering unique value:
1. Descriptive analytics
At the most basic level, analytics need to be able to describe what is happening in the manufacturing process. This can be accomplished by identifying indicators for key performance and operational efficiency and aggregating process and material data to provide detailed traceability reports that can also assist with proof of compliance and recall management.
2. Diagnostic analytics
After describing what is happening in the manufacturing process, the next step is to determine why it’s happening. Diagnostic analytics connect multiple data sources, preferably at the corporate and site levels, to gain insight into why KPIs behave in a certain way. They also help to identify root causes, as well as yield, utilization, quality, and performance issues.
3. Predictive analytics
The next step is to try to predict what will happen in the future. Using insights created through descriptive and diagnostic analytics, predictive analytics attempt to identify trends and predict future outcomes.
4. Prescriptive analytics
Prescriptive analytics utilize the accumulated data and the behavior patterns to drive effective and efficient executive decision-making.
Analytics in the field – example
Without analytics driven by smart data, electronics manufacturers can only assess overall equipment or line efficiency from a limited perspective. For example, they may focus solely on component placement, even though there are other issues, such as other bottlenecks or changeovers, that may have a greater impact on efficiency. Turning data into smart data and utilizing analytics can provide insights that address the entire design-to-manufacturing flow.
For instance, in a given week there are often multiple planned stoppages for maintenance, changeovers, inventory count, shift changes, and planning meetings as well as weekends and holidays that reduce the available production time on a production line. Smart data-driven analytics incorporating all these factors would enable managers to understand that If there is no third shift, it is impossible to reach 100% overall equipment efficiency (OEE), no matter what is happening in the placement process.
Availability breakdown also has a significant impact on OEE. If there is a line waiting upstream, the printer may have to wait for the pick-and-place machines, and the oven might have to wait for a line waiting downstream. If the printer is really slowing down upstream, it could be prudent to take the solder paste and solder paste inspection (SPand SPI) offline—it’s a simple step that can increase the line performance and eliminate soldering issues on the line. Analytics that factor real numbers into OEE and the current utilization rate (CUR) provide the overall picture and a baseline for comparison to enable better decisions and solutions.
In a nutshell
In general, analytics can be used to better manage assets and improve real-time utilization and OEE. They are critical for capturing and investigating complete material and process traceability data for individual PCBs. Analytics make it possible to assess the entire design-to-manufacturing flow to identify and analyze process and material failures and drive continuous improvement.
Our ebook, The Electronic Manufacturer’s Guide to Practical Data Analytics, explores this topic in more depth: